使用Python将图像转换为坐标的方法有很多,常见的包括:使用图像处理库如OpenCV进行边缘检测、通过颜色提取特定区域、利用机器学习进行特征点识别。接下来,我将详细描述如何使用OpenCV库进行边缘检测,将图像中的边缘转换为坐标点,并保存这些坐标供后续使用。
一、安装和导入必要的库
在进行图像处理前,我们需要安装并导入一些必要的库。主要使用的库有OpenCV(用于图像处理)、numpy(用于数值运算)和matplotlib(用于可视化)。
# 安装必要的库
!pip install opencv-python-headless numpy matplotlib
导入库
import cv2
import numpy as np
import matplotlib.pyplot as plt
二、读取图像并预处理
首先,读取图像并将其转换为灰度图像。灰度图像更便于进行边缘检测等操作。
# 读取图像
image = cv2.imread('path_to_image.jpg')
转换为灰度图像
gray = cv2.cvtColor(image, cv2.COLOR_BGR2GRAY)
显示灰度图像
plt.imshow(gray, cmap='gray')
plt.title('Gray Image')
plt.show()
三、应用边缘检测
使用OpenCV的Canny边缘检测器来检测图像中的边缘。Canny边缘检测器是一个多级边缘检测算法,能够有效地找到图像中的边缘。
# 使用Canny边缘检测器
edges = cv2.Canny(gray, threshold1=50, threshold2=150)
显示边缘检测结果
plt.imshow(edges, cmap='gray')
plt.title('Edge Image')
plt.show()
四、提取边缘坐标
使用numpy库提取检测到的边缘的坐标。我们可以通过numpy的where
函数找到所有非零像素的位置,这些位置即为边缘的坐标。
# 提取边缘的坐标
coordinates = np.column_stack(np.where(edges > 0))
打印一些坐标点
print(coordinates[:10])
五、保存坐标
将提取的坐标保存到文件中,以便后续使用。可以将坐标保存为CSV文件,方便在其他程序中读取和处理。
# 保存坐标到CSV文件
np.savetxt('coordinates.csv', coordinates, delimiter=',', fmt='%d')
读取并打印保存的坐标
saved_coordinates = np.loadtxt('coordinates.csv', delimiter=',', dtype=int)
print(saved_coordinates[:10])
六、可视化坐标
为了更直观地展示提取的坐标,可以使用matplotlib将这些坐标点绘制在图像上。
# 创建一个空白图像
height, width = edges.shape
blank_image = np.zeros((height, width), dtype=np.uint8)
将坐标点绘制在图像上
for coord in coordinates:
blank_image[coord[0], coord[1]] = 255
显示坐标点图像
plt.imshow(blank_image, cmap='gray')
plt.title('Coordinates Image')
plt.show()
通过上述步骤,我们可以使用Python将图像转换为坐标。该方法主要利用OpenCV进行图像处理,提取图像中的边缘,并将边缘的坐标保存下来。这样,我们就可以在后续的处理或分析中使用这些坐标。
七、扩展应用
除了基本的边缘检测和坐标提取,还有许多其他应用和方法可以进一步扩展这一过程。
1、颜色提取
如果图像中有特定颜色的区域,我们可以通过颜色提取来获取这些区域的坐标。以下是一个简单的示例,提取图像中红色区域的坐标:
# 定义红色的HSV范围
lower_red = np.array([0, 120, 70])
upper_red = np.array([10, 255, 255])
转换图像为HSV格式
hsv_image = cv2.cvtColor(image, cv2.COLOR_BGR2HSV)
提取红色区域
mask = cv2.inRange(hsv_image, lower_red, upper_red)
提取红色区域的坐标
red_coordinates = np.column_stack(np.where(mask > 0))
显示红色区域提取结果
plt.imshow(mask, cmap='gray')
plt.title('Red Mask')
plt.show()
打印一些红色区域的坐标
print(red_coordinates[:10])
2、特征点检测
使用特征点检测算法(如SIFT、SURF、ORB等)来提取图像中的关键点,并获取这些关键点的坐标。以下是使用ORB特征点检测器的示例:
# 创建ORB特征点检测器
orb = cv2.ORB_create()
检测关键点
keypoints, descriptors = orb.detectAndCompute(gray, None)
绘制关键点
keypoints_image = cv2.drawKeypoints(image, keypoints, None, color=(0, 255, 0))
显示关键点图像
plt.imshow(cv2.cvtColor(keypoints_image, cv2.COLOR_BGR2RGB))
