使用Python绘制查全率-查准率图
在计算机科学和数据科学领域,查全率(Recall)和查准率(Precision)是用于评估分类模型性能的重要指标。要绘制查全率-查准率图(Precision-Recall Curve),我们通常会使用Python中的常用数据科学库,如scikit-learn、matplotlib和numpy。首先,我们需要计算查全率和查准率,然后使用这些值绘制图表。下面,我们将详细介绍如何完成这一过程。
一、导入必要的库
首先,我们需要导入所需的Python库:
import numpy as np
import matplotlib.pyplot as plt
from sklearn.metrics import precision_recall_curve
from sklearn.metrics import average_precision_score
from sklearn.model_selection import train_test_split
from sklearn.datasets import make_classification
from sklearn.ensemble import RandomForestClassifier
这些库包括numpy用于数值计算,matplotlib用于绘图,scikit-learn中的函数用于计算查全率和查准率,并创建和评估机器学习模型。
二、创建数据集和模型
为了演示如何绘制查全率-查准率图,我们首先需要一个二分类数据集和一个分类模型。我们可以使用make_classification
函数来创建一个虚拟数据集,并使用随机森林分类器进行训练。
# 创建虚拟数据集
X, y = make_classification(n_samples=1000, n_features=20, n_classes=2, random_state=42)
将数据集分为训练集和测试集
X_train, X_test, y_train, y_test = train_test_split(X, y, test_size=0.3, random_state=42)
创建并训练随机森林分类器
clf = RandomForestClassifier(n_estimators=100, random_state=42)
clf.fit(X_train, y_train)
三、计算查全率和查准率
接下来,我们使用测试集预测结果计算查全率和查准率。
# 预测概率
y_scores = clf.predict_proba(X_test)[:, 1]
计算查全率和查准率
precision, recall, _ = precision_recall_curve(y_test, y_scores)
计算平均查全率
average_precision = average_precision_score(y_test, y_scores)
四、绘制查全率-查准率图
现在我们可以使用matplotlib来绘制查全率-查准率图。
# 绘制查全率-查准率图
plt.figure(figsize=(8, 6))
plt.plot(recall, precision, label=f'Random Forest (AP = {average_precision:.2f})')
plt.xlabel('Recall')
plt.ylabel('Precision')
plt.title('Precision-Recall Curve')
plt.legend(loc='best')
plt.grid()
plt.show()
这段代码将生成一个查全率-查准率图,显示模型在不同阈值下的查全率和查准率。
五、解释查全率-查准率图
查全率-查准率图的解读对于理解模型性能非常重要。在图中,每个点表示在某一阈值下的查全率和查准率。查全率表示模型识别出所有正例的比例,而查准率表示模型识别出的正例中实际为正例的比例。图中曲线越接近右上角,模型性能越好。
六、实例应用与扩展
为了进一步理解查全率-查准率图的应用,我们可以考虑以下几个实例应用:
1、不同模型的比较
我们可以比较不同模型的查全率-查准率曲线。例如,我们可以将逻辑回归、支持向量机和随机森林的查全率-查准率曲线放在同一个图中。
from sklearn.linear_model import LogisticRegression
from sklearn.svm import SVC
创建并训练逻辑回归模型
lr_clf = LogisticRegression(solver='liblinear')
lr_clf.fit(X_train, y_train)
lr_scores = lr_clf.predict_proba(X_test)[:, 1]
创建并训练支持向量机模型
svm_clf = SVC(probability=True)
svm_clf.fit(X_train, y_train)
svm_scores = svm_clf.predict_proba(X_test)[:, 1]
计算不同模型的查全率和查准率
lr_precision, lr_recall, _ = precision_recall_curve(y_test, lr_scores)
svm_precision, svm_recall, _ = precision_recall_curve(y_test, svm_scores)
绘制不同模型的查全率-查准率图
plt.figure(figsize=(8, 6))
