Python做内容查重率的方法有以下几种:使用哈希算法、使用n-gram模型、利用余弦相似度计算。其中,余弦相似度计算是一种常见且有效的方法。它通过将文本转换为向量,然后计算向量之间的夹角余弦值来判断相似度。具体实现时,可以利用Python的相关库,如sklearn
、nltk
等。接下来,我们将详细介绍如何使用这些方法来实现内容查重。
一、哈希算法
哈希算法是一种将文本数据转换为固定长度的字符串的方法。常见的哈希算法有MD5和SHA-1。通过将文本转换为哈希值,然后比较哈希值,可以判断两个文本是否相同。这种方法简单高效,但无法处理轻微修改后的文本。
1.1 使用MD5哈希算法
MD5(Message-Digest Algorithm 5)是计算机安全领域广泛使用的哈希函数,可以生成一个128位的哈希值。以下是使用Python实现MD5哈希算法的例子:
import hashlib
def calculate_md5(text):
md5 = hashlib.md5()
md5.update(text.encode('utf-8'))
return md5.hexdigest()
text1 = "This is a sample text."
text2 = "This is a sample text."
hash1 = calculate_md5(text1)
hash2 = calculate_md5(text2)
if hash1 == hash2:
print("The texts are identical.")
else:
print("The texts are different.")
1.2 使用SHA-1哈希算法
SHA-1(Secure Hash Algorithm 1)是一种广泛使用的哈希函数,可以生成一个160位的哈希值。以下是使用Python实现SHA-1哈希算法的例子:
import hashlib
def calculate_sha1(text):
sha1 = hashlib.sha1()
sha1.update(text.encode('utf-8'))
return sha1.hexdigest()
text1 = "This is a sample text."
text2 = "This is a sample text."
hash1 = calculate_sha1(text1)
hash2 = calculate_sha1(text2)
if hash1 == hash2:
print("The texts are identical.")
else:
print("The texts are different.")
二、n-gram模型
n-gram模型是一种基于统计的文本相似度计算方法。它通过将文本分割成n个连续的单词或字符的序列,然后比较这些序列之间的相似度来判断文本的相似度。
2.1 n-gram模型介绍
n-gram模型的基本思想是将文本分割成n个连续的单词或字符的序列。例如,对于文本 "This is a sample text.",当n=2时,可以得到以下2-gram序列:
['This is', 'is a', 'a sample', 'sample text']
通过比较这些序列之间的相似度,可以判断文本的相似度。
2.2 使用Python实现n-gram模型
以下是使用Python实现n-gram模型的例子:
from collections import Counter
def ngram(text, n):
words = text.split()
return [tuple(words[i:i+n]) for i in range(len(words)-n+1)]
def calculate_similarity(text1, text2, n):
ngrams1 = ngram(text1, n)
ngrams2 = ngram(text2, n)
counter1 = Counter(ngrams1)
counter2 = Counter(ngrams2)
intersection = sum((counter1 & counter2).values())
union = sum((counter1 | counter2).values())
return intersection / union
text1 = "This is a sample text."
text2 = "This is a sample text."
similarity = calculate_similarity(text1, text2, 2)
print(f"Similarity: {similarity:.2f}")
三、余弦相似度计算
余弦相似度(Cosine Similarity)是一种常用的文本相似度计算方法。它通过将文本转换为向量,然后计算向量之间的夹角余弦值来判断相似度。余弦相似度的取值范围在0到1之间,值越接近1,表示文本越相似。
3.1 余弦相似度介绍
余弦相似度的公式如下:
cosine_similarity = (A · B) / (||A|| ||B||)
其中,A和B是两个文本的向量,A · B表示向量A和向量B的点积,||A||和||B||分别表示向量A和向量B的模。
3.2 使用Python实现余弦相似度计算
以下是使用Python实现余弦相似度计算的例子:
from sklearn.feature_extraction.text import CountVectorizer
from sklearn.metrics.pairwise import cosine_similarity
def calculate_cosine_similarity(text1, text2):
vectorizer = CountVectorizer().fit_transform([text1, text2])
vectors = vectorizer.toarray()
cosine_sim = cosine_similarity(vectors)
return cosine_sim[0][1]
text1 = "This is a sample text."
text2 = "This is a sample text."
similarity = calculate_cosine_similarity(text1, text2)
print(f"Cosine Similarity: {similarity:.2f}")
3.3 使用TF-IDF改进余弦相似度计算
TF-IDF(Term Frequency-Inverse Document Frequency)是一种常用的文本特征提取方法,可以提高余弦相似度计算的准确性。以下是使用Python实现TF-IDF改进余弦相似度计算的例子:
from sklearn.feature_extraction.text import TfidfVectorizer
from sklearn.metrics.pairwise import cosine_similarity
def calculate_tfidf_cosine_similarity(text1, text2):
vectorizer = TfidfVectorizer().fit_transform([text1, text2])
vectors = vectorizer.toarray()
cosine_sim = cosine_similarity(vectors)
return cosine_sim[0][1]
text1 = "This is a sample text."
