通过Python找时间序列的波峰个数,可以使用库函数、信号处理技术和统计方法。常用的方法包括:使用SciPy库中的find_peaks函数、手动实现峰值检测、结合滑动窗口和阈值法等。其中,使用SciPy库中的find_peaks函数是最直接和高效的方法。下面我们详细介绍这种方法。
一、SciPy库中的find_peaks函数
SciPy库中的find_peaks函数是一个强大的工具,可以方便地检测时间序列中的波峰。首先,我们需要安装SciPy库,然后可以使用以下代码来检测波峰的个数。
import numpy as np
import matplotlib.pyplot as plt
from scipy.signal import find_peaks
生成一个示例时间序列数据
np.random.seed(10)
x = np.linspace(0, 10, 100)
y = np.sin(x) + np.random.normal(0, 0.1, 100)
使用find_peaks函数检测波峰
peaks, _ = find_peaks(y)
打印波峰的个数
print(f"波峰的个数: {len(peaks)}")
绘制时间序列和波峰
plt.plot(x, y)
plt.plot(x[peaks], y[peaks], "x")
plt.show()
find_peaks函数的参数和返回值
find_peaks函数的主要参数有:
- x:输入的时间序列数据。
- height:指定峰值的最低高度。
- threshold:指定峰值的最低阈值。
- distance:指定相邻峰值之间的最小距离。
返回值包括:
- peaks:检测到的峰值的索引。
- properties:包含峰值的属性信息。
可以根据具体需求调整这些参数,以提高峰值检测的准确性。
二、手动实现峰值检测
在某些情况下,使用库函数可能无法满足特定的需求,我们可以手动实现峰值检测算法。这种方法的核心思想是比较时间序列中每个点的值与其相邻点的值。
def find_peaks_manual(y):
peaks = []
for i in range(1, len(y) - 1):
if y[i] > y[i - 1] and y[i] > y[i + 1]:
peaks.append(i)
return peaks
使用手动实现的函数检测波峰
peaks_manual = find_peaks_manual(y)
打印波峰的个数
print(f"手动检测的波峰个数: {len(peaks_manual)}")
绘制时间序列和波峰
plt.plot(x, y)
plt.plot(x[peaks_manual], y[peaks_manual], "x")
plt.show()
三、滑动窗口和阈值法
滑动窗口和阈值法是一种常用于平滑和去噪的方法,可以结合使用来检测波峰。滑动窗口用于平滑时间序列数据,而阈值法用于检测超过特定阈值的点。
def smooth(y, window_size):
return np.convolve(y, np.ones(window_size) / window_size, mode='valid')
def find_peaks_threshold(y, threshold):
peaks = []
for i in range(1, len(y) - 1):
if y[i] > threshold and y[i] > y[i - 1] and y[i] > y[i + 1]:
peaks.append(i)
return peaks
使用滑动窗口平滑数据
y_smooth = smooth(y, window_size=5)
使用阈值法检测波峰
threshold = 0.5
peaks_threshold = find_peaks_threshold(y_smooth, threshold)
打印波峰的个数
print(f"阈值法检测的波峰个数: {len(peaks_threshold)}")
绘制时间序列和平滑后的数据及波峰
plt.plot(x, y, label='Original')
plt.plot(x[2:-2], y_smooth, label='Smoothed')
plt.plot(x[peaks_threshold], y_smooth[peaks_threshold], "x", label='Peaks')
plt.legend()
plt.show()
四、结合多种方法提高检测准确性
在实际应用中,单一的方法可能无法满足所有需求,可以结合多种方法以提高波峰检测的准确性。例如,先使用滑动窗口平滑数据,再使用find_peaks函数检测波峰。
# 使用滑动窗口平滑数据
y_smooth_combined = smooth(y, window_size=5)
使用find_peaks函数检测波峰
peaks_combined, _ = find_peaks(y_smooth_combined, height=0.5)
打印波峰的个数
print(f"组合方法检测的波峰个数: {len(peaks_combined)}")
绘制时间序列和平滑后的数据及波峰
plt.plot(x, y, label='Original')
plt.plot(x[2:-2], y_smooth_combined, label='Smoothed')
plt.plot(x[peaks_combined], y_smooth_combined[peaks_combined], "x", label='Peaks')
plt.legend()
plt.show()
五、总结
Python提供了多种方法来检测时间序列中的波峰,包括使用SciPy库中的find_peaks函数、手动实现峰值检测、滑动窗口和阈值法等。使用这些方法时,可以根据具体需求调整参数以提高检测的准确性。此外,结合多种方法可以进一步提高波峰检测的效果。在实际应用中,选择合适的方法和参数是至关重要的,应该根据具体的时间序列数据特点进行调整和优化。
相关问答FAQs:
如何使用Python库来识别时间序列中的波峰?
在Python中,可以使用SciPy库中的find_peaks
函数来识别时间序列中的波峰。该函数可以处理一维数组,返回波峰的索引。通过设置不同的参数,如最小高度、最小距离等,可以精确控制波峰的检测。此外,Pandas库可以方便地处理时间序列数据,结合Matplotlib进行可视化,可以更直观地观察波峰的分布。
我应该如何准备我的时间序列数据以便找出波峰?
在找出波峰之前,确保你的时间序列数据已被整理为一个一维的数组格式。如果数据包含缺失值,建议先进行插值处理。使用Pandas可以轻松清理数据,确保数据的连续性和一致性。此外,对数据进行标准化或平滑处理可以提高波峰检测的准确性。
检测到的波峰如何进行后续分析?
一旦检测到波峰,可以进行多种后续分析。例如,可以计算波峰之间的间隔时间,以评估周期性;也可以分析波峰的高度变化,以识别趋势。此外,结合其他统计指标,如波谷、均值和标准差,可以深入了解时间序列的行为模式,帮助做出更好的决策。
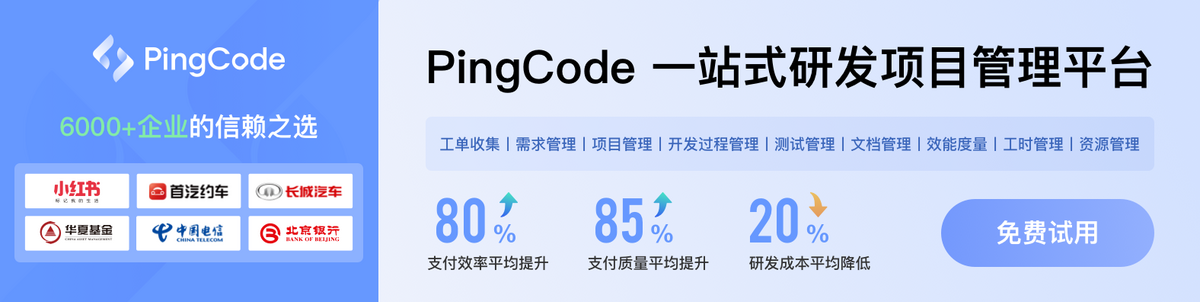