如何用Python自动处理文章的核心观点有:使用自然语言处理库、文本预处理、关键词提取、情感分析、自动摘要生成。 其中,文本预处理是自动处理文章的基础步骤,涉及到去除停用词、标点符号,进行分词和词性标注,确保后续处理的准确性和有效性。文本预处理的良好实现能够极大地提高整个自动处理文章过程的质量和效率。
一、使用自然语言处理库
自然语言处理(NLP)库是Python自动处理文章的基础工具,这些库提供了丰富的功能和方法来处理和分析文本数据。常用的自然语言处理库包括NLTK、spaCy、TextBlob、Gensim等。
1、NLTK
NLTK(Natural Language Toolkit)是一个功能强大的NLP库,提供了丰富的工具和资源来处理文本数据。它包含了大量的文本处理和分析功能,如分词、词性标注、命名实体识别、语法解析等。
import nltk
from nltk.tokenize import word_tokenize
from nltk.corpus import stopwords
下载必要的数据包
nltk.download('punkt')
nltk.download('stopwords')
示例文本
text = "Python is a powerful programming language."
分词
tokens = word_tokenize(text)
去除停用词
stop_words = set(stopwords.words('english'))
filtered_tokens = [word for word in tokens if word.lower() not in stop_words]
print(filtered_tokens)
2、spaCy
spaCy是一个工业级的NLP库,具有高效和快速的特点,适用于大规模的文本数据处理。它提供了分词、词性标注、依存解析、命名实体识别等功能。
import spacy
加载英语模型
nlp = spacy.load('en_core_web_sm')
示例文本
text = "Python is a powerful programming language."
处理文本
doc = nlp(text)
分词和词性标注
for token in doc:
print(token.text, token.pos_)
二、文本预处理
文本预处理是自动处理文章的重要步骤,确保文本数据的质量和一致性,为后续的分析和处理奠定基础。常见的文本预处理步骤包括去除停用词、标点符号,进行分词和词性标注。
1、去除停用词和标点符号
停用词和标点符号通常在文本分析中没有实际意义,需要在预处理阶段去除。
import string
示例文本
text = "Python is a powerful programming language."
去除标点符号
text = text.translate(str.maketrans('', '', string.punctuation))
分词
tokens = word_tokenize(text)
去除停用词
filtered_tokens = [word for word in tokens if word.lower() not in stop_words]
print(filtered_tokens)
2、分词和词性标注
分词和词性标注是文本预处理的重要步骤,有助于后续的文本分析和处理。
# 使用spaCy进行分词和词性标注
doc = nlp(text)
分词和词性标注
for token in doc:
print(token.text, token.pos_)
三、关键词提取
关键词提取是自动处理文章的重要步骤,有助于识别和提取文本中的重要信息。常用的关键词提取方法包括TF-IDF、RAKE、TextRank等。
1、TF-IDF
TF-IDF(Term Frequency-Inverse Document Frequency)是一种常用的关键词提取方法,通过计算词语在文档中的频率和在整个语料库中的逆频率来衡量词语的重要性。
from sklearn.feature_extraction.text import TfidfVectorizer
示例文本
documents = ["Python is a powerful programming language.",
"Machine learning is a fascinating field."]
计算TF-IDF
vectorizer = TfidfVectorizer()
tfidf_matrix = vectorizer.fit_transform(documents)
输出TF-IDF矩阵
print(tfidf_matrix.toarray())
2、RAKE
RAKE(Rapid Automatic Keyword Extraction)是一种简单高效的关键词提取算法,通过分析词语的共现关系来提取关键词。
from rake_nltk import Rake
示例文本
text = "Python is a powerful programming language."
使用RAKE提取关键词
rake = Rake()
rake.extract_keywords_from_text(text)
keywords = rake.get_ranked_phrases()
print(keywords)
四、情感分析
情感分析是自动处理文章的重要应用,通过分析文本中的情感倾向来识别和分类文本的情感。常用的情感分析库包括TextBlob、VADER等。
1、TextBlob
TextBlob是一个简单易用的NLP库,提供了文本处理和情感分析功能。
from textblob import TextBlob
示例文本
text = "Python is a powerful programming language."
