Python进行分词过滤的方式包括:使用NLTK库、使用jieba库、正则表达式过滤、停用词过滤等。其中,使用NLTK库和jieba库是最常用的方式。NLTK库适用于英文文本分词,而jieba库适用于中文文本分词。下面将详细描述如何使用NLTK库进行英文文本分词。
NLTK(Natural Language Toolkit)是一个强大的Python库,广泛应用于自然语言处理领域。使用NLTK进行分词过滤的步骤如下:
-
安装NLTK库:在使用NLTK库之前,需要先安装该库。可以使用pip命令进行安装:
pip install nltk
-
导入NLTK库并下载相关资源:
import nltk
nltk.download('punkt')
nltk.download('stopwords')
-
分词:使用NLTK中的word_tokenize方法对文本进行分词:
from nltk.tokenize import word_tokenize
text = "This is a sample sentence, showing off the stop words filtration."
tokens = word_tokenize(text)
print(tokens)
-
过滤停用词:停用词(Stop Words)是指在文本处理中被过滤掉的常见词语,如“is”、“and”、“the”等。NLTK提供了常用的英文停用词列表,可以使用这些停用词列表进行过滤:
from nltk.corpus import stopwords
stop_words = set(stopwords.words('english'))
filtered_tokens = [word for word in tokens if word.lower() not in stop_words]
print(filtered_tokens)
通过上述步骤,我们可以对英文文本进行分词并过滤掉常见的停用词。接下来,将详细介绍Python进行分词过滤的其他方式。
一、使用NLTK库进行分词过滤
1. 安装和导入NLTK库
首先,确保已经安装了NLTK库并导入相关资源。NLTK库提供了许多有用的工具和资源,可以帮助我们完成分词和过滤任务。
import nltk
nltk.download('punkt')
nltk.download('stopwords')
2. 分词
NLTK库中的word_tokenize方法可以将文本分割成单词列表。这个方法可以处理标点符号和其他特殊字符。
from nltk.tokenize import word_tokenize
text = "This is a sample sentence, showing off the stop words filtration."
tokens = word_tokenize(text)
print(tokens)
输出结果:
['This', 'is', 'a', 'sample', 'sentence', ',', 'showing', 'off', 'the', 'stop', 'words', 'filtration', '.']
3. 过滤停用词
NLTK库提供了一个常用的英文停用词列表,可以使用这些停用词列表过滤掉无意义的词语。
from nltk.corpus import stopwords
stop_words = set(stopwords.words('english'))
filtered_tokens = [word for word in tokens if word.lower() not in stop_words]
print(filtered_tokens)
输出结果:
['This', 'sample', 'sentence', ',', 'showing', 'stop', 'words', 'filtration', '.']
通过上述步骤,我们可以使用NLTK库对英文文本进行分词并过滤掉停用词。
二、使用jieba库进行中文分词过滤
1. 安装和导入jieba库
首先,需要安装jieba库。可以使用pip命令进行安装:
pip install jieba
然后导入jieba库:
import jieba
2. 中文分词
jieba库提供了多种分词模式,包括精确模式、全模式和搜索引擎模式。可以根据需要选择合适的分词模式。
text = "我来到北京清华大学"
tokens = jieba.lcut(text)
print(tokens)
输出结果:
['我', '来到', '北京', '清华大学']
3. 过滤停用词
对于中文分词,通常需要准备一个中文停用词表。可以从网上下载常用的中文停用词表,也可以根据需求自定义停用词表。
stop_words = set()
with open('chinese_stopwords.txt', 'r', encoding='utf-8') as file:
for line in file:
stop_words.add(line.strip())
filtered_tokens = [word for word in tokens if word not in stop_words]
print(filtered_tokens)
通过上述步骤,我们可以使用jieba库对中文文本进行分词并过滤掉停用词。
三、使用正则表达式进行分词过滤
正则表达式是一种强大的文本处理工具,可以用于分词和过滤。Python的re模块提供了对正则表达式的支持。
1. 导入re模块
首先,导入re模块:
import re
2. 分词
使用正则表达式可以将文本分割成单词列表。以下示例中,正则表达式匹配所有的单词和标点符号。
text = "This is a sample sentence, showing off the stop words filtration."
