开头段落:
Python命令行制作美颜可以通过使用OpenCV库、利用dlib库进行人脸识别、使用图像处理技术进行美颜。其中,利用dlib库进行人脸识别是其中的一个关键步骤,它可以帮助我们精确定位人脸的各个特征点,从而更好地进行图像处理。通过这些步骤,我们可以在Python命令行中实现基本的美颜效果。
一、使用OpenCV库
OpenCV(Open Source Computer Vision Library)是一个开源计算机视觉和机器学习软件库。它提供了超过2500个优化的算法,可以用于各种图像和视频处理操作。
- 安装OpenCV库
pip install opencv-python
- 读取和显示图像
import cv2
读取图像
image = cv2.imread('path_to_image.jpg')
显示图像
cv2.imshow('Original Image', image)
cv2.waitKey(0)
cv2.destroyAllWindows()
二、利用dlib库进行人脸识别
dlib库是一个现代化的C++工具包,包含了机器学习算法和工具,特别擅长人脸检测和人脸特征点定位。
- 安装dlib库
pip install dlib
- 加载预训练的人脸识别模型
import dlib
加载dlib预训练的人脸检测模型
detector = dlib.get_frontal_face_detector()
加载dlib预训练的人脸特征点检测模型
predictor = dlib.shape_predictor('shape_predictor_68_face_landmarks.dat')
- 检测人脸并标记特征点
# 检测人脸
faces = detector(image, 1)
for face in faces:
# 获取人脸特征点
landmarks = predictor(image, face)
# 绘制特征点
for n in range(0, 68):
x = landmarks.part(n).x
y = landmarks.part(n).y
cv2.circle(image, (x, y), 2, (0, 255, 0), -1)
cv2.imshow('Landmarks', image)
cv2.waitKey(0)
cv2.destroyAllWindows()
三、图像处理技术进行美颜
在获得了人脸特征点之后,我们可以应用各种图像处理技术来实现美颜效果,比如磨皮、美白、瘦脸等。
- 磨皮(平滑处理)
# 将图像转换为灰度图
gray_image = cv2.cvtColor(image, cv2.COLOR_BGR2GRAY)
使用高斯模糊来平滑皮肤
smooth_image = cv2.GaussianBlur(gray_image, (15, 15), 0)
将平滑后的图像与原图像融合
alpha = 0.5
beautified_image = cv2.addWeighted(image, alpha, smooth_image, 1 - alpha, 0)
cv2.imshow('Beautified Image', beautified_image)
cv2.waitKey(0)
cv2.destroyAllWindows()
- 美白(亮度和对比度调整)
# 调整亮度和对比度
alpha = 1.2 # 对比度
beta = 30 # 亮度
beautified_image = cv2.convertScaleAbs(image, alpha=alpha, beta=beta)
cv2.imshow('Whitened Image', beautified_image)
cv2.waitKey(0)
cv2.destroyAllWindows()
- 瘦脸(基于特征点的仿射变换)
def apply_transformation(image, src_points, dst_points):
# 计算仿射变换矩阵
matrix = cv2.getAffineTransform(src_points, dst_points)
# 应用仿射变换
transformed_image = cv2.warpAffine(image, matrix, (image.shape[1], image.shape[0]))
return transformed_image
示例:将人脸下巴部分收窄
src_points = np.float32([
[landmarks.part(3).x, landmarks.part(3).y], # 左颚角
[landmarks.part(13).x, landmarks.part(13).y], # 右颚角
[landmarks.part(8).x, landmarks.part(8).y] # 下巴
])
dst_points = np.float32([
[landmarks.part(3).x, landmarks.part(3).y],
[landmarks.part(13).x, landmarks.part(13).y],
[landmarks.part(8).x, landmarks.part(8).y - 10] # 将下巴向上移动
])
beautified_image = apply_transformation(image, src_points, dst_points)
