在Python中查看GPU的使用情况,可以通过以下几种方法:使用NVIDIA的nvidia-smi工具、使用Python的GPUtil库、使用TensorFlow或PyTorch等深度学习框架的内置方法。 其中,nvidia-smi工具是最直接和常用的方法,因为它是由NVIDIA官方提供的,可以准确地显示GPU的详细信息。我们接下来将详细讨论如何使用这些方法来查看和监控GPU的使用情况。
一、NVIDIA的nvidia-smi工具
什么是nvidia-smi工具?
nvidia-smi(NVIDIA System Management Interface)是NVIDIA提供的一款命令行工具,用于管理和监控GPU设备。它可以显示GPU的温度、使用率、显存占用等详细信息。
如何使用nvidia-smi工具?
-
安装NVIDIA驱动:首先,确保你已经安装了NVIDIA的显卡驱动,因为nvidia-smi工具是驱动的一部分。
-
运行nvidia-smi命令:在命令行中输入
nvidia-smi
,你会看到如下输出:+-----------------------------------------------------------------------------+
| NVIDIA-SMI 460.32.03 Driver Version: 460.32.03 CUDA Version: 11.2 |
|-------------------------------+----------------------+----------------------+
| GPU Name Persistence-M| Bus-Id Disp.A | Volatile Uncorr. ECC |
| Fan Temp Perf Pwr:Usage/Cap| Memory-Usage | GPU-Util Compute M. |
| | | MIG M. |
|===============================+======================+======================|
| 0 Tesla K80 On | 00000000:00:1E.0 Off | 0 |
| N/A 54C P8 30W / 149W | 777MiB / 11441MiB | 0% Default |
+-------------------------------+----------------------+----------------------+
-
解释输出信息:
- GPU Name:GPU的型号名称。
- Fan:风扇的转速情况。
- Temp:GPU的温度。
- Perf:性能状态。
- Pwr:Usage/Cap:当前功耗和最大功耗。
- Memory-Usage:显存使用情况。
- GPU-Util:GPU使用率。
通过nvidia-smi命令,你可以实时监控GPU的各项指标,方便进行调试和优化。
二、使用Python的GPUtil库
什么是GPUtil库?
GPUtil是一个Python库,用于获取NVIDIA GPU的使用情况。它可以很方便地在Python脚本中嵌入GPU监控功能。
如何安装和使用GPUtil库?
-
安装GPUtil:你可以通过pip命令安装GPUtil库:
pip install gputil
-
使用GPUtil获取GPU信息:
import GPUtil
获取所有可用的GPU
gpus = GPUtil.getGPUs()
for gpu in gpus:
print(f"GPU ID: {gpu.id}")
print(f"GPU Name: {gpu.name}")
print(f"GPU Load: {gpu.load * 100}%")
print(f"GPU Free Memory: {gpu.memoryFree}MB")
print(f"GPU Used Memory: {gpu.memoryUsed}MB")
print(f"GPU Total Memory: {gpu.memoryTotal}MB")
print(f"GPU Temperature: {gpu.temperature} °C")
-
解释GPUtil的输出信息:
- GPU ID:GPU的ID编号。
- GPU Name:GPU的型号名称。
- GPU Load:GPU的使用率。
- GPU Free Memory:GPU空闲显存。
- GPU Used Memory:GPU已用显存。
- GPU Total Memory:GPU总显存。
- GPU Temperature:GPU的温度。
GPUtil库提供了一个简洁的接口,使得在Python脚本中监控GPU变得非常简单。
三、使用TensorFlow查看GPU使用情况
TensorFlow中的GPU监控
TensorFlow是一个广泛使用的深度学习框架,它提供了内置的方法来查看和管理GPU的使用情况。
如何查看TensorFlow中的GPU信息?
-
安装TensorFlow:确保你已经安装了TensorFlow,可以通过pip命令安装:
pip install tensorflow
-
查看可用的GPU:
import tensorflow as tf
列出所有可用的GPU
gpus = tf.config.experimental.list_physical_devices('GPU')
for gpu in gpus:
print(gpu)
-
监控TensorFlow中的GPU使用情况:
import tensorflow as tf
创建一个简单的TensorFlow模型
model = tf.keras.models.Sequential([
tf.keras.layers.Dense(64, activation='relu', input_shape=(1000,)),
tf.keras.layers.Dense(64, activation='relu'),
tf.keras.layers.Dense(10, activation='softmax')
])
model.compile(optimizer='adam', loss='categorical_crossentropy')
生成一些随机数据
import numpy as np
x_train = np.random.random((1000, 1000))
y_train = np.random.random((1000, 10))
训练模型
model.fit(x_train, y_train, epochs=10)
查看GPU使用情况
from tensorflow.python.client import device_lib
print(device_lib.list_local_devices())
通过上述代码,你可以查看TensorFlow在训练过程中使用的GPU设备。
四、使用PyTorch查看GPU使用情况
PyTorch中的GPU监控
PyTorch是另一个广泛使用的深度学习框架,它同样提供了方便的方法来查看和管理GPU的使用情况。
如何查看PyTorch中的GPU信息?
