要用Python分析图片,可以使用OpenCV、PIL、scikit-image、TensorFlow、Keras、PyTorch等库。这些库提供了不同的工具和功能,使得在Python中处理和分析图像变得相对简单。例如,OpenCV是一个强大的计算机视觉库,适用于各种图像处理任务;PIL(Python Imaging Library)适合基本的图像操作;scikit-image提供了一些高级的图像处理功能;TensorFlow和Keras可以用于深度学习图像识别任务;PyTorch也是一个广泛使用的深度学习框架。具体使用哪种库取决于你的需求和项目规模。
下面将详细介绍如何用这些库进行图像分析。
一、使用OpenCV分析图像
OpenCV是一个开源的计算机视觉和机器学习软件库,广泛用于实时图像处理和分析。它支持多种编程语言,包括Python。
-
安装和导入OpenCV
首先需要安装OpenCV库,可以使用以下命令:
pip install opencv-python
然后在Python脚本中导入OpenCV:
import cv2
-
读取和显示图像
使用OpenCV读取和显示图像非常简单。
cv2.imread()
用于读取图像,cv2.imshow()
用于显示图像。image = cv2.imread('path_to_image.jpg')
cv2.imshow('Image', image)
cv2.waitKey(0)
cv2.destroyAllWindows()
-
图像处理
OpenCV提供了多种图像处理功能,例如灰度转换、边缘检测、图像平滑等。
-
灰度转换:
gray_image = cv2.cvtColor(image, cv2.COLOR_BGR2GRAY)
-
边缘检测:
使用Canny边缘检测:
edges = cv2.Canny(gray_image, 100, 200)
-
图像平滑:
例如高斯模糊:
blurred_image = cv2.GaussianBlur(image, (5, 5), 0)
-
-
特征检测
OpenCV也支持特征检测和描述,例如SIFT、SURF和ORB。
- ORB特征检测:
orb = cv2.ORB_create()
keypoints, descriptors = orb.detectAndCompute(image, None)
image_with_keypoints = cv2.drawKeypoints(image, keypoints, None)
特征检测在图像匹配、物体识别等任务中非常有用。
- ORB特征检测:
二、使用PIL处理图像
PIL(Python Imaging Library)是一个Python图像处理库,适合基本的图像操作。虽然PIL已经不再维护,但其派生库Pillow仍然广泛使用。
-
安装和导入Pillow
可以使用以下命令安装Pillow:
pip install pillow
然后在Python脚本中导入Pillow:
from PIL import Image
-
打开和显示图像
使用Pillow打开和显示图像:
image = Image.open('path_to_image.jpg')
image.show()
-
基本图像操作
Pillow支持多种基本图像操作,例如裁剪、旋转、调整大小。
-
裁剪:
cropped_image = image.crop((left, top, right, bottom))
-
旋转:
rotated_image = image.rotate(45)
-
调整大小:
resized_image = image.resize((width, height))
-
-
图像滤镜
Pillow提供了一些简单的图像滤镜:
from PIL import ImageFilter
blurred_image = image.filter(ImageFilter.BLUR)
三、使用scikit-image分析图像
scikit-image是一个基于SciPy的Python库,专门用于图像处理。它提供了一些高级的图像处理功能。
-
安装和导入scikit-image
可以使用以下命令安装scikit-image:
pip install scikit-image
然后在Python脚本中导入scikit-image:
from skimage import io
-
读取和显示图像
使用scikit-image读取和显示图像:
image = io.imread('path_to_image.jpg')
io.imshow(image)
io.show()
-
图像变换
scikit-image支持多种图像变换,例如颜色变换、几何变换。
-
颜色变换:
from skimage.color import rgb2gray
gray_image = rgb2gray(image)
-
几何变换:
from skimage.transform import rotate
rotated_image = rotate(image, angle=45)
-
-
图像分割
图像分割是图像分析中的一个重要步骤。scikit-image提供了多种分割算法。
from skimage.filters import threshold_otsu
thresh = threshold_otsu(gray_image)
binary_image = gray_image > thresh
四、使用TensorFlow和Keras进行深度学习图像分析
TensorFlow和Keras是用于深度学习的流行框架,常用于图像分类和识别任务。
-
安装和导入TensorFlow和Keras
可以使用以下命令安装TensorFlow和Keras:
pip install tensorflow
然后在Python脚本中导入TensorFlow和Keras:
import tensorflow as tf
from tensorflow import keras
-
加载和预处理数据
使用Keras的数据集模块可以轻松加载和预处理图像数据:
(train_images, train_labels), (test_images, test_labels) = keras.datasets.cifar10.load_data()
train_images = train_images / 255.0
test_images = test_images / 255.0
-
构建模型
使用Keras构建深度学习模型:
model = keras.Sequential([
keras.layers.Flatten(input_shape=(32, 32, 3)),
keras.layers.Dense(128, activation='relu'),
keras.layers.Dense(10, activation='softmax')
])
-
编译和训练模型
编译和训练模型:
model.compile(optimizer='adam',
loss='sparse_categorical_crossentropy',
