Python构建决策树的节点可以通过以下几个步骤:定义节点类、计算信息增益、选择最佳分割属性、递归构建树,其中,定义节点类是最关键的一步。下面将详细描述如何通过这几个步骤来构建决策树节点。
一、定义节点类
在构建决策树的过程中,我们需要定义一个节点类来表示树中的每个节点。这个类通常包含以下几个属性:分割属性、分割值、左子节点、右子节点、以及当前节点的类标签(对于叶节点)。
class Node:
def __init__(self, gini, num_samples, num_samples_per_class, predicted_class):
self.gini = gini
self.num_samples = num_samples
self.num_samples_per_class = num_samples_per_class
self.predicted_class = predicted_class
self.feature_index = 0
self.threshold = 0
self.left = None
self.right = None
二、计算信息增益
信息增益是决策树算法用于选择分割属性的标准之一。信息增益可以通过计算数据集的熵和分割后的熵来获得。
def entropy(y):
hist = np.bincount(y)
ps = hist / len(y)
return -np.sum([p * np.log2(p) for p in ps if p > 0])
def information_gain(y, y_left, y_right):
p = len(y_left) / len(y)
return entropy(y) - p * entropy(y_left) - (1 - p) * entropy(y_right)
三、选择最佳分割属性
选择最佳分割属性是决策树算法的重要步骤。我们需要遍历所有的特征和可能的分割点,并计算每个分割的熵值,选择使信息增益最大的分割点。
def best_split(X, y):
m, n = X.shape
if m <= 1:
return None, None
num_parent = [np.sum(y == c) for c in range(num_classes)]
best_gini = 1.0 - sum((num / m) 2 for num in num_parent)
best_idx, best_thr = None, None
for idx in range(n):
thresholds, classes = zip(*sorted(zip(X[:, idx], y)))
num_left = [0] * num_classes
num_right = num_parent.copy()
for i in range(1, m):
c = classes[i - 1]
num_left[c] += 1
num_right[c] -= 1
gini_left = 1.0 - sum((num_left[x] / i) 2 for x in range(num_classes))
gini_right = 1.0 - sum((num_right[x] / (m - i)) 2 for x in range(num_classes))
gini = (i * gini_left + (m - i) * gini_right) / m
if thresholds[i] == thresholds[i - 1]:
continue
if gini < best_gini:
best_gini = gini
best_idx = idx
best_thr = (thresholds[i] + thresholds[i - 1]) / 2
return best_idx, best_thr
四、递归构建树
通过递归地创建节点并分割数据集,我们可以构建整个决策树。
def grow_tree(X, y, depth=0):
num_samples_per_class = [np.sum(y == i) for i in range(num_classes)]
predicted_class = np.argmax(num_samples_per_class)
node = Node(
gini=gini(y),
num_samples=len(y),
num_samples_per_class=num_samples_per_class,
predicted_class=predicted_class,
)
if depth < max_depth:
idx, thr = best_split(X, y)
if idx is not None:
indices_left = X[:, idx] < thr
X_left, y_left = X[indices_left], y[indices_left]
X_right, y_right = X[~indices_left], y[~indices_left]
node.feature_index = idx
node.threshold = thr
node.left = grow_tree(X_left, y_left, depth + 1)
node.right = grow_tree(X_right, y_right, depth + 1)
return node
五、预测
最后,我们需要实现一个预测函数,用于对新数据进行分类。
def predict(inputs, tree):
while tree.left:
if inputs[tree.feature_index] < tree.threshold:
tree = tree.left
else:
tree = tree.right
return tree.predicted_class
总结
以上就是Python构建决策树节点的详细步骤。我们从定义节点类开始,介绍了如何计算信息增益,选择最佳分割属性,递归构建树,以及如何对新数据进行预测。通过这些步骤,你可以构建一个简单但功能强大的决策树模型。
相关问答FAQs:
决策树的节点在Python中是如何实现的?
构建决策树的节点通常涉及到对数据进行划分和决策规则的制定。在Python中,我们可以使用scikit-learn
库来实现决策树的构建。具体来说,决策树的每个节点代表一个特征的测试,每个分支代表测试结果的不同,最终的叶子节点则代表输出的类别或回归值。通过DecisionTreeClassifier
或DecisionTreeRegressor
类,可以方便地创建决策树,使用fit()
方法训练模型,并通过predict()
方法进行预测。
如何选择决策树的最佳分割点?
选择最佳分割点通常依赖于某种评价标准,例如信息增益、基尼指数或均方误差等。在scikit-learn
中,DecisionTreeClassifier
和DecisionTreeRegressor
会自动为每个节点计算这些指标,以选择最优的特征和分割点。用户可以通过调整参数,如criterion
,来选择不同的评估标准,从而优化决策树的性能。
如何防止决策树过拟合?
决策树容易出现过拟合,尤其是当树的深度较大时。为了防止过拟合,可以采取几种策略:限制树的深度(使用max_depth
参数)、设置最小样本分割数(min_samples_split
)、最小叶子节点样本数(min_samples_leaf
)等。此外,可以考虑使用集成学习方法,如随机森林,来提高模型的泛化能力,通过组合多棵树的预测结果来减少过拟合的风险。
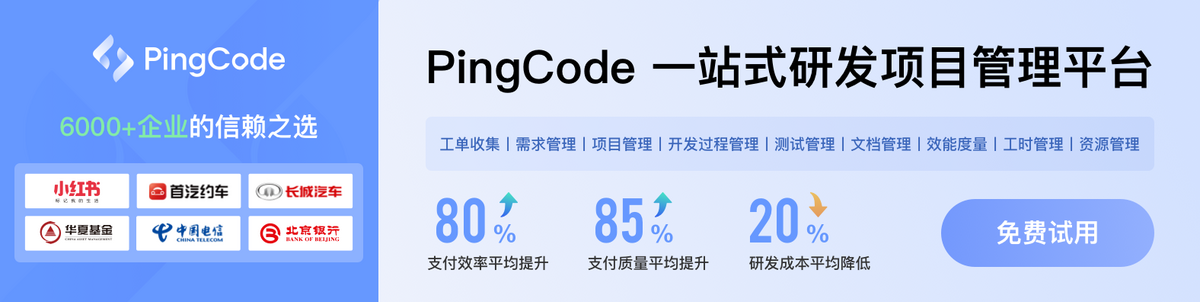