在Python中,可以通过多种方式来输出较大数据,常见的方法包括使用文件操作、使用数据持久化工具如数据库和序列化工具、优化内存管理等。 其中,使用文件操作是最常用且简单的一种方式。通过将数据写入文件,可以避免一次性在内存中存储大量数据,减轻内存压力。下面将详细介绍这些方法。
一、文件操作
1.1 使用 open()
函数写入文件
Python 提供了内置的 open()
函数来进行文件操作。通过 open()
函数可以将数据写入文件,从而实现较大数据的输出。
data = "这里是一些较大的数据" * 1000000
with open('output.txt', 'w') as file:
file.write(data)
1.2 使用 with
语句
使用 with
语句可以确保文件在操作完成后被正确关闭,避免文件资源泄露。
large_data = ["数据行1", "数据行2", "数据行3"] * 1000000
with open('output_large.txt', 'w') as f:
for line in large_data:
f.write(line + '\n')
二、使用数据持久化工具
2.1 使用 SQLite 数据库
SQLite 是一个轻量级的嵌入式数据库,适合存储较大数据并进行查询操作。
import sqlite3
连接到 SQLite 数据库
conn = sqlite3.connect('large_data.db')
cursor = conn.cursor()
创建表
cursor.execute('''
CREATE TABLE IF NOT EXISTS data (
id INTEGER PRIMARY KEY AUTOINCREMENT,
content TEXT
)
''')
插入大量数据
large_data = ["数据行1", "数据行2", "数据行3"] * 1000000
for line in large_data:
cursor.execute("INSERT INTO data (content) VALUES (?)", (line,))
提交事务并关闭连接
conn.commit()
conn.close()
2.2 使用 Pandas 和 CSV 文件
Pandas 是一个强大的数据分析库,可以方便地将大数据集导出到 CSV 文件中。
import pandas as pd
创建 DataFrame
large_data = ["数据行1", "数据行2", "数据行3"] * 1000000
df = pd.DataFrame(large_data, columns=['content'])
导出到 CSV 文件
df.to_csv('large_data.csv', index=False)
三、优化内存管理
3.1 使用生成器
生成器是一种高效的迭代器,可以在需要时生成数据,而不是一次性加载所有数据到内存中。
def generate_large_data():
for i in range(1000000):
yield f"数据行{i}"
with open('large_data_generated.txt', 'w') as f:
for line in generate_large_data():
f.write(line + '\n')
3.2 使用 yield
关键字
yield
关键字可以创建一个生成器函数,逐步生成数据,避免一次性加载大量数据到内存中。
def large_data_generator():
for i in range(1000000):
yield f"数据行{i}"
with open('output_yield.txt', 'w') as f:
for line in large_data_generator():
f.write(line + '\n')
四、使用序列化工具
4.1 使用 pickle
模块
pickle
模块可以将 Python 对象序列化到文件中,方便存储和传输大数据。
import pickle
large_data = ["数据行1", "数据行2", "数据行3"] * 1000000
with open('large_data.pkl', 'wb') as f:
pickle.dump(large_data, f)
4.2 使用 json
模块
json
模块可以将 Python 对象转化为 JSON 格式,适合存储和传输结构化数据。
import json
large_data = ["数据行1", "数据行2", "数据行3"] * 1000000
with open('large_data.json', 'w') as f:
json.dump(large_data, f)
五、分块处理大数据
5.1 读取大文件
在处理大文件时,可以分块读取文件,避免一次性加载所有数据到内存中。
def read_large_file(file_path, chunk_size=1024):
with open(file_path, 'r') as f:
while True:
data = f.read(chunk_size)
if not data:
break
yield data
for chunk in read_large_file('large_file.txt'):
process(chunk)
5.2 写入大文件
同样,在写入大文件时,也可以分块写入,避免一次性占用大量内存。
def write_large_file(file_path, data, chunk_size=1024):
with open(file_path, 'w') as f:
for i in range(0, len(data), chunk_size):
f.write(data[i:i + chunk_size])
large_data = "这里是一些较大的数据" * 1000000
write_large_file('large_output.txt', large_data)
六、使用并行处理
6.1 使用多线程
通过多线程可以同时处理多个数据块,提高处理大数据的效率。
import threading
def write_chunk(data_chunk, file_path):
