使用Python编写语音交互应用程序需要使用一些特定的库和工具,如SpeechRecognition、pyttsx3、gTTS以及NLTK。通过安装并导入这些库,可以实现语音识别、语音合成以及自然语言处理等功能,从而创建一个完整的语音交互系统。其中,SpeechRecognition库用于将语音转换为文本,pyttsx3和gTTS用于将文本转换为语音,而NLTK则可以用于处理和理解自然语言。我们将详细描述如何使用这些工具实现语音交互功能。
一、安装所需库
在开始编写语音交互应用程序之前,我们需要安装一些必要的Python库。可以使用pip命令来安装这些库:
pip install SpeechRecognition
pip install pyttsx3
pip install gtts
pip install nltk
pip install pyaudio
这些库分别用于语音识别、文本转语音转换、自然语言处理以及音频输入输出。
二、语音识别
Python的SpeechRecognition库提供了多种语音识别API。我们将使用Google Web Speech API来进行语音识别。以下是一个简单的示例代码,展示了如何使用SpeechRecognition库将语音转换为文本:
import speech_recognition as sr
创建语音识别对象
recognizer = sr.Recognizer()
使用麦克风录制音频
with sr.Microphone() as source:
print("请说话...")
audio = recognizer.listen(source)
try:
# 使用Google Web Speech API进行语音识别
text = recognizer.recognize_google(audio, language='zh-CN')
print("你说的是: " + text)
except sr.UnknownValueError:
print("无法识别语音")
except sr.RequestError as e:
print("无法请求结果; {0}".format(e))
在这段代码中,我们创建了一个语音识别对象,并使用麦克风录制音频。然后,我们使用Google Web Speech API将录制的音频转换为文本。如果语音识别成功,打印识别到的文本;如果失败,打印相应的错误信息。
三、文本转语音
Python的pyttsx3和gTTS库可以用于将文本转换为语音。pyttsx3是一个离线的文本转语音库,而gTTS(Google Text-to-Speech)则是一个在线库。以下是使用pyttsx3和gTTS将文本转换为语音的示例代码:
使用pyttsx3:
import pyttsx3
创建文本转语音引擎
engine = pyttsx3.init()
设置语音属性
engine.setProperty('rate', 150) # 语速
engine.setProperty('volume', 1) # 音量
将文本转换为语音并播放
text = "你好,欢迎使用语音交互系统。"
engine.say(text)
engine.runAndWait()
使用gTTS:
from gtts import gTTS
import os
将文本转换为语音
text = "你好,欢迎使用语音交互系统。"
tts = gTTS(text=text, lang='zh-CN')
保存语音文件并播放
tts.save("output.mp3")
os.system("mpg321 output.mp3")
在这两个示例中,我们首先创建了一个文本转语音引擎,然后设置了语音属性(如语速和音量),最后将文本转换为语音并播放。
四、自然语言处理
为了实现更复杂的语音交互功能,我们可以使用自然语言处理(NLP)技术来理解和处理用户的语音输入。Python的NLTK库是一个强大的NLP工具包,可以帮助我们进行文本处理和理解。
以下是一个简单的示例代码,展示了如何使用NLTK库进行基本的自然语言处理:
import nltk
from nltk.tokenize import word_tokenize
from nltk.corpus import stopwords
下载必要的NLTK数据
nltk.download('punkt')
nltk.download('stopwords')
定义一个示例文本
text = "你好,我想了解一下今天的天气情况。"
分词
tokens = word_tokenize(text)
去除停用词
stop_words = set(stopwords.words('chinese'))
filtered_tokens = [word for word in tokens if word not in stop_words]
print("分词结果: ", tokens)
print("去除停用词后: ", filtered_tokens)
在这个示例中,我们使用NLTK库对文本进行分词,并去除停用词。首先,我们下载了必要的NLTK数据,然后定义了一个示例文本。接着,我们使用word_tokenize函数对文本进行分词,并使用stopwords模块去除停用词。
五、整合语音识别、文本转语音和自然语言处理
现在,我们可以将语音识别、文本转语音和自然语言处理整合在一起,创建一个完整的语音交互系统。以下是一个示例代码,展示了如何实现这一整合:
import speech_recognition as sr
import pyttsx3
import nltk
from nltk.tokenize import word_tokenize
from nltk.corpus import stopwords
下载必要的NLTK数据
nltk.download('punkt')
nltk.download('stopwords')
创建语音识别对象
recognizer = sr.Recognizer()
创建文本转语音引擎
engine = pyttsx3.init()
engine.setProperty('rate', 150)
engine.setProperty('volume', 1)
def recognize_speech():
with sr.Microphone() as source:
print("请说话...")
