使用爬虫抓取资源的步骤包括选择合适的工具、制定抓取策略、处理反爬措施、解析数据并存储。 其中,选择合适的工具是最为关键的一步。Python中有许多爬虫工具和框架可以使用,例如Requests、BeautifulSoup、Scrapy等。Requests用于发送HTTP请求,BeautifulSoup用于解析网页,Scrapy则是一个功能强大且灵活的爬虫框架。本文将详细讲解如何使用这些工具进行数据抓取。
一、选择合适的工具
Python中有许多爬虫工具和框架,每个工具都有其独特的功能和适用场景。
1、Requests
Requests是一个简单易用的HTTP库,主要用于发送HTTP请求。它可以轻松地发送GET、POST等请求,并处理响应。
import requests
response = requests.get('https://example.com')
print(response.text)
2、BeautifulSoup
BeautifulSoup是一个解析HTML和XML的库,常与Requests结合使用。它能够方便地提取网页中的数据。
from bs4 import BeautifulSoup
import requests
response = requests.get('https://example.com')
soup = BeautifulSoup(response.text, 'html.parser')
print(soup.title.text)
3、Scrapy
Scrapy是一个强大的爬虫框架,适用于复杂的爬虫任务。它具有高效、灵活、易扩展的特点,适合用于大规模的爬取任务。
import scrapy
class ExampleSpider(scrapy.Spider):
name = 'example'
start_urls = ['https://example.com']
def parse(self, response):
title = response.css('title::text').get()
yield {'title': title}
二、制定抓取策略
制定抓取策略是确保爬虫高效、稳定地运行的关键。抓取策略包括确定抓取目标、设置抓取频率、处理分页等。
1、确定抓取目标
在开始抓取之前,需要明确抓取的目标网站和数据。可以通过浏览器的开发者工具查看网页结构,找出需要的数据位置。
2、设置抓取频率
为了避免对目标网站造成过大压力,需要设置合理的抓取频率。可以通过设置延迟或使用随机间隔来控制抓取速度。
import time
import random
time.sleep(random.uniform(1, 3))
3、处理分页
许多网站的数据是分页展示的,需要编写代码处理分页逻辑,确保抓取到所有的数据。
import requests
from bs4 import BeautifulSoup
page = 1
while True:
response = requests.get(f'https://example.com/page/{page}')
soup = BeautifulSoup(response.text, 'html.parser')
data = soup.find_all('div', class_='data')
if not data:
break
for item in data:
print(item.text)
page += 1
三、处理反爬措施
许多网站都会有反爬措施,如限制IP访问频率、检测用户代理等。需要采取相应措施来应对这些反爬机制。
1、更换IP
可以使用代理服务器来更换IP,避免被网站封禁。市面上有许多代理服务提供商,可以选择合适的服务。
import requests
proxies = {
'http': 'http://10.10.10.10:8000',
'https': 'http://10.10.10.10:8000',
}
response = requests.get('https://example.com', proxies=proxies)
print(response.text)
2、更换用户代理
更换用户代理可以伪装成不同的浏览器,避免被检测为爬虫。可以随机选择用户代理来发送请求。
import requests
import random
user_agents = [
'Mozilla/5.0 (Windows NT 10.0; Win64; x64) AppleWebKit/537.36 (KHTML, like Gecko) Chrome/91.0.4472.124 Safari/537.36',
'Mozilla/5.0 (Macintosh; Intel Mac OS X 10_15_7) AppleWebKit/605.1.15 (KHTML, like Gecko) Version/14.1.1 Safari/605.1.15',
]
headers = {'User-Agent': random.choice(user_agents)}
response = requests.get('https://example.com', headers=headers)