cv2.imshow('Slimmed Face Image', beautified_image)
cv2.waitKey(0)
cv2.destroyAllWindows()
四、综合应用
通过组合上述技术,我们可以在Python命令行中实现一个简单的美颜程序。
- 完整示例代码
import cv2
import dlib
import numpy as np
读取图像
image = cv2.imread('path_to_image.jpg')
加载dlib模型
detector = dlib.get_frontal_face_detector()
predictor = dlib.shape_predictor('shape_predictor_68_face_landmarks.dat')
检测人脸
faces = detector(image, 1)
for face in faces:
# 获取人脸特征点
landmarks = predictor(image, face)
# 绘制特征点
for n in range(0, 68):
x = landmarks.part(n).x
y = landmarks.part(n).y
cv2.circle(image, (x, y), 2, (0, 255, 0), -1)
# 磨皮
gray_image = cv2.cvtColor(image, cv2.COLOR_BGR2GRAY)
smooth_image = cv2.GaussianBlur(gray_image, (15, 15), 0)
alpha = 0.5
beautified_image = cv2.addWeighted(image, alpha, smooth_image, 1 - alpha, 0)
# 美白
alpha = 1.2
beta = 30
beautified_image = cv2.convertScaleAbs(beautified_image, alpha=alpha, beta=beta)
# 瘦脸
def apply_transformation(image, src_points, dst_points):
matrix = cv2.getAffineTransform(src_points, dst_points)
transformed_image = cv2.warpAffine(image, matrix, (image.shape[1], image.shape[0]))
return transformed_image
src_points = np.float32([
[landmarks.part(3).x, landmarks.part(3).y],
[landmarks.part(13).x, landmarks.part(13).y],
[landmarks.part(8).x, landmarks.part(8).y]
])
dst_points = np.float32([
[landmarks.part(3).x, landmarks.part(3).y],
[landmarks.part(13).x, landmarks.part(13).y],
[landmarks.part(8).x, landmarks.part(8).y - 10]
])
beautified_image = apply_transformation(beautified_image, src_points, dst_points)
cv2.imshow('Beautified Image', beautified_image)
cv2.waitKey(0)
cv2.destroyAllWindows()
通过以上步骤,我们可以在Python命令行中实现基本的美颜效果。这个过程不仅展示了如何使用OpenCV和dlib进行图像处理和人脸识别,还展示了如何应用图像处理技术来实现美颜效果。希望这篇文章对你有所帮助。
相关问答FAQs:
如何在Python命令行中使用美颜效果?
在Python命令行中实现美颜效果通常需要借助图像处理库,例如OpenCV或PIL。可以使用这些库来读取图像,并应用平滑、模糊等效果来达到美颜的目的。具体步骤包括安装相关库、读取图像、应用美颜滤镜和保存结果。可以通过简单的代码示例来实现这一过程。
使用Python命令行制作美颜效果需要哪些库?
要在Python命令行中制作美颜效果,建议使用OpenCV和NumPy。OpenCV提供丰富的图像处理功能,而NumPy用于高效的数组操作。可以通过pip install opencv-python numpy
命令安装这两个库。安装后,便可以编写脚本,加载图像并应用各种美颜效果。
在Python命令行中实现美颜效果的代码示例是什么?
一个简单的美颜效果代码示例如下:
import cv2
import numpy as np
# 读取图像
image = cv2.imread('input.jpg')
# 应用高斯模糊
beautified_image = cv2.GaussianBlur(image, (15, 15), 0)
# 保存结果
cv2.imwrite('output.jpg', beautified_image)
上述代码将读取一张名为“input.jpg”的图像,使用高斯模糊处理后保存为“output.jpg”。可以根据需求调整模糊参数,以获得理想的美颜效果。
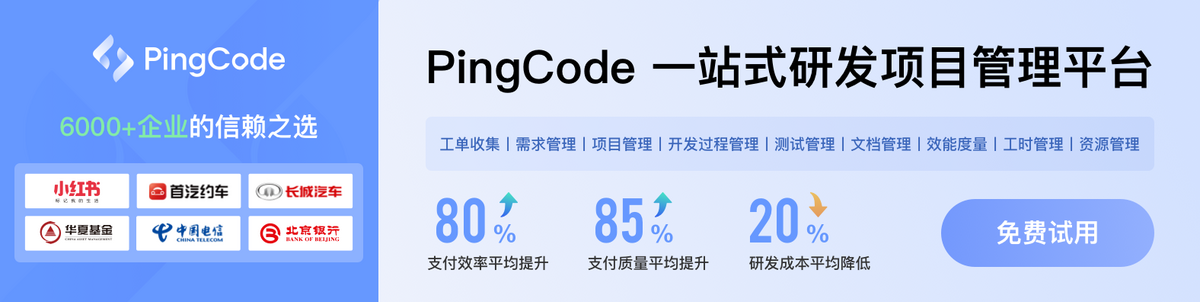