with open(file_path, 'a') as f:
f.write(data_chunk)
large_data = ["数据行1", "数据行2", "数据行3"] * 1000000
chunk_size = 100000
threads = []
for i in range(0, len(large_data), chunk_size):
thread = threading.Thread(target=write_chunk, args=(large_data[i:i + chunk_size], 'large_output_threaded.txt'))
threads.append(thread)
thread.start()
for thread in threads:
thread.join()
6.2 使用多进程
使用多进程可以充分利用多核 CPU,提高处理大数据的性能。
import multiprocessing
def write_chunk(data_chunk, file_path):
with open(file_path, 'a') as f:
f.write(data_chunk)
large_data = ["数据行1", "数据行2", "数据行3"] * 1000000
chunk_size = 100000
processes = []
for i in range(0, len(large_data), chunk_size):
process = multiprocessing.Process(target=write_chunk, args=(large_data[i:i + chunk_size], 'large_output_multiprocessed.txt'))
processes.append(process)
process.start()
for process in processes:
process.join()
七、使用云存储服务
7.1 使用 Amazon S3
Amazon S3 是一个高度可扩展的云存储服务,可以用来存储和检索大数据。
import boto3
s3 = boto3.client('s3')
large_data = "这里是一些较大的数据" * 1000000
s3.put_object(Bucket='my-bucket', Key='large_data.txt', Body=large_data)
7.2 使用 Google Cloud Storage
Google Cloud Storage 是一个可扩展的对象存储服务,可以用来存储和检索大数据。
from google.cloud import storage
client = storage.Client()
bucket = client.get_bucket('my-bucket')
large_data = "这里是一些较大的数据" * 1000000
blob = bucket.blob('large_data.txt')
blob.upload_from_string(large_data)
八、使用消息队列
8.1 使用 RabbitMQ
RabbitMQ 是一个消息队列系统,可以用来分发和处理大数据。
import pika
connection = pika.BlockingConnection(pika.ConnectionParameters('localhost'))
channel = connection.channel()
channel.queue_declare(queue='large_data_queue')
large_data = ["数据行1", "数据行2", "数据行3"] * 1000000
for line in large_data:
channel.basic_publish(exchange='', routing_key='large_data_queue', body=line)
connection.close()
8.2 使用 Kafka
Kafka 是一个分布式流处理平台,可以用来处理和存储大数据。
from kafka import KafkaProducer
producer = KafkaProducer(bootstrap_servers='localhost:9092')
large_data = ["数据行1", "数据行2", "数据行3"] * 1000000
for line in large_data:
producer.send('large_data_topic', value=line.encode('utf-8'))
producer.flush()
producer.close()
通过以上多种方法,我们可以在 Python 中高效地输出和处理较大数据。选择合适的方法取决于具体的应用场景和需求。无论是简单的文件操作、使用数据库、优化内存管理、还是利用并行处理和云存储服务,都可以有效地解决大数据输出的问题。
相关问答FAQs:
如何在Python中处理和输出大量数据以确保效率?
在处理大数据时,使用合适的数据结构和算法非常重要。可以考虑使用生成器来逐步读取数据,而不是一次性将所有数据加载到内存中。这可以显著降低内存使用并提高程序的响应速度。还可以利用pandas库来高效地处理和输出数据,特别是在处理表格数据时。
Python中有没有推荐的库来处理大数据的输出?
确实有多个库可以帮助处理大数据的输出。pandas是一个非常强大的库,特别适合处理数据框和表格数据。对于需要处理更大数据集的情况,可以考虑使用Dask,它允许并行计算和懒加载。还有Apache PySpark,适合处理分布式数据集。
在Python中输出大量数据时,如何避免内存溢出?
为了避免内存溢出,建议使用分块读取和写入数据。使用pd.read_csv()
中的chunksize
参数可以分块读取CSV文件。对于输出,可以逐步写入文件,而不是将所有数据一次性加载到内存中。此外,使用更高效的数据格式,如Parquet或Feather,可以显著减少内存使用和提高读写速度。
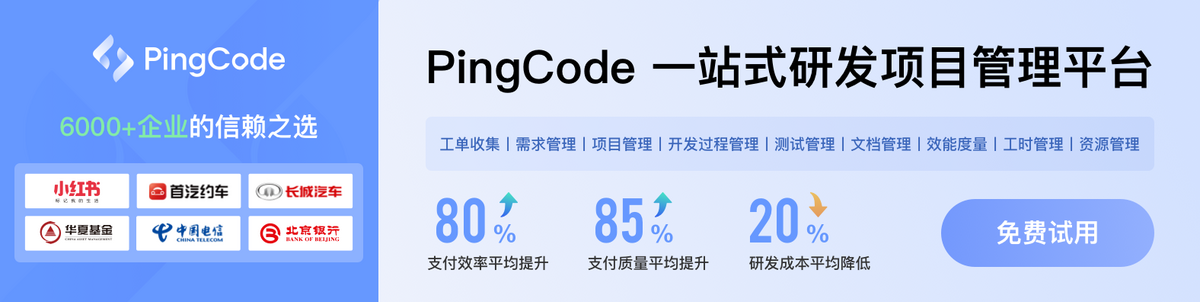