print(response.text)
四、解析数据
抓取到网页后,需要对网页内容进行解析,提取出需要的数据。可以使用正则表达式、BeautifulSoup、lxml等工具进行解析。
1、使用BeautifulSoup解析
BeautifulSoup是一个强大的HTML解析库,可以方便地提取网页中的数据。
from bs4 import BeautifulSoup
import requests
response = requests.get('https://example.com')
soup = BeautifulSoup(response.text, 'html.parser')
titles = soup.find_all('h1')
for title in titles:
print(title.text)
2、使用正则表达式解析
正则表达式是一种强大的文本匹配工具,适用于结构简单的网页内容提取。
import re
import requests
response = requests.get('https://example.com')
titles = re.findall(r'<h1>(.*?)</h1>', response.text)
for title in titles:
print(title)
五、存储数据
抓取到的数据需要进行存储,以便后续的分析和使用。常见的存储方式包括文件存储、数据库存储等。
1、文件存储
可以将数据存储到本地文件,如CSV、JSON等格式。
import csv
data = [{'title': 'Example 1'}, {'title': 'Example 2'}]
with open('data.csv', 'w', newline='') as file:
writer = csv.DictWriter(file, fieldnames=['title'])
writer.writeheader()
writer.writerows(data)
2、数据库存储
对于大规模的数据,可以使用数据库进行存储,如MySQL、MongoDB等。
import pymysql
connection = pymysql.connect(host='localhost',
user='user',
password='passwd',
database='db')
cursor = connection.cursor()
cursor.execute('CREATE TABLE IF NOT EXISTS data (title VARCHAR(255))')
data = [{'title': 'Example 1'}, {'title': 'Example 2'}]
for item in data:
cursor.execute('INSERT INTO data (title) VALUES (%s)', (item['title'],))
connection.commit()
connection.close()
六、处理动态网页
许多现代网站使用JavaScript动态加载内容,传统的静态解析方法无法抓取到这些数据。可以使用Selenium等工具来处理动态网页。
1、使用Selenium
Selenium是一个自动化测试工具,可以模拟浏览器操作,适用于抓取动态加载的网页内容。
from selenium import webdriver
driver = webdriver.Chrome()
driver.get('https://example.com')
titles = driver.find_elements_by_tag_name('h1')
for title in titles:
print(title.text)
driver.quit()
2、使用Splash
Splash是一个JavaScript渲染服务,可以用于抓取动态网页内容。Scrapy与Splash结合使用可以高效地抓取动态网页。
import scrapy
from scrapy_splash import SplashRequest
class ExampleSpider(scrapy.Spider):
name = 'example'
start_urls = ['https://example.com']
def start_requests(self):
for url in self.start_urls:
yield SplashRequest(url, self.parse, args={'wait': 2})
def parse(self, response):
title = response.css('title::text').get()
yield {'title': title}
七、提高爬虫效率
对于大规模的数据抓取任务,需要提高爬虫的效率。可以通过异步爬取、多线程等方式来提升爬虫的性能。
1、异步爬取
使用异步编程可以提高爬虫的效率,asyncio和aiohttp是常用的异步爬虫工具。
import asyncio
import aiohttp
