用Python进行逐步回归的方法有多种,其中常见的包括使用statsmodels库、scikit-learn库以及手动实现逐步回归。逐步回归的基本步骤包括:特征选择、模型拟合、模型评估。本文将详细介绍如何使用Python进行逐步回归,并结合代码示例进行说明。
逐步回归是一种特征选择方法,用于根据特征的重要性逐步添加或删除特征,从而构建最优回归模型。主要分为前向选择(Forward Selection)、后向消除(Backward Elimination)和双向逐步回归(Stepwise Regression)三种方法。
一、逐步回归的基本概念
逐步回归是一种特征选择方法,主要用于线性回归模型中。其基本思想是通过添加或删除特征,来找到最优的特征子集,从而构建出性能最好的模型。根据特征添加或删除的顺序,逐步回归分为三种:
- 前向选择(Forward Selection):从空模型开始,逐步添加特征,每次添加一个特征,使得模型的表现(如AIC、BIC、R²等)得到最大提升。
- 后向消除(Backward Elimination):从包含所有特征的模型开始,逐步删除特征,每次删除一个不显著的特征,使得模型的表现得到最大提升。
- 双向逐步回归(Stepwise Regression):结合前向选择和后向消除,每次添加或删除特征,使得模型的表现得到最大提升。
二、数据准备
在进行逐步回归之前,我们需要准备数据。这里我们使用波士顿房价数据集作为示例,该数据集包含506个样本,每个样本有13个特征。
import pandas as pd
from sklearn.datasets import load_boston
import statsmodels.api as sm
加载波士顿房价数据集
boston = load_boston()
X = pd.DataFrame(boston.data, columns=boston.feature_names)
y = pd.Series(boston.target)
查看数据集信息
print(X.head())
print(y.head())
三、前向选择(Forward Selection)
前向选择是一种逐步回归方法,从空模型开始,每次添加一个特征,使得模型的表现(如AIC、BIC、R²等)得到最大提升。下面是前向选择的实现代码:
def forward_selection(X, y, significance_level=0.05):
initial_features = X.columns.tolist()
best_features = []
while len(initial_features) > 0:
remaining_features = list(set(initial_features) - set(best_features))
new_pval = pd.Series(index=remaining_features)
for new_column in remaining_features:
model = sm.OLS(y, sm.add_constant(pd.DataFrame(X[best_features + [new_column]]))).fit()
new_pval[new_column] = model.pvalues[new_column]
min_p_value = new_pval.min()
if min_p_value < significance_level:
best_features.append(new_pval.idxmin())
else:
break
return best_features
进行前向选择
best_features = forward_selection(X, y)
print("Selected features:", best_features)
四、后向消除(Backward Elimination)
后向消除是一种逐步回归方法,从包含所有特征的模型开始,每次删除一个不显著的特征,使得模型的表现得到最大提升。下面是后向消除的实现代码:
def backward_elimination(X, y, significance_level=0.05):
features = X.columns.tolist()
while len(features) > 0:
model = sm.OLS(y, sm.add_constant(pd.DataFrame(X[features]))).fit()
max_p_value = model.pvalues[1:].max()
if max_p_value >= significance_level:
excluded_feature = model.pvalues[1:].idxmax()
features.remove(excluded_feature)
else:
break
return features
进行后向消除
best_features = backward_elimination(X, y)
print("Selected features:", best_features)
五、双向逐步回归(Stepwise Regression)
