Python编写验证码识别程序的方法有很多,常见的有使用OCR技术、深度学习模型、图像处理等技术来实现。通过使用OCR技术、利用深度学习模型、结合图像处理技术,可以开发出一个有效的验证码识别程序。下面将详细介绍如何使用这些技术来编写一个高效的验证码识别程序。
一、OCR技术
OCR(Optical Character Recognition,光学字符识别)技术是将图像上的文字识别成可编辑文本的技术。Tesseract是一个广泛使用的开源OCR引擎,Python可以通过pytesseract库来调用。
1、安装Tesseract和pytesseract库
首先,需要安装Tesseract OCR和pytesseract库。
pip install pytesseract
pip install pillow
2、识别验证码
from PIL import Image
import pytesseract
加载图像
image = Image.open('captcha.png')
使用Tesseract OCR识别图像中的文字
text = pytesseract.image_to_string(image)
print(f"识别结果: {text}")
3、优化图像预处理
为了提高识别率,可以对图像进行一些预处理操作,如灰度化、二值化、去噪等。
import cv2
import numpy as np
def preprocess_image(image_path):
image = cv2.imread(image_path, cv2.IMREAD_GRAYSCALE)
_, thresh_image = cv2.threshold(image, 128, 255, cv2.THRESH_BINARY)
return thresh_image
preprocessed_image = preprocess_image('captcha.png')
text = pytesseract.image_to_string(preprocessed_image)
print(f"优化后的识别结果: {text}")
二、利用深度学习模型
深度学习模型,如卷积神经网络(CNN),在图像识别任务中表现出色。我们可以使用Keras或TensorFlow来训练一个自定义的验证码识别模型。
1、数据准备
首先需要准备训练数据,通常包括大量标注好的验证码图像。可以使用工具生成验证码图片,并手动或自动标注。
import os
import random
from captcha.image import ImageCaptcha
生成验证码图片
def generate_captcha(text, output_dir):
image = ImageCaptcha()
image.write(text, os.path.join(output_dir, f"{text}.png"))
示例生成
output_dir = 'captchas'
os.makedirs(output_dir, exist_ok=True)
for _ in range(1000):
text = ''.join(random.choices('ABCDEFGHIJKLMNOPQRSTUVWXYZ1234567890', k=5))
generate_captcha(text, output_dir)
2、数据预处理
将图像数据转换为适合输入神经网络的格式。
from tensorflow.keras.preprocessing.image import img_to_array, load_img
import numpy as np
def load_data(data_dir):
images = []
labels = []
for filename in os.listdir(data_dir):
if filename.endswith('.png'):
label = filename.split('.')[0]
image = load_img(os.path.join(data_dir, filename), color_mode='grayscale')
image = img_to_array(image)
images.append(image)
labels.append(label)
return np.array(images), np.array(labels)
images, labels = load_data(output_dir)
3、构建和训练模型
使用Keras构建卷积神经网络,并训练模型。
from tensorflow.keras.models import Sequential
from tensorflow.keras.layers import Conv2D, MaxPooling2D, Flatten, Dense
def build_model(input_shape):
model = Sequential()
model.add(Conv2D(32, (3, 3), activation='relu', input_shape=input_shape))
model.add(MaxPooling2D((2, 2)))
model.add(Conv2D(64, (3, 3), activation='relu'))
model.add(MaxPooling2D((2, 2)))
model.add(Flatten())
model.add(Dense(128, activation='relu'))