plt.title('Keypoints Image')
plt.show()
提取关键点坐标
keypoints_coordinates = np.array([kp.pt for kp in keypoints], dtype=np.int)
打印一些关键点坐标
print(keypoints_coordinates[:10])
3、机器学习方法
利用机器学习方法,如卷积神经网络(CNN),可以进行更复杂和精确的图像处理和特征提取。通过训练一个模型,可以识别图像中的特定对象,并提取其坐标。以下是一个简单的示例,使用预训练的YOLO模型进行对象检测:
# 下载YOLO模型权重和配置文件
!wget -O yolov3.weights https://pjreddie.com/media/files/yolov3.weights
!wget -O yolov3.cfg https://raw.githubusercontent.com/pjreddie/darknet/master/cfg/yolov3.cfg
!wget -O coco.names https://raw.githubusercontent.com/pjreddie/darknet/master/data/coco.names
加载YOLO模型
net = cv2.dnn.readNet('yolov3.weights', 'yolov3.cfg')
加载类别名称
with open('coco.names', 'r') as f:
classes = f.read().strip().split('\n')
准备图像输入
blob = cv2.dnn.blobFromImage(image, 1/255.0, (416, 416), swapRB=True, crop=False)
net.setInput(blob)
进行前向传播,获取检测结果
layer_names = net.getLayerNames()
output_layers = [layer_names[i[0] - 1] for i in net.getUnconnectedOutLayers()]
detections = net.forward(output_layers)
解析检测结果
height, width = image.shape[:2]
boxes, confidences, class_ids = [], [], []
for output in detections:
for detection in output:
scores = detection[5:]
class_id = np.argmax(scores)
confidence = scores[class_id]
if confidence > 0.5:
box = detection[0:4] * np.array([width, height, width, height])
(centerX, centerY, w, h) = box.astype("int")
x = int(centerX - (w / 2))
y = int(centerY - (h / 2))
boxes.append([x, y, int(w), int(h)])
confidences.append(float(confidence))
class_ids.append(class_id)
应用非极大值抑制,消除冗余的重叠框
indices = cv2.dnn.NMSBoxes(boxes, confidences, 0.5, 0.4)
绘制检测框
for i in indices.flatten():
x, y, w, h = boxes[i]
label = str(classes[class_ids[i]])
cv2.rectangle(image, (x, y), (x+w, y+h), (0, 255, 0), 2)
cv2.putText(image, label, (x, y-10), cv2.FONT_HERSHEY_SIMPLEX, 0.5, (0, 255, 0), 2)
显示检测结果
plt.imshow(cv2.cvtColor(image, cv2.COLOR_BGR2RGB))
plt.title('YOLO Detection')
plt.show()
通过这些示例,可以看到如何使用不同的方法将图像转换为坐标。这些方法包括基本的图像处理、颜色提取、特征点检测和机器学习方法。根据具体的应用场景和需求,可以选择合适的方法来实现图像到坐标的转换。
相关问答FAQs:
如何使用Python提取图像中的坐标数据?
通过一些图像处理库,如OpenCV或Pillow,可以轻松提取图像中的坐标。具体步骤包括加载图像、转换为灰度图、应用边缘检测,然后使用轮廓检测功能来识别并提取坐标信息。可以根据需要进一步处理这些坐标。
Python有哪些库可以用于图像到坐标的转换?
Python中有多种库可以帮助实现图像转坐标的功能。常用的包括OpenCV、Pillow、Matplotlib和Scikit-image等。OpenCV是最常用的库之一,提供了丰富的图像处理工具,可以方便地进行坐标提取和分析。
如何处理提取的坐标以便于后续分析?
提取到的坐标数据通常以列表或数组的形式存在。可以使用Pandas库将这些坐标数据转换为DataFrame,便于后续的分析和可视化。通过数据清洗和转换,能够更好地利用这些坐标数据进行图形绘制或其他数据分析任务。
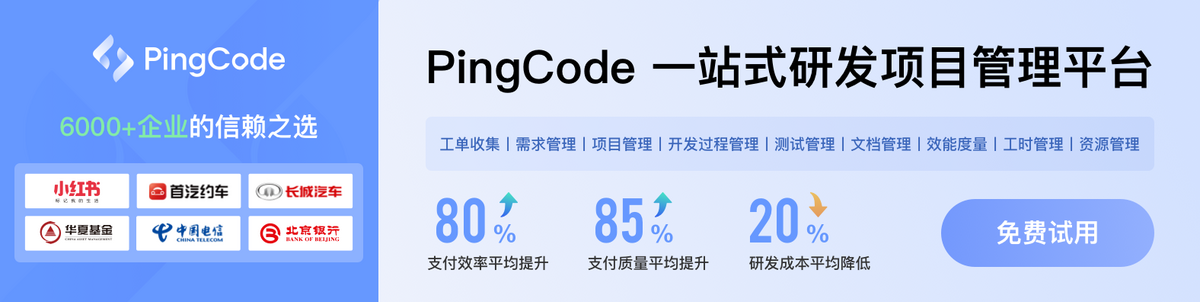