双向逐步回归结合了前向选择和后向消除,每次添加或删除特征,使得模型的表现得到最大提升。下面是双向逐步回归的实现代码:
def stepwise_selection(X, y, significance_level=0.05):
initial_features = X.columns.tolist()
best_features = []
while len(initial_features) > 0:
remaining_features = list(set(initial_features) - set(best_features))
new_pval = pd.Series(index=remaining_features)
for new_column in remaining_features:
model = sm.OLS(y, sm.add_constant(pd.DataFrame(X[best_features + [new_column]]))).fit()
new_pval[new_column] = model.pvalues[new_column]
min_p_value = new_pval.min()
if min_p_value < significance_level:
best_features.append(new_pval.idxmin())
while len(best_features) > 0:
model = sm.OLS(y, sm.add_constant(pd.DataFrame(X[best_features]))).fit()
max_p_value = model.pvalues[1:].max()
if max_p_value >= significance_level:
excluded_feature = model.pvalues[1:].idxmax()
best_features.remove(excluded_feature)
else:
break
else:
break
return best_features
进行双向逐步回归
best_features = stepwise_selection(X, y)
print("Selected features:", best_features)
六、模型评估
完成特征选择后,我们可以使用选择的特征来构建最终的回归模型,并对模型进行评估。下面是模型评估的代码:
from sklearn.model_selection import train_test_split
from sklearn.linear_model import LinearRegression
from sklearn.metrics import mean_squared_error, r2_score
分割数据集
X_train, X_test, y_train, y_test = train_test_split(X[best_features], y, test_size=0.2, random_state=42)
构建回归模型
model = LinearRegression()
model.fit(X_train, y_train)
预测结果
y_pred = model.predict(X_test)
评估模型
mse = mean_squared_error(y_test, y_pred)
r2 = r2_score(y_test, y_pred)
print(f"Mean Squared Error: {mse}")
print(f"R² Score: {r2}")
七、总结
本文详细介绍了逐步回归的基本概念和实现方法,包括前向选择、后向消除和双向逐步回归。通过对波士顿房价数据集的实例分析,展示了逐步回归的实际应用过程。逐步回归是一种有效的特征选择方法,能够帮助我们构建性能优良的回归模型。在实际应用中,我们可以根据具体问题选择合适的逐步回归方法,来完成特征选择和模型构建。
相关问答FAQs:
逐步回归是什么,它的应用场景有哪些?
逐步回归是一种用于选择回归模型中自变量的统计方法。它通过逐步添加或删除变量来寻找最佳的回归模型。逐步回归常用于多重线性回归分析,特别是在自变量较多的情况下。它能够帮助研究人员和数据分析师识别出对因变量影响最大的因素,从而提高模型的预测能力。在经济学、医学、社会科学等领域,逐步回归广泛应用于变量选择和模型优化。
在Python中如何实现逐步回归?
在Python中,逐步回归通常通过使用statsmodels库来实现。首先,您需要导入所需的库并准备数据。可以使用OLS方法来拟合模型,并结合AIC(赤池信息量准则)或BIC(贝叶斯信息量准则)来评估模型的优劣。通过编写一个函数循环添加或删除变量,逐步寻找最佳的自变量组合。此外,使用sklearn库中的特征选择模块也可以实现类似的功能。
逐步回归的优缺点是什么?
逐步回归的优点在于其简便性和直观性,能够快速识别出重要的自变量,适合初步的数据分析。然而,其缺点也不可忽视,包括可能导致模型过拟合以及在变量间存在多重共线性时表现不佳。此外,逐步回归依赖于特定的准则(如AIC或BIC),可能会受到样本大小和变量选择顺序的影响,因此在使用时需谨慎评估模型的可靠性。
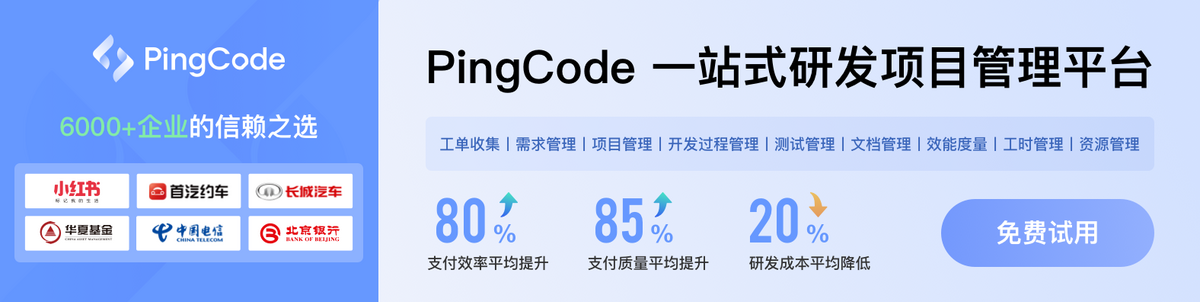