plt.plot(recall, precision, label=f'Random Forest (AP = {average_precision:.2f})')
plt.plot(lr_recall, lr_precision, label=f'Logistic Regression (AP = {average_precision_score(y_test, lr_scores):.2f})')
plt.plot(svm_recall, svm_precision, label=f'SVM (AP = {average_precision_score(y_test, svm_scores):.2f})')
plt.xlabel('Recall')
plt.ylabel('Precision')
plt.title('Precision-Recall Curve Comparison')
plt.legend(loc='best')
plt.grid()
plt.show()
2、不同阈值对模型性能的影响
通过查全率-查准率曲线,我们可以观察不同阈值对模型性能的影响。在某些应用中,我们可能希望在高查全率和高查准率之间找到一个平衡点。
# 设置不同阈值
thresholds = np.linspace(0, 1, 100)
存储查全率和查准率
precisions = []
recalls = []
for threshold in thresholds:
y_pred = (y_scores >= threshold).astype(int)
tp = np.sum((y_test == 1) & (y_pred == 1))
fp = np.sum((y_test == 0) & (y_pred == 1))
fn = np.sum((y_test == 1) & (y_pred == 0))
precision = tp / (tp + fp) if (tp + fp) > 0 else 0
recall = tp / (tp + fn) if (tp + fn) > 0 else 0
precisions.append(precision)
recalls.append(recall)
绘制查全率-查准率图
plt.figure(figsize=(8, 6))
plt.plot(recalls, precisions, label='Precision-Recall Curve')
plt.xlabel('Recall')
plt.ylabel('Precision')
plt.title('Precision-Recall Curve with Different Thresholds')
plt.legend(loc='best')
plt.grid()
plt.show()
七、总结
查全率-查准率图是评估分类模型性能的重要工具。通过这种图表,我们可以直观地看到模型在不同阈值下的查全率和查准率表现。本文详细介绍了如何使用Python绘制查全率-查准率图,并解释了如何在实际应用中使用这些图表进行模型评估和优化。
在实际应用中,查全率和查准率的权衡取决于具体的业务需求。例如,在医疗诊断中,可能需要更高的查全率以确保不漏掉任何阳性病例,而在垃圾邮件过滤中,可能更需要更高的查准率以减少误报。通过查全率-查准率图,我们可以更好地理解和优化模型,以满足特定应用的需求。
相关问答FAQs:
如何用Python绘制查全率和查准率图?
要绘制查全率和查准率图,可以使用Matplotlib和Scikit-learn库。首先,计算模型的查全率和查准率,然后使用Matplotlib绘制曲线。代码示例如下:
import matplotlib.pyplot as plt
from sklearn.metrics import precision_recall_curve
# 假设y_true是实际标签,y_scores是模型预测的分数
precision, recall, _ = precision_recall_curve(y_true, y_scores)
plt.plot(recall, precision, marker='.')
plt.xlabel('Recall')
plt.ylabel('Precision')
plt.title('Precision-Recall Curve')
plt.grid()
plt.show()
查全率和查准率的定义是什么?
查全率(Recall)是指正确预测的正样本占所有实际正样本的比例,反映了模型捕捉正样本的能力。查准率(Precision)是指正确预测的正样本占所有预测为正样本的比例,反映了模型的准确性。两者都是评估分类模型性能的重要指标。
在Python中如何获取查全率和查准率的数值?
可以使用Scikit-learn库中的precision_recall_curve
函数来计算查全率和查准率。该函数需要实际标签和预测分数作为输入,并返回相应的查全率、查准率及阈值。示例代码如下:
from sklearn.metrics import precision_recall_curve
precision, recall, thresholds = precision_recall_curve(y_true, y_scores)
使用查全率和查准率图可以帮助我们做什么?
查全率和查准率图能够帮助我们直观地了解模型在不同阈值下的表现。通过观察曲线的形状,可以评估模型在处理不同类别时的平衡情况,帮助选择最适合特定任务的阈值,从而优化模型的性能。
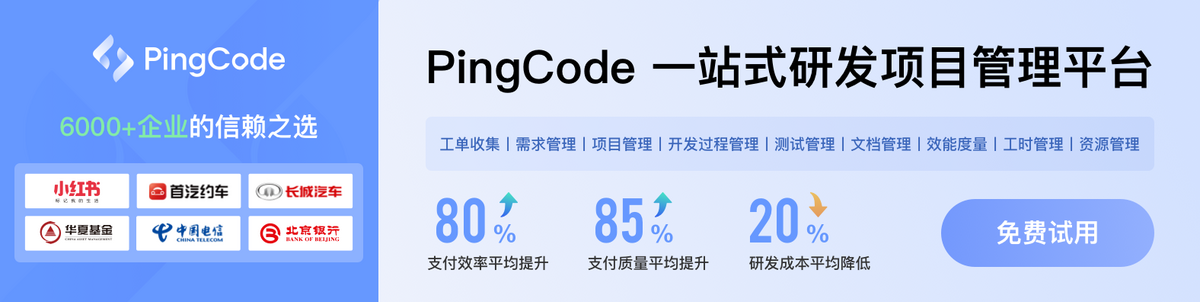