情感分析
blob = TextBlob(text)
sentiment = blob.sentiment
print(sentiment)
2、VADER
VADER(Valence Aware Dictionary and sEntiment Reasoner)是一种专为社交媒体文本设计的情感分析工具,具有高效和准确的特点。
from vaderSentiment.vaderSentiment import SentimentIntensityAnalyzer
示例文本
text = "Python is a powerful programming language."
使用VADER进行情感分析
analyzer = SentimentIntensityAnalyzer()
sentiment = analyzer.polarity_scores(text)
print(sentiment)
五、自动摘要生成
自动摘要生成是自动处理文章的高级应用,通过提取文本中的重要信息来生成简洁的摘要。常用的自动摘要生成方法包括提取式摘要和生成式摘要。
1、提取式摘要
提取式摘要通过提取原文中的重要句子来生成摘要,常用的方法包括TextRank、LSA等。
from gensim.summarization import summarize
示例文本
text = "Python is a powerful programming language. It is widely used in various fields such as data science, machine learning, web development, etc. Python has a simple syntax and a large number of libraries, making it easy to learn and use."
使用Gensim生成摘要
summary = summarize(text)
print(summary)
2、生成式摘要
生成式摘要通过生成新的句子来生成摘要,常用的方法包括序列到序列(Seq2Seq)模型、Transformer等。
from transformers import pipeline
示例文本
text = "Python is a powerful programming language. It is widely used in various fields such as data science, machine learning, web development, etc. Python has a simple syntax and a large number of libraries, making it easy to learn and use."
使用Transformer生成摘要
summarizer = pipeline("summarization")
summary = summarizer(text, max_length=50, min_length=25, do_sample=False)
print(summary)
六、文本分类
文本分类是自动处理文章的重要应用,通过将文本分配到预定义的类别中来实现分类。常用的文本分类方法包括朴素贝叶斯、支持向量机、深度学习等。
1、朴素贝叶斯
朴素贝叶斯是一种简单高效的文本分类方法,适用于小规模文本数据分类。
from sklearn.feature_extraction.text import CountVectorizer
from sklearn.naive_bayes import MultinomialNB
示例文本
documents = ["Python is a powerful programming language.",
"Machine learning is a fascinating field."]
labels = ["programming", "machine_learning"]
特征提取
vectorizer = CountVectorizer()
X = vectorizer.fit_transform(documents)
训练朴素贝叶斯分类器
classifier = MultinomialNB()
classifier.fit(X, labels)
测试分类器
test_document = ["Python is widely used in data science."]
test_X = vectorizer.transform(test_document)
predicted_label = classifier.predict(test_X)
print(predicted_label)
2、深度学习
深度学习方法,如卷积神经网络(CNN)、循环神经网络(RNN)、Transformer等,适用于大规模文本数据分类,具有较高的分类准确率。
from keras.preprocessing.text import Tokenizer
from keras.preprocessing.sequence import pad_sequences
from keras.models import Sequential
from keras.layers import Embedding, LSTM, Dense
示例文本
documents = ["Python is a powerful programming language.",
"Machine learning is a fascinating field."]
labels = [1, 0]
文本预处理
tokenizer = Tokenizer(num_words=5000)
tokenizer.fit_on_texts(documents)
X = tokenizer.texts_to_sequences(documents)
X = pad_sequences(X, maxlen=100)
构建LSTM模型
model = Sequential()
model.add(Embedding(input_dim=5000, output_dim=128, input_length=100))
model.add(LSTM(units=128))
model.add(Dense(units=1, activation='sigmoid'))
编译模型
model.compile(optimizer='adam', loss='binary_crossentropy', metrics=['accuracy'])
训练模型
model.fit(X, labels, epochs=10, batch_size=32)
测试模型
test_document = ["Python is widely used in data science."]
test_X = tokenizer.texts_to_sequences(test_document)
test_X = pad_sequences(test_X, maxlen=100)
predicted_label = model.predict(test_X)
print(predicted_label)
七、文本生成
文本生成是自动处理文章的高级应用,通过生成新的文本内容来实现自动化写作。常用的文本生成方法包括序列到序列(Seq2Seq)模型、GPT等。
1、序列到序列(Seq2Seq)模型
序列到序列模型通过编码器-解码器架构来实现文本生成,适用于机器翻译、对话生成等任务。
from keras.models import Model
from keras.layers import Input, LSTM, Dense
示例数据
input_texts = ["Python is a powerful programming language."]