-
安装PyTorch:确保你已经安装了PyTorch,可以通过pip命令安装:
pip install torch
-
查看可用的GPU:
import torch
if torch.cuda.is_available():
device = torch.device("cuda")
print("GPU is available")
print(f"GPU Name: {torch.cuda.get_device_name(0)}")
print(f"Total Memory: {torch.cuda.get_device_properties(0).total_memory} bytes")
print(f"Memory Allocated: {torch.cuda.memory_allocated(0)} bytes")
print(f"Memory Cached: {torch.cuda.memory_reserved(0)} bytes")
else:
print("GPU is not available")
-
监控PyTorch中的GPU使用情况:
import torch
创建一个简单的PyTorch模型
model = torch.nn.Sequential(
torch.nn.Linear(1000, 64),
torch.nn.ReLU(),
torch.nn.Linear(64, 64),
torch.nn.ReLU(),
torch.nn.Linear(64, 10),
torch.nn.Softmax(dim=1)
)
model.to(device)
生成一些随机数据
x_train = torch.randn(1000, 1000).to(device)
y_train = torch.randn(1000, 10).to(device)
定义损失函数和优化器
criterion = torch.nn.CrossEntropyLoss()
optimizer = torch.optim.Adam(model.parameters())
训练模型
for epoch in range(10):
optimizer.zero_grad()
output = model(x_train)
loss = criterion(output, y_train)
loss.backward()
optimizer.step()
查看GPU使用情况
print(f"Memory Allocated: {torch.cuda.memory_allocated(0)} bytes")
print(f"Memory Cached: {torch.cuda.memory_reserved(0)} bytes")
通过上述代码,你可以查看PyTorch在训练过程中使用的GPU设备。
五、总结
通过使用nvidia-smi工具、GPUtil库、TensorFlow和PyTorch的内置方法,你可以非常方便地在Python中查看和监控GPU的使用情况。每种方法都有其独特的优势和适用场景,选择合适的方法可以帮助你更好地优化和调试你的程序。nvidia-smi工具最为直接和详细,GPUtil库在Python脚本中使用非常方便,而TensorFlow和PyTorch提供了与深度学习框架紧密结合的解决方案。
相关问答FAQs:
如何确认我的Python环境是否能够使用GPU?
要确认您的Python环境是否能够使用GPU,您可以尝试安装并导入适用于GPU计算的库,如TensorFlow或PyTorch。在安装完成后,运行以下代码来检查GPU的可用性:
import tensorflow as tf
print("Num GPUs Available: ", len(tf.config.list_physical_devices('GPU')))
如果您使用的是PyTorch,可以执行:
import torch
print("Is GPU available: ", torch.cuda.is_available())
这些代码将告诉您当前环境是否检测到GPU。
我可以通过哪些工具监控GPU的实时使用情况?
可以使用NVIDIA的nvidia-smi工具来监控GPU的实时使用情况。只需在命令行中输入nvidia-smi
,即可查看GPU的使用率、内存占用、温度等信息。此外,一些图形化工具如GPU-Z和MSI Afterburner也能够提供详细的监控功能。
在Python中如何优化我的代码以利用GPU?
要优化您的Python代码以更好地利用GPU,您可以考虑以下几个方面:
- 使用GPU加速的库,如CuPy、TensorFlow或PyTorch,这些库提供了GPU支持的高效运算。
- 尽量减少数据传输的频率,因为数据在CPU和GPU之间传输会造成延迟。将数据尽可能多地保留在GPU内存中。
- 对于大规模的矩阵运算,使用批处理的方式来提高计算效率。
- 确保代码中没有不必要的同步操作,以减少GPU的空闲时间。
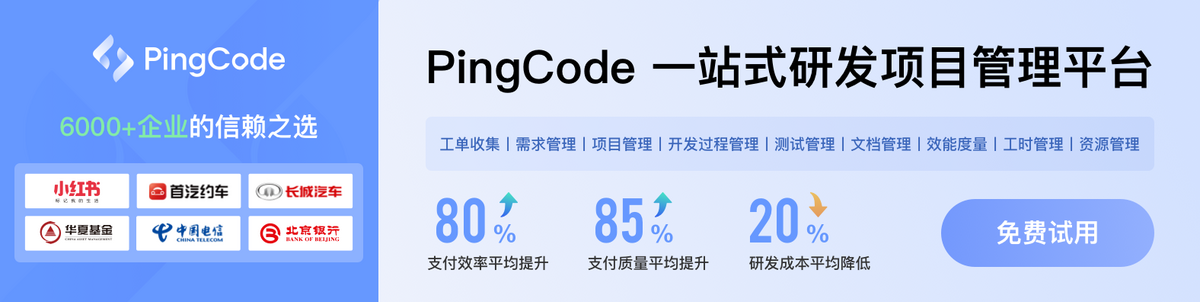