Python浮点类型的数如何求分位数可以通过使用Python标准库中的numpy
库来实现。主要方法包括:使用numpy.percentile()
函数、使用numpy.quantile()
函数。下面将详细介绍如何使用这些方法来求浮点类型数的分位数,并提供示例代码。
一、使用numpy.percentile()
函数
numpy.percentile()
函数可以用于计算数组中指定百分位的值。该函数的第一个参数是数组,第二个参数是百分位数。以下是详细的步骤和示例代码:
import numpy as np
示例数据
data = np.array([1.5, 2.3, 3.7, 4.1, 5.0])
计算第25百分位数(即第一四分位数)
percentile_25 = np.percentile(data, 25)
print(f"第25百分位数:{percentile_25}")
计算第50百分位数(即中位数)
percentile_50 = np.percentile(data, 50)
print(f"第50百分位数:{percentile_50}")
计算第75百分位数(即第三四分位数)
percentile_75 = np.percentile(data, 75)
print(f"第75百分位数:{percentile_75}")
在上面的代码中,我们首先导入了numpy
库,然后定义了一个包含浮点类型数的数组data
。接着,我们使用numpy.percentile()
函数分别计算了第25、第50和第75百分位数,并输出了结果。
二、使用numpy.quantile()
函数
numpy.quantile()
函数与numpy.percentile()
函数类似,但它接受的第二个参数是一个分位点(在0到1之间),而不是百分位数。以下是详细的步骤和示例代码:
import numpy as np
示例数据
data = np.array([1.5, 2.3, 3.7, 4.1, 5.0])
计算第25百分位数(即第一四分位数)
quantile_25 = np.quantile(data, 0.25)
print(f"第25百分位数:{quantile_25}")
计算第50百分位数(即中位数)
quantile_50 = np.quantile(data, 0.50)
print(f"第50百分位数:{quantile_50}")
计算第75百分位数(即第三四分位数)
quantile_75 = np.quantile(data, 0.75)
print(f"第75百分位数:{quantile_75}")
在上面的代码中,我们使用numpy.quantile()
函数分别计算了第25、第50和第75分位点的值,并输出了结果。与numpy.percentile()
函数类似,我们首先导入了numpy
库,然后定义了一个包含浮点类型数的数组data
。
三、自定义分位数函数
有时候我们可能希望自定义分位数计算函数,以便更好地满足特定需求。以下是一个示例代码,演示了如何实现自定义分位数函数:
def calculate_percentile(data, percentile):
"""
计算数据中指定百分位数的值
:param data: 数据列表
:param percentile: 百分位数(0到100之间的数值)
:return: 指定百分位数的值
"""
data_sorted = sorted(data)
index = (len(data_sorted) - 1) * percentile / 100
lower = int(np.floor(index))
upper = int(np.ceil(index))
if lower == upper:
return data_sorted[lower]
else:
return data_sorted[lower] * (upper - index) + data_sorted[upper] * (index - lower)
示例数据
data = [1.5, 2.3, 3.7, 4.1, 5.0]
计算第25百分位数(即第一四分位数)
percentile_25 = calculate_percentile(data, 25)
print(f"第25百分位数:{percentile_25}")
计算第50百分位数(即中位数)
percentile_50 = calculate_percentile(data, 50)
print(f"第50百分位数:{percentile_50}")
计算第75百分位数(即第三四分位数)
percentile_75 = calculate_percentile(data, 75)
print(f"第75百分位数:{percentile_75}")
在上面的代码中,我们定义了一个名为calculate_percentile()
的函数,该函数接受两个参数:数据列表data
和百分位数percentile
。函数首先对数据进行排序,然后根据百分位数计算索引位置,并返回相应的值。
四、使用scipy
库
除了numpy
库外,我们还可以使用scipy
库来计算分位数。scipy
库提供了更丰富的统计功能,以下是使用scipy
库计算分位数的示例代码:
import numpy as np