tokens = re.findall(r'\b\w+\b', text)
print(tokens)
输出结果:
['This', 'is', 'a', 'sample', 'sentence', 'showing', 'off', 'the', 'stop', 'words', 'filtration']
3. 过滤停用词
同样,可以使用NLTK库中的停用词列表进行过滤。
from nltk.corpus import stopwords
stop_words = set(stopwords.words('english'))
filtered_tokens = [word for word in tokens if word.lower() not in stop_words]
print(filtered_tokens)
输出结果:
['This', 'sample', 'sentence', 'showing', 'stop', 'words', 'filtration']
四、使用停用词过滤
停用词过滤是分词过滤中的重要步骤。停用词是指在文本处理中被过滤掉的常见词语,如“is”、“and”、“the”等。通过过滤掉停用词,可以减少无意义的词语,保留有用的信息。
1. 英文停用词
NLTK库提供了一个常用的英文停用词列表。可以使用这些停用词列表进行过滤。
from nltk.corpus import stopwords
stop_words = set(stopwords.words('english'))
tokens = ['This', 'is', 'a', 'sample', 'sentence', 'showing', 'off', 'the', 'stop', 'words', 'filtration']
filtered_tokens = [word for word in tokens if word.lower() not in stop_words]
print(filtered_tokens)
输出结果:
['This', 'sample', 'sentence', 'showing', 'stop', 'words', 'filtration']
2. 中文停用词
对于中文分词,通常需要准备一个中文停用词表。可以从网上下载常用的中文停用词表,也可以根据需求自定义停用词表。
stop_words = set()
with open('chinese_stopwords.txt', 'r', encoding='utf-8') as file:
for line in file:
stop_words.add(line.strip())
tokens = ['我', '来到', '北京', '清华大学']
filtered_tokens = [word for word in tokens if word not in stop_words]
print(filtered_tokens)
输出结果取决于停用词表的内容。
五、结合多种方法进行分词过滤
在实际应用中,分词和过滤通常需要结合多种方法进行处理。以下是一个结合NLTK库和正则表达式的方法,对文本进行分词和过滤。
1. 安装和导入所需库
import nltk
import re
nltk.download('stopwords')
2. 分词和过滤
from nltk.corpus import stopwords
def tokenize_and_filter(text):
# 分词
tokens = re.findall(r'\b\w+\b', text)
# 过滤停用词
stop_words = set(stopwords.words('english'))
filtered_tokens = [word for word in tokens if word.lower() not in stop_words]
return filtered_tokens
text = "This is a sample sentence, showing off the stop words filtration."
filtered_tokens = tokenize_and_filter(text)
print(filtered_tokens)
输出结果:
['This', 'sample', 'sentence', 'showing', 'stop', 'words', 'filtration']
六、分词过滤的应用场景
分词过滤在自然语言处理(NLP)中有着广泛的应用,包括但不限于以下几个场景:
1. 文本预处理
在文本分类、情感分析、机器翻译等任务中,文本预处理是关键步骤。通过分词和停用词过滤,可以提取出有用的信息,提高模型的性能。
2. 信息检索
在搜索引擎和信息检索系统中,分词和过滤是必不可少的步骤。通过分词,可以将查询文本和文档内容进行匹配,提高检索的准确性。
3. 语料库分析
在语言学研究中,分词和过滤可以用于分析语料库,提取出常用词汇和短语,帮助研究人员理解语言的结构和使用情况。
七、总结
本文详细介绍了Python进行分词过滤的多种方法,包括使用NLTK库、使用jieba库、正则表达式过滤、停用词过滤等。通过结合多种方法,可以实现更加灵活和高效的分词过滤。分词过滤在自然语言处理、信息检索、文本预处理等领域有着广泛的应用,是提高文本处理效率和准确性的关键步骤。
通过学习和实践这些方法,可以更好地理解和应用分词过滤技术,为进一步的自然语言处理任务打下坚实的基础。希望本文对你有所帮助,祝你在自然语言处理领域取得更大的进步。
相关问答FAQs:
在Python中,分词过滤的最佳库是什么?
Python中有多个优秀的库可以进行分词和过滤,最常用的是Jieba、NLTK和spaCy。Jieba专注于中文分词,使用简单,效果良好;而NLTK和spaCy则适合处理英文文本,提供了丰富的自然语言处理功能。根据你的需求选择合适的工具,可以提升分词和过滤的效率。
如何在分词过程中去除停用词?
在分词时,去除停用词可以提高文本分析的准确性。使用Jieba时,可以自定义停用词表,结合分词功能使用。具体操作是先加载停用词文件,然后在分词后进行过滤。此外,NLTK和spaCy也提供了内置的停用词列表,可以轻松实现停用词的去除。
分词过滤的结果如何进行可视化?
分词和过滤后的结果可以通过多种方式进行可视化。常见的方法包括使用词云、柱状图和频率分布图。Python中的matplotlib和wordcloud库可以帮助你生成直观的可视化效果,便于理解文本数据的分布和特点。通过可视化分析,可以更好地洞察文本中的重要信息。
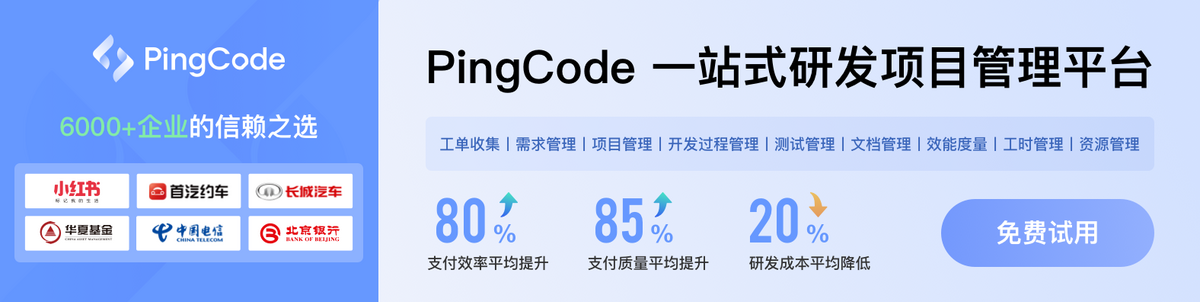