audio = recognizer.listen(source)
try:
text = recognizer.recognize_google(audio, language='zh-CN')
print("你说的是: " + text)
return text
except sr.UnknownValueError:
print("无法识别语音")
return None
except sr.RequestError as e:
print("无法请求结果; {0}".format(e))
return None
def process_text(text):
tokens = word_tokenize(text)
stop_words = set(stopwords.words('chinese'))
filtered_tokens = [word for word in tokens if word not in stop_words]
return filtered_tokens
def respond(text):
engine.say(text)
engine.runAndWait()
def main():
while True:
speech_text = recognize_speech()
if speech_text:
processed_text = process_text(speech_text)
print("处理后的文本: ", processed_text)
if "天气" in processed_text:
respond("今天的天气晴朗,气温25度。")
elif "时间" in processed_text:
respond("现在是下午3点。")
else:
respond("对不起,我不明白你的意思。")
if __name__ == "__main__":
main()
在这个示例中,我们首先创建了一个语音识别对象和一个文本转语音引擎。接着,我们定义了三个函数:recognize_speech用于语音识别,process_text用于文本处理,respond用于文本转语音。最后,我们在main函数中使用一个循环来不断地进行语音识别、文本处理和语音响应。
六、进一步扩展
上述示例展示了一个基本的语音交互系统,但实际应用中可能需要更多的功能和更复杂的处理。以下是一些可以进一步扩展的方向:
-
语义理解:可以使用更高级的自然语言处理技术,如命名实体识别(NER)、依存句法分析等,来更好地理解用户的意图。
-
多轮对话:可以实现多轮对话功能,通过上下文信息来理解用户的连续输入,并给出相应的响应。
-
语音合成:可以使用更高级的语音合成技术,如WaveNet、Tacotron等,来生成更自然的语音。
-
集成外部API:可以集成外部API,如天气API、新闻API等,来提供更多的信息和服务。
-
图形用户界面(GUI):可以使用PyQt、Tkinter等库来创建一个图形用户界面,使语音交互系统更加友好和直观。
以下是一个扩展示例,展示了如何集成天气API来提供天气信息:
import requests
def get_weather(city):
api_key = "your_api_key_here"
base_url = "http://api.openweathermap.org/data/2.5/weather?"
complete_url = base_url + "q=" + city + "&appid=" + api_key + "&units=metric"
response = requests.get(complete_url)
data = response.json()
if data["cod"] != "404":
main = data["main"]
weather = data["weather"][0]
weather_description = weather["description"]
temperature = main["temp"]
return f"{city}的天气情况:{weather_description},气温{temperature}度。"
else:
return "无法获取天气信息"
def main():
while True:
speech_text = recognize_speech()
if speech_text:
processed_text = process_text(speech_text)
print("处理后的文本: ", processed_text)
if "天气" in processed_text:
city = "北京" # 可以从用户输入中提取城市信息
weather_info = get_weather(city)
respond(weather_info)
elif "时间" in processed_text:
respond("现在是下午3点。")
else:
respond("对不起,我不明白你的意思。")
if __name__ == "__main__":
main()
在这个示例中,我们定义了一个get_weather函数,通过调用OpenWeatherMap API来获取天气信息。然后,我们在main函数中调用get_weather函数,并将获取到的天气信息通过语音合成响应给用户。
通过不断扩展和改进,可以创建一个功能强大、用户友好的语音交互系统,满足各种应用场景的需求。
相关问答FAQs:
如何在Python中实现语音识别功能?
在Python中,可以使用库如SpeechRecognition来实现语音识别功能。这个库支持多种语音识别API,包括Google的语音识别API。用户只需安装该库,然后通过简单的代码就能将语音转换为文本。示例代码如下:
import speech_recognition as sr
recognizer = sr.Recognizer()
with sr.Microphone() as source:
print("请说话:")
audio = recognizer.listen(source)
try:
print("你说的是: " + recognizer.recognize_google(audio, language="zh-CN"))
except sr.UnknownValueError:
print("无法识别音频")
except sr.RequestError:
print("无法连接到语音识别服务")
通过这样的代码,用户可以轻松实现语音输入。
如何将Python中的语音输出与语音输入结合使用?
结合语音输入和输出可以通过使用pyttsx3库实现语音合成。用户可以在识别到语音后,使用该库将文本转化为语音进行反馈。例如,用户可以在识别到特定命令后,让程序用语音回答或执行相应操作。下面是一个简单的示例:
import pyttsx3
engine = pyttsx3.init()
engine.say("你好,我可以帮助你吗?")
engine.runAndWait()
通过结合这两个库,用户可以创建一个完整的语音交互系统。
如何优化Python语音交互程序的性能?
为了提升Python语音交互程序的性能,可以考虑几个方面。首先,选择更强大的语音识别API,例如使用离线识别或更高级的API。其次,优化麦克风的输入设置,确保环境安静,以减少背景噪音的干扰。此外,使用多线程来处理语音识别和语音合成任务,可以提升用户体验,使交互更加流畅。
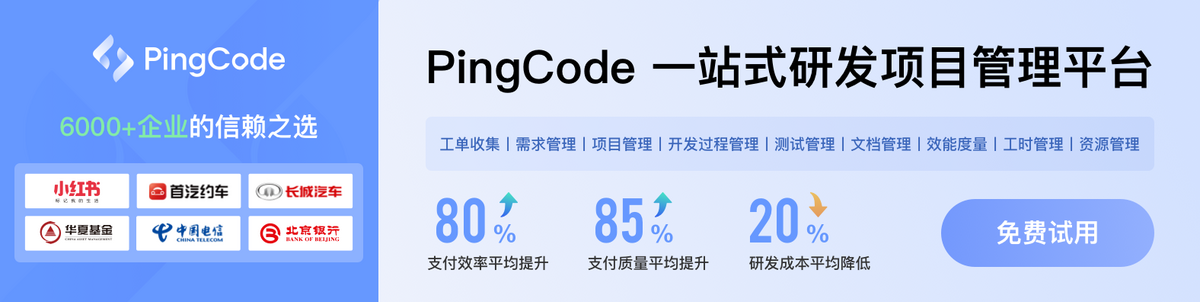