async def fetch(url):
async with aiohttp.ClientSession() as session:
async with session.get(url) as response:
return await response.text()
async def main():
urls = ['https://example.com/page/1', 'https://example.com/page/2']
tasks = [fetch(url) for url in urls]
results = await asyncio.gather(*tasks)
for result in results:
print(result)
asyncio.run(main())
2、多线程爬取
使用多线程可以并发地抓取数据,提高爬虫的效率。线程池可以方便地管理多线程任务。
from concurrent.futures import ThreadPoolExecutor
import requests
def fetch(url):
response = requests.get(url)
return response.text
urls = ['https://example.com/page/1', 'https://example.com/page/2']
with ThreadPoolExecutor(max_workers=2) as executor:
results = executor.map(fetch, urls)
for result in results:
print(result)
八、处理反反爬措施
一些网站会采取高级的反爬措施,如验证码、滑动验证等。需要使用相应的技术来处理这些反反爬措施。
1、处理验证码
对于验证码,可以使用图像识别技术来自动识别。可以选择开源的OCR库,如Tesseract。
import pytesseract
from PIL import Image
image = Image.open('captcha.png')
text = pytesseract.image_to_string(image)
print(text)
2、处理滑动验证
对于滑动验证,可以使用Selenium模拟滑动操作,破解验证。
from selenium import webdriver
from selenium.webdriver.common.action_chains import ActionChains
driver = webdriver.Chrome()
driver.get('https://example.com')
slider = driver.find_element_by_id('slider')
action = ActionChains(driver)
action.click_and_hold(slider).move_by_offset(200, 0).release().perform()
九、爬虫的法律与道德
在进行爬虫操作时,需要遵守法律与道德规范。确保不侵犯他人的知识产权和隐私权,不对目标网站造成过大压力。
1、遵守机器人协议
许多网站会通过robots.txt文件来指示哪些页面允许被抓取,哪些页面禁止抓取。需要遵守这些规定。
import requests
response = requests.get('https://example.com/robots.txt')
print(response.text)
2、尊重知识产权
确保抓取的数据不侵犯他人的知识产权。不要抓取受版权保护的内容,或在未经授权的情况下使用抓取的数据。
十、总结
爬虫是一项复杂且有趣的技术,涉及到多个方面的知识。本文详细介绍了如何使用Python进行数据抓取,包括选择合适的工具、制定抓取策略、处理反爬措施、解析数据并存储等。希望通过本文的介绍,能帮助你更好地掌握爬虫技术。
相关问答FAQs:
如何选择合适的Python爬虫库进行抓取?
在选择Python爬虫库时,可以考虑使用Beautiful Soup、Scrapy或Requests等。Beautiful Soup适合简单的HTML解析,Scrapy则更强大,适合大规模爬取和数据处理。Requests库则用于发送HTTP请求,获取网页内容。根据项目的复杂程度和数据量来决定最适合的库。
抓取网站数据时需要注意哪些法律和道德问题?
在抓取网站数据时,遵守robots.txt文件中的爬虫协议是非常重要的。许多网站明确规定了允许或禁止爬虫访问的内容。此外,避免对网站造成过大负载,合理设置请求频率,以免影响网站的正常运行。尊重知识产权和个人隐私,确保抓取的数据不会用于不当用途。
如何处理抓取过程中遇到的反爬虫机制?
反爬虫机制可能会导致抓取失败,常见的措施包括修改请求头、使用代理IP和设置随机的请求间隔。可以通过伪装成浏览器的User-Agent来避免被识别为爬虫,同时使用代理可以隐藏真实IP地址,降低被封的风险。此外,采用延时策略,使请求间隔不固定,可以有效减少被检测的机会。
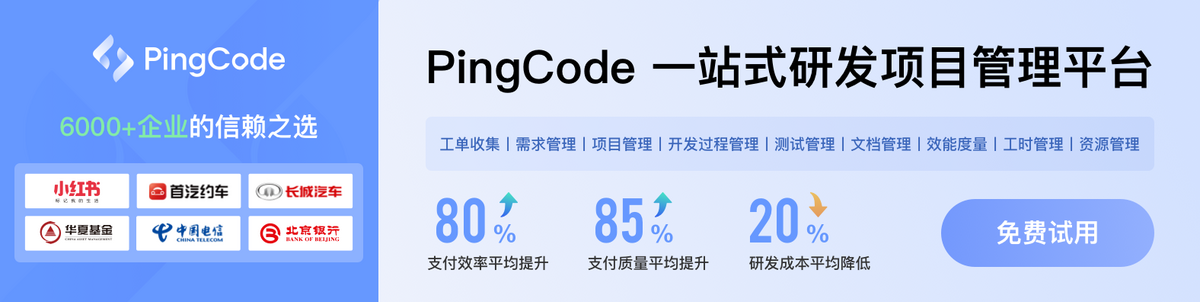