target_texts = ["Python 是一种强大的编程语言。"]
文本预处理
input_tokenizer = Tokenizer(num_words=5000)
target_tokenizer = Tokenizer(num_words=5000)
input_tokenizer.fit_on_texts(input_texts)
target_tokenizer.fit_on_texts(target_texts)
input_sequences = input_tokenizer.texts_to_sequences(input_texts)
target_sequences = target_tokenizer.texts_to_sequences(target_texts)
input_sequences = pad_sequences(input_sequences, maxlen=100)
target_sequences = pad_sequences(target_sequences, maxlen=100)
构建Seq2Seq模型
encoder_inputs = Input(shape=(None,))
encoder_embedding = Embedding(input_dim=5000, output_dim=128)(encoder_inputs)
encoder_lstm = LSTM(units=128, return_state=True)
encoder_outputs, state_h, state_c = encoder_lstm(encoder_embedding)
encoder_states = [state_h, state_c]
decoder_inputs = Input(shape=(None,))
decoder_embedding = Embedding(input_dim=5000, output_dim=128)(decoder_inputs)
decoder_lstm = LSTM(units=128, return_sequences=True, return_state=True)
decoder_outputs, _, _ = decoder_lstm(decoder_embedding, initial_state=encoder_states)
decoder_dense = Dense(units=5000, activation='softmax')
decoder_outputs = decoder_dense(decoder_outputs)
model = Model([encoder_inputs, decoder_inputs], decoder_outputs)
编译模型
model.compile(optimizer='adam', loss='sparse_categorical_crossentropy', metrics=['accuracy'])
训练模型
model.fit([input_sequences, target_sequences], target_sequences, epochs=10, batch_size=32)
测试模型
test_input_text = ["Python is a powerful programming language."]
test_input_sequences = input_tokenizer.texts_to_sequences(test_input_text)
test_input_sequences = pad_sequences(test_input_sequences, maxlen=100)
predicted_sequence = model.predict([test_input_sequences, target_sequences])
print(predicted_sequence)
2、GPT
GPT(Generative Pre-trained Transformer)是一种基于Transformer的文本生成模型,具有强大的文本生成能力,适用于自动化写作、对话生成等任务。
from transformers import GPT2LMHeadModel, GPT2Tokenizer
加载GPT模型和分词器
model_name = 'gpt2'
model = GPT2LMHeadModel.from_pretrained(model_name)
tokenizer = GPT2Tokenizer.from_pretrained(model_name)
示例文本
input_text = "Python is a powerful programming language."
文本生成
input_ids = tokenizer.encode(input_text, return_tensors='pt')
output = model.generate(input_ids, max_length=50)
generated_text = tokenizer.decode(output[0], skip_special_tokens=True)
print(generated_text)
八、文本相似度计算
文本相似度计算是自动处理文章的常见任务,通过计算文本之间的相似度来实现文本匹配、推荐等功能。常用的文本相似度计算方法包括余弦相似度、Jaccard相似度、Word2Vec等。
1、余弦相似度
余弦相似度通过计算两个文本向量的余弦值来衡量文本之间的相似度。
from sklearn.feature_extraction.text import TfidfVectorizer
from sklearn.metrics.pairwise import cosine_similarity
示例文本
documents = ["Python is a powerful programming language.",
"Machine learning is a fascinating field."]
计算TF-IDF
vectorizer = TfidfVectorizer()
tfidf_matrix = vectorizer.fit_transform(documents)
计算余弦相似度
cosine_sim = cosine_similarity(tfidf_matrix[0:1], tfidf_matrix)
print(cosine_sim)
2、Word2Vec
Word2Vec通过将词语映射到向量空间来计算文本之间的相似度,适用于大规模文本数据相似度计算。
from gensim.models import Word2Vec
from nltk.tokenize import word_tokenize
示例文本
documents = ["Python is a powerful programming language.",
"Machine learning is a fascinating field."]
分词
tokenized_documents = [word_tokenize(doc.lower()) for doc in documents]
训练Word2Vec模型
model = Word2Vec(sentences=tokenized_documents, vector_size=100, window=5, min_count=1, workers=4)
计算词语相似度
similarity = model.wv.similarity('python', 'machine')
print(similarity)
九、命名实体识别
命名实体识别(NER)是自动处理文章的重要任务,通过识别和分类文本中的实体来实现信息抽取。常用的NER方法包括规则基方法、统计学习方法、深度学习方法等。
1、spaCy NER
spaCy提供了强大的NER功能,能够识别文本中的人名、地名、组织等实体。
import spacy
加载英语模型
nlp = spacy.load('en_core_web_sm')
示例文本
text = "Python is developed by Guido van Rossum."