text2 = "This is a sample text."
similarity = calculate_tfidf_cosine_similarity(text1, text2)
print(f"TF-IDF Cosine Similarity: {similarity:.2f}")
四、综合应用
在实际应用中,可以将上述几种方法结合使用,以提高查重的准确性。例如,可以先使用哈希算法进行快速筛选,然后使用n-gram模型和余弦相似度计算进行进一步比较。
4.1 综合应用示例
以下是一个综合应用示例:
import hashlib
from collections import Counter
from sklearn.feature_extraction.text import TfidfVectorizer
from sklearn.metrics.pairwise import cosine_similarity
def calculate_md5(text):
md5 = hashlib.md5()
md5.update(text.encode('utf-8'))
return md5.hexdigest()
def ngram(text, n):
words = text.split()
return [tuple(words[i:i+n]) for i in range(len(words)-n+1)]
def calculate_ngram_similarity(text1, text2, n):
ngrams1 = ngram(text1, n)
ngrams2 = ngram(text2, n)
counter1 = Counter(ngrams1)
counter2 = Counter(ngrams2)
intersection = sum((counter1 & counter2).values())
union = sum((counter1 | counter2).values())
return intersection / union
def calculate_tfidf_cosine_similarity(text1, text2):
vectorizer = TfidfVectorizer().fit_transform([text1, text2])
vectors = vectorizer.toarray()
cosine_sim = cosine_similarity(vectors)
return cosine_sim[0][1]
def check_similarity(text1, text2):
hash1 = calculate_md5(text1)
hash2 = calculate_md5(text2)
if hash1 == hash2:
print("The texts are identical.")
return
ngram_similarity = calculate_ngram_similarity(text1, text2, 2)
tfidf_cosine_similarity = calculate_tfidf_cosine_similarity(text1, text2)
print(f"n-gram Similarity: {ngram_similarity:.2f}")
print(f"TF-IDF Cosine Similarity: {tfidf_cosine_similarity:.2f}")
text1 = "This is a sample text."
text2 = "This is a sample text."
check_similarity(text1, text2)
通过综合使用哈希算法、n-gram模型和余弦相似度计算,可以更准确地判断文本的相似度,从而实现高效的内容查重。
相关问答FAQs:
如何使用Python检测文本的相似性?
使用Python进行文本相似性检测,通常可以利用多种方法,如计算余弦相似度、Jaccard相似性或使用自然语言处理库,如spaCy或NLTK。通过分词、去除停用词后,可以对文本进行向量化处理,再通过相应的算法计算相似度分数,判断文本是否存在重复内容。
有哪些Python库可以帮助我进行内容查重?
有几个常用的Python库可以帮助进行内容查重,包括difflib
、fuzzywuzzy
和sklearn
。difflib
提供了简单的文本比较功能,fuzzywuzzy
能够处理模糊匹配,而sklearn
则可用于更复杂的文本分析和机器学习模型构建。
如何评估查重结果的准确性?
评估查重结果的准确性可以通过人工抽样检查、计算查重算法的精确度和召回率等方式进行。如果使用机器学习模型,可以通过交叉验证方法评估模型的性能,以确保查重结果的可靠性和有效性。此外,结合多种查重算法的结果也有助于提升准确性。
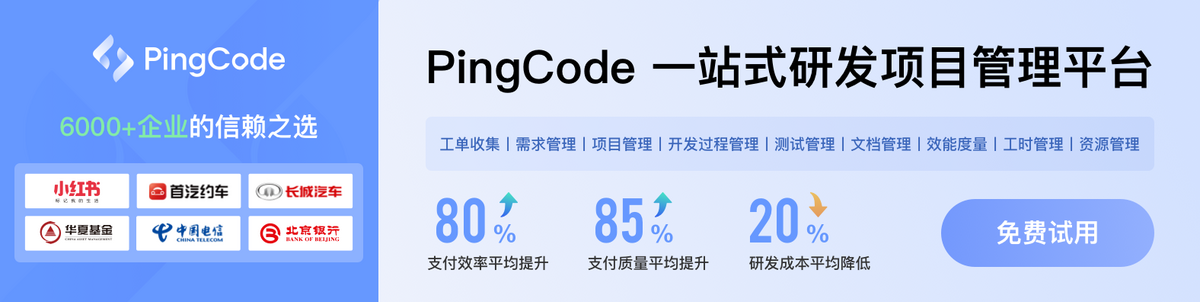