用Python做决策树的方法包括数据准备、特征选择、树的构建、剪枝和模型评估。本文将详细介绍如何实现这些步骤。
一、数据准备
在进行任何机器学习任务之前,数据准备是至关重要的步骤。数据准备包括数据清洗、特征选择以及数据分割等。
- 数据清洗:数据清洗是指删除数据集中不必要的数据,处理缺失值和异常值等操作。可以使用Pandas库进行数据清洗。
import pandas as pd
读取数据
data = pd.read_csv('data.csv')
检查缺失值
print(data.isnull().sum())
填充缺失值或删除
data.fillna(method='ffill', inplace=True)
- 特征选择:选择对决策树有用的特征,特征选择可以通过相关性分析、特征重要性等方法进行。
# 相关性分析
correlation_matrix = data.corr()
print(correlation_matrix)
手动选择特征
features = ['feature1', 'feature2', 'feature3']
X = data[features]
y = data['target']
- 数据分割:将数据集分为训练集和测试集。
from sklearn.model_selection import train_test_split
X_train, X_test, y_train, y_test = train_test_split(X, y, test_size=0.2, random_state=42)
二、特征选择
特征选择是构建决策树的关键步骤。特征选择算法有很多,常见的有熵增益、基尼指数等。
- 信息增益:信息增益是基于熵的一个特征选择方法,通常用于分类任务。
import numpy as np
def entropy(y):
class_labels = np.unique(y)
entropy = 0
for cls in class_labels:
p_cls = len(y[y == cls]) / len(y)
entropy -= p_cls * np.log2(p_cls)
return entropy
def information_gain(X, y, feature):
unique_values = np.unique(X[feature])
total_entropy = entropy(y)
weighted_entropy = 0
for value in unique_values:
subset_y = y[X[feature] == value]
weighted_entropy += (len(subset_y) / len(y)) * entropy(subset_y)
return total_entropy - weighted_entropy
计算每个特征的信息增益
for feature in features:
print(f"Information Gain for {feature}: {information_gain(X_train, y_train, feature)}")
三、树的构建
- 使用Scikit-Learn构建决策树:Scikit-Learn提供了一个非常方便的决策树实现。
from sklearn.tree import DecisionTreeClassifier
构建决策树
clf = DecisionTreeClassifier(criterion='entropy', max_depth=5, random_state=42)
clf.fit(X_train, y_train)
预测
y_pred = clf.predict(X_test)
- 可视化决策树:使用Graphviz库进行决策树的可视化。
from sklearn.tree import export_graphviz
import graphviz
dot_data = export_graphviz(clf, out_file=None,
feature_names=features,
class_names=['class0', 'class1'],
filled=True, rounded=True,
special_characters=True)
graph = graphviz.Source(dot_data)
graph.render("decision_tree")
四、剪枝
剪枝是防止决策树过拟合的一个重要步骤。剪枝的方法有预剪枝和后剪枝。
- 预剪枝:预剪枝是在构建决策树的过程中,通过设置参数来限制树的生长。
clf = DecisionTreeClassifier(criterion='entropy', max_depth=5, min_samples_split=10, random_state=42)
clf.fit(X_train, y_train)
- 后剪枝:后剪枝是在构建完决策树后,通过评估子树的性能来剪去一些子树。
from sklearn.tree import DecisionTreeClassifier
from sklearn.model_selection import cross_val_score
path = clf.cost_complexity_pruning_path(X_train, y_train)
ccp_alphas, impurities = path.ccp_alphas, path.impurities
找到最佳剪枝参数
clf = DecisionTreeClassifier(random_state=42)
clf.fit(X_train, y_train)
alpha_scores = [(alpha, cross_val_score(clf.set_params(ccp_alpha=alpha), X_train, y_train, cv=5).mean()) for alpha in ccp_alphas]
best_alpha = max(alpha_scores, key=lambda x: x[1])[0]
使用最佳剪枝参数重新训练模型
clf = DecisionTreeClassifier(random_state=42, ccp_alpha=best_alpha)
clf.fit(X_train, y_train)
五、模型评估
模型评估是验证决策树性能的重要步骤。常见的评估指标包括准确率、精确率、召回率、F1值等。
from sklearn.metrics import accuracy_score, precision_score, recall_score, f1_score, classification_report
预测
y_pred = clf.predict(X_test)
评估
print(f"Accuracy: {accuracy_score(y_test, y_pred)}")
print(f"Precision: {precision_score(y_test, y_pred, average='weighted')}")
print(f"Recall: {recall_score(y_test, y_pred, average='weighted')}")
print(f"F1 Score: {f1_score(y_test, y_pred, average='weighted')}")
print(classification_report(y_test, y_pred))
通过以上步骤,我们完成了一个简单的决策树模型的构建、训练和评估。这只是决策树的基础应用,实际应用中还可以根据具体问题进行更多的优化和调整。
相关问答FAQs:
决策树是什么,为什么选择使用决策树?
决策树是一种常用的机器学习算法,用于分类和回归任务。它通过将数据分割成不同的部分,形成树状结构,来帮助模型做出决策。选择决策树的原因包括其易于理解和解释,能够处理非线性关系,并且对缺失数据具有一定的鲁棒性。
在Python中构建决策树的步骤有哪些?
在Python中构建决策树通常包括以下几个步骤:首先,导入必要的库,如pandas
、numpy
和scikit-learn
;其次,加载并预处理数据,包括处理缺失值和特征选择;接着,使用DecisionTreeClassifier
或DecisionTreeRegressor
创建决策树模型;最后,使用训练数据拟合模型并进行预测。
如何评估决策树模型的性能?
评估决策树模型的性能可以通过几种方法。可以使用交叉验证来判断模型的稳健性,计算准确率、召回率和F1分数来评估分类模型的效果。同时,可以生成混淆矩阵以了解模型在不同类别上的表现。对于回归模型,则可以使用均方误差(MSE)和决定系数(R²)来衡量预测精度。
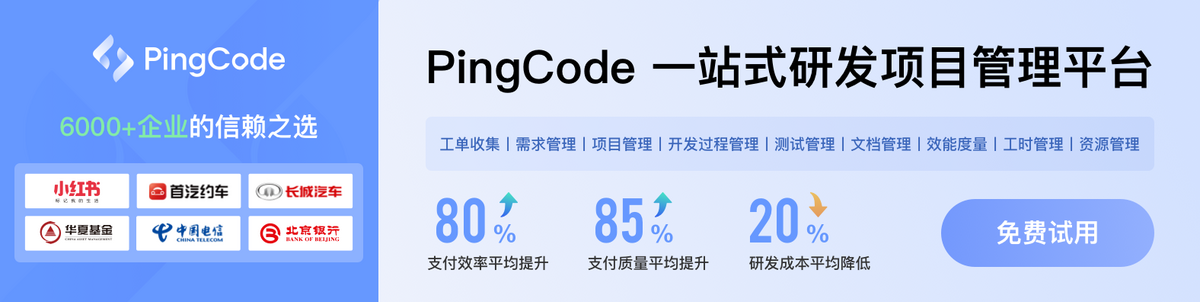