如何让Python一直爬取
使用循环、处理异常、设置合理的间隔时间、优化性能、使用代理、重试机制。其中使用循环是最重要的。循环是确保Python脚本能够持续运行的关键,通过使用无限循环(如while True
)可以使脚本不断执行爬取操作。下面详细描述如何实现这一点。
一、使用循环
在Python中,最常用的方式是使用while
循环来实现持续爬取。while True
循环会一直运行,直到手动停止脚本或遇到特定的条件。下面是一个简单的例子:
import time
while True:
# 爬取任务
print("正在爬取数据...")
# 设置间隔时间,防止被封禁
time.sleep(10)
这个脚本会每10秒钟执行一次爬取任务,直到被手动终止。实际应用中,可以将爬取任务封装成一个函数,并在循环中调用该函数。
二、处理异常
爬虫过程中可能会遇到各种异常,如网络错误、服务器响应错误等。如果不处理这些异常,脚本可能会意外终止。使用try-except
结构可以捕获并处理异常,从而确保脚本能够继续运行。
import time
import requests
def fetch_data():
try:
response = requests.get('https://example.com')
response.raise_for_status() # 检查是否有请求错误
return response.text
except requests.exceptions.RequestException as e:
print(f"请求错误: {e}")
return None
while True:
data = fetch_data()
if data:
print("数据爬取成功")
else:
print("数据爬取失败,等待重试")
time.sleep(10)
三、设置合理的间隔时间
频繁的请求可能会导致IP被封禁,因此设置合理的间隔时间非常重要。可以根据目标网站的响应时间和访问限制来调整间隔时间。
import random
while True:
data = fetch_data()
if data:
print("数据爬取成功")
else:
print("数据爬取失败,等待重试")
time.sleep(random.uniform(5, 15)) # 随机等待5到15秒
四、优化性能
对于大规模的爬取任务,可以考虑使用并发技术来提高效率。Python的threading
和multiprocessing
模块可以帮助实现这一点。下面是一个使用threading
的例子:
import threading
def fetch_data():
# 爬取任务
pass
def worker():
while True:
fetch_data()
time.sleep(random.uniform(5, 15))
threads = []
for i in range(5): # 创建5个线程
t = threading.Thread(target=worker)
t.start()
threads.append(t)
for t in threads:
t.join()
五、使用代理
使用代理可以避免IP被封禁。代理服务器可以隐藏真实IP地址,并且可以轮换使用多个代理IP。
import requests
proxies = {
'http': 'http://your_proxy_ip:port',
'https': 'https://your_proxy_ip:port',
}
def fetch_data():
try:
response = requests.get('https://example.com', proxies=proxies)
response.raise_for_status()
return response.text
except requests.exceptions.RequestException as e:
print(f"请求错误: {e}")
return None
六、重试机制
在某些情况下,爬取任务可能会失败。为了确保数据的完整性,可以设置重试机制。
import time
import requests
def fetch_data():
for i in range(5): # 重试5次
try:
response = requests.get('https://example.com')
response.raise_for_status()
return response.text
except requests.exceptions.RequestException as e:
print(f"请求错误: {e}, 正在重试({i+1}/5)")
time.sleep(5)
return None
while True:
data = fetch_data()
if data:
print("数据爬取成功")
else:
print("数据爬取失败,等待重试")
time.sleep(10)
七、数据存储与管理
在持续爬取过程中,收集到的数据需要进行有效的存储和管理。可以将数据存储到数据库中,如MySQL、MongoDB等,以便后续处理和分析。
import pymysql
def save_data(data):
connection = pymysql.connect(host='localhost',
user='user',
password='passwd',
db='database',
charset='utf8mb4')
try:
with connection.cursor() as cursor:
sql = "INSERT INTO `table` (`field1`, `field2`) VALUES (%s, %s)"