from scipy import stats
示例数据
data = np.array([1.5, 2.3, 3.7, 4.1, 5.0])
计算第25百分位数(即第一四分位数)
percentile_25 = stats.scoreatpercentile(data, 25)
print(f"第25百分位数:{percentile_25}")
计算第50百分位数(即中位数)
percentile_50 = stats.scoreatpercentile(data, 50)
print(f"第50百分位数:{percentile_50}")
计算第75百分位数(即第三四分位数)
percentile_75 = stats.scoreatpercentile(data, 75)
print(f"第75百分位数:{percentile_75}")
在上面的代码中,我们首先导入了numpy
库和scipy
库中的stats
模块,然后定义了一个包含浮点类型数的数组data
。接着,我们使用stats.scoreatpercentile()
函数分别计算了第25、第50和第75百分位数,并输出了结果。
五、处理大数据集
在处理大数据集时,我们可能需要优化分位数计算的方法,以提高计算效率。以下是一个示例代码,演示了如何使用numpy
库中的partition()
函数来优化分位数计算:
import numpy as np
def calculate_large_dataset_percentile(data, percentile):
"""
计算大数据集中指定百分位数的值
:param data: 数据列表
:param percentile: 百分位数(0到100之间的数值)
:return: 指定百分位数的值
"""
k = int(len(data) * percentile / 100)
partitioned_data = np.partition(data, k)
return partitioned_data[k]
示例数据
data = np.random.rand(1000000) # 生成100万个随机数
计算第25百分位数(即第一四分位数)
percentile_25 = calculate_large_dataset_percentile(data, 25)
print(f"第25百分位数:{percentile_25}")
计算第50百分位数(即中位数)
percentile_50 = calculate_large_dataset_percentile(data, 50)
print(f"第50百分位数:{percentile_50}")
计算第75百分位数(即第三四分位数)
percentile_75 = calculate_large_dataset_percentile(data, 75)
print(f"第75百分位数:{percentile_75}")
在上面的代码中,我们定义了一个名为calculate_large_dataset_percentile()
的函数,该函数接受两个参数:数据列表data
和百分位数percentile
。函数使用numpy.partition()
函数对数据进行分区,并返回指定百分位数的值。通过这种方法,我们可以有效地处理大数据集,并提高分位数计算的效率。
六、总结
在本文中,我们详细介绍了如何在Python中计算浮点类型数的分位数,并提供了多种方法和示例代码。主要方法包括使用numpy.percentile()
函数、使用numpy.quantile()
函数、自定义分位数函数、使用scipy
库以及优化大数据集分位数计算的方法。
通过这些方法,我们可以灵活地计算各种分位数,以满足不同的需求和应用场景。无论是处理小数据集还是大数据集,我们都可以选择合适的方法来提高计算效率和准确性。
总之,计算分位数是数据分析中的一个重要步骤,它可以帮助我们更好地了解数据的分布和特征。希望本文提供的方法和示例代码能够对您有所帮助,并在实际应用中发挥作用。
相关问答FAQs:
如何在Python中计算浮点数的分位数?
在Python中,可以使用NumPy库的numpy.percentile()
函数来计算浮点数的分位数。首先,需要将浮点数放入一个列表或数组中,然后指定所需的百分位数。例如,要计算25%的分位数,可以这样使用:numpy.percentile(data, 25)
,其中data
是你的浮点数数据。
有没有其他库可以计算浮点数的分位数?
除了NumPy,Pandas库也提供了方便的分位数计算功能。通过使用Pandas的DataFrame或Series对象,你可以调用quantile()
方法来获取分位数。例如,df['column_name'].quantile(0.25)
可以获取指定列的25%分位数。
在计算分位数时,如何处理缺失值?
在Python中,处理缺失值时可以使用NumPy和Pandas的内置功能。NumPy的numpy.nanpercentile()
函数会自动忽略NaN值,而Pandas的quantile()
方法默认也会跳过缺失值。如果你的数据中包含缺失值,使用这些函数可以确保你的分位数计算是准确的。
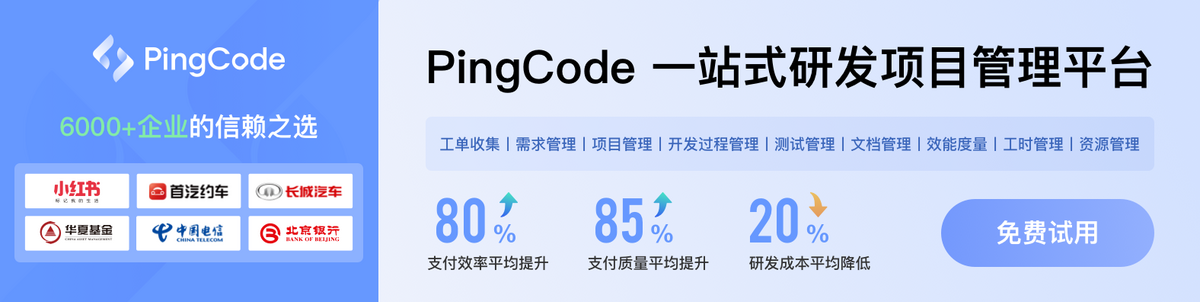