命名实体识别
doc = nlp(text)
for ent in doc.ents:
print(ent.text, ent.label_)
2、深度学习 NER
深度学习方法通过训练神经网络模型来实现NER,具有较高的识别准确率。
from transformers import BertTokenizer, BertForTokenClassification
from transformers import pipeline
加载BERT模型和分词器
model_name = 'dbmdz/bert-large-cased-finetuned-conll03-english'
tokenizer = BertTokenizer.from_pretrained(model_name)
model = BertForTokenClassification.from_pretrained(model_name)
示例文本
text = "Python is developed by Guido van Rossum."
命名实体识别
ner_pipeline = pipeline('ner', model=model, tokenizer=tokenizer)
entities = ner_pipeline(text)
print(entities)
十、主题模型
主题模型是自动处理文章的重要方法,通过识别和提取文本中的主题来实现文本分类、信息检索等任务。常用的主题模型方法包括LDA、NMF等。
1、LDA
LDA(Latent Dirichlet Allocation)是一种常用的主题模型方法,通过假设文本由多个主题混合生成来提取文本中的主题。
from sklearn.feature_extraction.text import CountVectorizer
from sklearn.decomposition import LatentDirichletAllocation
示例文本
documents = ["Python is a powerful programming language.",
"Machine learning is a fascinating field."]
特征提取
vectorizer = CountVectorizer()
X = vectorizer.fit_transform(documents)
训练LDA模型
lda = LatentDirichletAllocation(n_components=2, random_state=0)
lda.fit(X)
输出主题词
for index, topic in enumerate(lda.components_):
print(f"Topic {index+1}:")
print([vectorizer
相关问答FAQs:
如何用Python处理不同格式的文章?
Python提供了丰富的库和工具,可以处理多种格式的文章,例如文本文件、Markdown、HTML等。使用pandas
可以轻松读取CSV或Excel文件,BeautifulSoup
适合解析HTML,markdown
库可以将Markdown转换为HTML格式。根据文章格式的不同,选择合适的库和方法,可以高效地处理和分析文章内容。
Python中有哪些库可以帮助我自动化文章处理?
在Python中,有多个库可以帮助自动化文章处理。nltk
和spaCy
是处理自然语言的重要工具,能够进行分词、词性标注等操作。gensim
适合进行主题建模,而PyPDF2
和pdfminer
则用于处理PDF文件。此外,docx
库可以处理Word文档。这些库各具特色,能够满足不同的需求。
如何用Python自动生成文章摘要?
生成文章摘要可以通过自然语言处理技术实现。使用nltk
或spaCy
提取关键词或重要句子,可以创建简洁的摘要。此外,transformers
库中的预训练模型,如BERT和GPT,可以生成更为自然和连贯的摘要。通过对输入文章进行分析,可以自动化生成高质量的摘要,节省时间和精力。
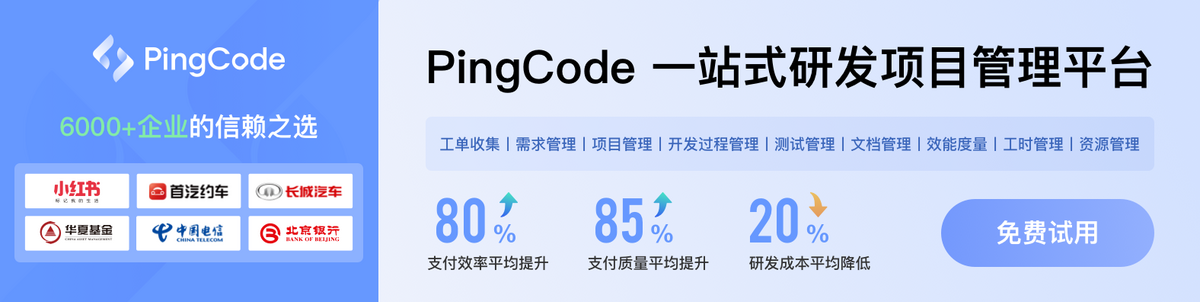