metrics=['accuracy'])
model.fit(train_images, train_labels, epochs=10)
-
评估模型
使用测试数据评估模型:
test_loss, test_acc = model.evaluate(test_images, test_labels)
print('Test accuracy:', test_acc)
五、使用PyTorch进行深度学习图像分析
PyTorch是另一个广泛使用的深度学习框架,具有灵活的设计和动态计算图。
-
安装和导入PyTorch
可以使用以下命令安装PyTorch:
pip install torch torchvision
然后在Python脚本中导入PyTorch:
import torch
import torchvision
import torchvision.transforms as transforms
-
加载和预处理数据
使用torchvision加载和预处理CIFAR-10数据集:
transform = transforms.Compose(
[transforms.ToTensor(),
transforms.Normalize((0.5, 0.5, 0.5), (0.5, 0.5, 0.5))])
trainset = torchvision.datasets.CIFAR10(root='./data', train=True,
download=True, transform=transform)
trainloader = torch.utils.data.DataLoader(trainset, batch_size=4,
shuffle=True, num_workers=2)
-
定义模型
使用torch.nn定义卷积神经网络:
import torch.nn as nn
import torch.nn.functional as F
class Net(nn.Module):
def __init__(self):
super(Net, self).__init__()
self.conv1 = nn.Conv2d(3, 6, 5)
self.pool = nn.MaxPool2d(2, 2)
self.conv2 = nn.Conv2d(6, 16, 5)
self.fc1 = nn.Linear(16 * 5 * 5, 120)
self.fc2 = nn.Linear(120, 84)
self.fc3 = nn.Linear(84, 10)
def forward(self, x):
x = self.pool(F.relu(self.conv1(x)))
x = self.pool(F.relu(self.conv2(x)))
x = x.view(-1, 16 * 5 * 5)
x = F.relu(self.fc1(x))
x = F.relu(self.fc2(x))
x = self.fc3(x)
return x
net = Net()
-
定义损失函数和优化器
使用交叉熵损失和随机梯度下降优化器:
import torch.optim as optim
criterion = nn.CrossEntropyLoss()
optimizer = optim.SGD(net.parameters(), lr=0.001, momentum=0.9)
-
训练模型
训练模型:
for epoch in range(2): # loop over the dataset multiple times
running_loss = 0.0
for i, data in enumerate(trainloader, 0):
# get the inputs; data is a list of [inputs, labels]
inputs, labels = data
# zero the parameter gradients
optimizer.zero_grad()
# forward + backward + optimize
outputs = net(inputs)
loss = criterion(outputs, labels)
loss.backward()
optimizer.step()
# print statistics
running_loss += loss.item()
if i % 2000 == 1999: # print every 2000 mini-batches
print('[%d, %5d] loss: %.3f' %
(epoch + 1, i + 1, running_loss / 2000))
running_loss = 0.0
print('Finished Training')
-
评估模型
使用测试数据评估模型:
correct = 0
total = 0
with torch.no_grad():
for data in testloader:
images, labels = data
outputs = net(images)
_, predicted = torch.max(outputs.data, 1)
total += labels.size(0)
correct += (predicted == labels).sum().item()
print('Accuracy of the network on the 10000 test images: %d %%' % (
100 * correct / total))
通过以上介绍,可以看到Python提供了丰富的图像分析库和工具,每个库都有其独特的功能和使用场景。选择合适的库来进行图像分析,可以大大提高工作效率和分析效果。
相关问答FAQs:
如何开始使用Python进行图像分析?
要开始使用Python进行图像分析,您需要安装一些基础库,如OpenCV和Pillow。这些库提供了强大的图像处理功能。您可以通过pip命令轻松安装它们:pip install opencv-python pillow
。接着,您可以使用这些库加载图像、进行基本处理,如调整大小、裁剪和过滤等。
Python图像分析中常用的技术有哪些?
在图像分析中,常用的技术包括边缘检测、特征提取、图像分割和模式识别。边缘检测可以帮助识别图像中的物体轮廓,而特征提取则有助于提取重要信息以供后续分析。图像分割技术常用于将图像分割成多个部分,以便更好地分析每一部分。
有哪些实用的Python库可以用于图像分析?
除了OpenCV和Pillow,其他一些有用的Python库包括scikit-image、TensorFlow和Keras。scikit-image专注于图像处理和计算机视觉任务,而TensorFlow和Keras则提供了深度学习框架,可以用于更复杂的图像分析任务,如图像分类和对象检测。这些库的结合能够满足不同层次的图像分析需求。
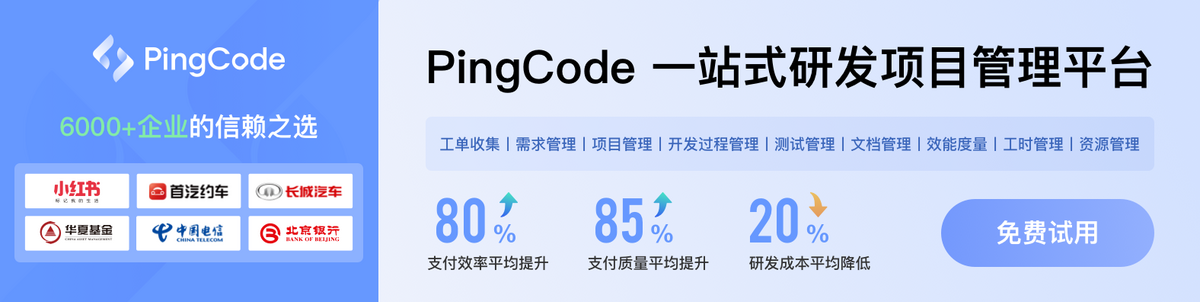