cursor.execute(sql, (data['field1'], data['field2']))
connection.commit()
finally:
connection.close()
while True:
data = fetch_data()
if data:
save_data(data)
print("数据爬取并保存成功")
else:
print("数据爬取失败,等待重试")
time.sleep(10)
八、日志记录
在长时间运行的爬取任务中,日志记录是非常重要的。通过记录日志,可以了解脚本的运行情况、错误信息等,有助于调试和优化。
import logging
logging.basicConfig(filename='scraper.log', level=logging.INFO)
def fetch_data():
try:
response = requests.get('https://example.com')
response.raise_for_status()
logging.info('数据爬取成功')
return response.text
except requests.exceptions.RequestException as e:
logging.error(f"请求错误: {e}")
return None
while True:
data = fetch_data()
if data:
print("数据爬取成功")
else:
print("数据爬取失败,等待重试")
time.sleep(10)
九、监控与报警
为了确保爬虫的稳定运行,可以设置监控与报警机制。当脚本出现异常时,可以及时发送通知。可以使用诸如Prometheus、Grafana等监控工具,或者通过邮件、短信等方式发送报警。
import smtplib
from email.mime.text import MIMEText
def send_alert(message):
msg = MIMEText(message)
msg['Subject'] = '爬虫脚本报警'
msg['From'] = 'your_email@example.com'
msg['To'] = 'alert_email@example.com'
with smtplib.SMTP('smtp.example.com') as server:
server.login('your_email@example.com', 'your_password')
server.sendmail(msg['From'], [msg['To']], msg.as_string())
def fetch_data():
try:
response = requests.get('https://example.com')
response.raise_for_status()
return response.text
except requests.exceptions.RequestException as e:
send_alert(f"爬虫脚本出现错误: {e}")
return None
while True:
data = fetch_data()
if data:
print("数据爬取成功")
else:
print("数据爬取失败,等待重试")
time.sleep(10)
十、调试与优化
在实际开发中,爬虫脚本可能会遇到各种问题。通过调试和优化,可以提高脚本的稳定性和性能。可以使用Python的调试工具,如pdb
,或者集成开发环境(IDE)提供的调试功能,来排查问题。
import pdb
def fetch_data():
try:
response = requests.get('https://example.com')
response.raise_for_status()
return response.text
except requests.exceptions.RequestException as e:
pdb.set_trace() # 设置断点
return None
while True:
data = fetch_data()
if data:
print("数据爬取成功")
else:
print("数据爬取失败,等待重试")
time.sleep(10)
总结:通过使用循环、处理异常、设置合理的间隔时间、优化性能、使用代理、重试机制、数据存储与管理、日志记录、监控与报警,以及调试与优化,可以确保Python爬虫脚本能够稳定、持续地运行,实现长期的数据爬取任务。
相关问答FAQs:
如何确保我的Python爬虫程序不被网站封禁?
为了避免被目标网站封禁,可以采取以下措施:设置请求间隔,使用随机的User-Agent,采用代理IP,限制爬取频率。此外,遵守网站的robots.txt文件中的爬取规则,合理控制并发数也是有效的策略。
如何在Python中处理爬取过程中遇到的异常情况?
在爬取过程中,可能会遇到网络错误、数据解析异常等问题。可以使用try-except语句捕获这些异常,并在异常发生时进行重试或记录错误日志。此外,使用requests库的timeout参数设置请求超时时间也是一个好方法,以防止程序长时间挂起。
有没有推荐的Python库可以帮助我更高效地进行持续爬取?
可以使用Scrapy框架,它专为爬虫开发而设计,提供了强大的异步处理能力和数据存储功能。此外,Beautiful Soup和requests库也常被用于简单的爬虫任务,能够快速解析HTML并提取数据。这些工具都可以提高爬取的效率和可维护性。
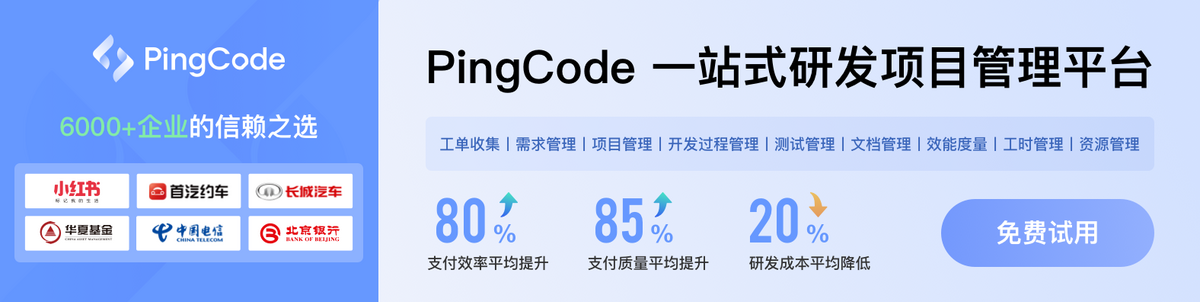