model.add(Dense(36, activation='softmax'))
return model
input_shape = (60, 160, 1) # 根据图像大小调整
model = build_model(input_shape)
model.compile(optimizer='adam', loss='categorical_crossentropy', metrics=['accuracy'])
将标签转换为one-hot编码
from tensorflow.keras.utils import to_categorical
labels_one_hot = to_categorical([int(c) for c in labels.flatten()])
model.fit(images, labels_one_hot, epochs=10, batch_size=32)
4、识别验证码
使用训练好的模型来识别新验证码。
def predict_captcha(model, image_path):
image = load_img(image_path, color_mode='grayscale', target_size=(60, 160))
image = img_to_array(image)
image = np.expand_dims(image, axis=0)
prediction = model.predict(image)
return ''.join(map(str, np.argmax(prediction, axis=1)))
captcha_text = predict_captcha(model, 'new_captcha.png')
print(f"预测结果: {captcha_text}")
三、结合图像处理技术
图像处理技术可以帮助我们预处理和增强验证码图像,从而提高识别率。
1、去噪
验证码图像中常常包含噪点,可以使用中值滤波、均值滤波等方法去噪。
def denoise_image(image_path):
image = cv2.imread(image_path, cv2.IMREAD_GRAYSCALE)
denoised_image = cv2.medianBlur(image, 3)
return denoised_image
denoised_image = denoise_image('captcha.png')
text = pytesseract.image_to_string(denoised_image)
print(f"去噪后的识别结果: {text}")
2、分割字符
有些验证码将字符连在一起,可以使用图像分割技术将每个字符单独提取出来。
def segment_characters(image):
contours, _ = cv2.findContours(image, cv2.RETR_EXTERNAL, cv2.CHAIN_APPROX_SIMPLE)
character_images = [cv2.boundingRect(contour) for contour in contours]
character_images = sorted(character_images, key=lambda x: x[0])
return character_images
segmented_characters = segment_characters(denoised_image)
for x, y, w, h in segmented_characters:
character_image = denoised_image[y:y+h, x:x+w]
cv2.imshow('Character', character_image)
cv2.waitKey(0)
四、总结
通过结合OCR技术、深度学习模型和图像处理技术,我们可以开发出一个高效的验证码识别程序。每种技术都有其优缺点,OCR技术简单易用,但识别率可能不高;深度学习模型可以达到高精度,但需要大量的标注数据和计算资源;图像处理技术可以辅助预处理图像,提升整体识别效果。根据具体应用场景选择合适的技术,并不断优化算法,才能实现高效的验证码识别。
相关问答FAQs:
如何选择合适的库来实现验证码识别?
在实现验证码识别时,常用的库包括Tesseract、OpenCV和Pillow。Tesseract是一个强大的OCR工具,适合处理文本识别;OpenCV则提供了图像处理的多种功能,可以帮助您预处理验证码图像;Pillow则用于基本的图像操作和处理。根据验证码的复杂程度和需求,选择合适的库将有助于提高识别的准确性。
验证码识别的准确率如何提高?
提高验证码识别的准确率可以通过多种方法实现。首先,进行图像预处理,例如去噪声、二值化和边缘检测,能够显著增强特征的清晰度。其次,训练自定义的深度学习模型也能提高特定验证码的识别能力。此外,使用数据增强技术,增加训练样本的多样性,能够帮助模型更好地适应不同的验证码样式。
在识别验证码时,如何处理复杂的背景和干扰元素?
对于背景复杂或含有干扰元素的验证码,可以采取多种策略。使用图像分割技术,可以有效提取出验证码的文本区域。对于干扰元素,利用形态学操作(如膨胀和腐蚀)能够帮助去除或减少这些噪声的影响。此外,结合深度学习方法,可以训练模型识别和忽略这些干扰,提升整体的识别效果。
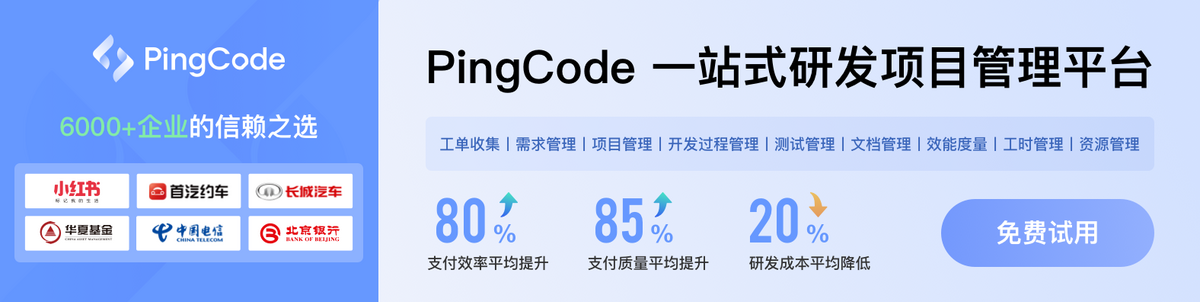