Python如何使用GPU运算
Python使用GPU运算的方式有多种,如使用CUDA、利用TensorFlow、PyTorch等深度学习框架、通过CuPy库进行GPU计算。本文将详细介绍其中的几种方法,帮助你更好地理解并应用GPU运算。
一、CUDA和NVIDIA GPU
CUDA(Compute Unified Device Architecture)是由NVIDIA开发的一种并行计算架构,能够充分挖掘GPU的计算能力。为了在Python中使用CUDA,需要安装NVIDIA的CUDA Toolkit和cuDNN。以下是具体步骤:
-
安装CUDA Toolkit和cuDNN:
首先,需要确保你的系统安装了NVIDIA驱动程序,然后从NVIDIA官网下载并安装CUDA Toolkit和cuDNN。
-
安装pycuda库:
pycuda
是Python调用CUDA的一个库,可以通过pip安装:pip install pycuda
-
编写CUDA代码:
使用pycuda编写一些简单的CUDA代码,例如向量加法。
import pycuda.autoinit
import pycuda.driver as drv
import numpy as np
from pycuda.compiler import SourceModule
mod = SourceModule("""
__global__ void add(float *a, float *b, float *c)
{
int idx = threadIdx.x + blockDim.x * blockIdx.x;
c[idx] = a[idx] + b[idx];
}
""")
add = mod.get_function("add")
a = np.random.randn(400).astype(np.float32)
b = np.random.randn(400).astype(np.float32)
c = np.zeros_like(a)
add(
drv.In(a), drv.In(b), drv.Out(c),
block=(400, 1, 1), grid=(1, 1, 1)
)
print(c - (a + b))
二、TensorFlow和GPU
TensorFlow是一个广泛使用的开源深度学习框架,支持GPU加速。使用GPU进行计算可以显著提高模型训练和推理的速度。
-
安装TensorFlow:
使用pip安装TensorFlow的GPU版本:
pip install tensorflow-gpu
-
检查TensorFlow是否使用GPU:
安装完成后,可以通过以下代码检查TensorFlow是否成功使用了GPU:
import tensorflow as tf
print("Num GPUs Available: ", len(tf.config.experimental.list_physical_devices('GPU')))
-
编写TensorFlow代码:
编写一个简单的神经网络,并在GPU上运行:
import tensorflow as tf
from tensorflow.keras import layers, models
model = models.Sequential()
model.add(layers.Conv2D(32, (3, 3), activation='relu', input_shape=(28, 28, 1)))
model.add(layers.MaxPooling2D((2, 2)))
model.add(layers.Conv2D(64, (3, 3), activation='relu'))
model.add(layers.MaxPooling2D((2, 2)))
model.add(layers.Conv2D(64, (3, 3), activation='relu'))
model.add(layers.Flatten())
model.add(layers.Dense(64, activation='relu'))
model.add(layers.Dense(10, activation='softmax'))
model.compile(optimizer='adam', loss='sparse_categorical_crossentropy', metrics=['accuracy'])
Load and preprocess data
(train_images, train_labels), (test_images, test_labels) = tf.keras.datasets.mnist.load_data()
train_images = train_images.reshape((60000, 28, 28, 1)).astype('float32') / 255
test_images = test_images.reshape((10000, 28, 28, 1)).astype('float32') / 255
Train the model
model.fit(train_images, train_labels, epochs=5, batch_size=64, validation_data=(test_images, test_labels))
三、PyTorch和GPU
PyTorch是另一个流行的深度学习框架,同样支持GPU加速。它以其动态计算图和灵活性受到广泛欢迎。
-
安装PyTorch:
使用pip安装PyTorch的GPU版本:
pip install torch torchvision torchaudio
-
检查PyTorch是否使用GPU:
安装完成后,可以通过以下代码检查PyTorch是否成功使用了GPU:
import torch
print(torch.cuda.is_available())
-
编写PyTorch代码:
编写一个简单的神经网络,并在GPU上运行:
import torch
import torch.nn as nn
import torch.optim as optim
import torchvision
import torchvision.transforms as transforms
device = torch.device("cuda" if torch.cuda.is_available() else "cpu")
transform = transforms.Compose([transforms.ToTensor(), transforms.Normalize((0.5,), (0.5,))])
trainset = torchvision.datasets.MNIST(root='./data', train=True, download=True, transform=transform)
trainloader = torch.utils.data.DataLoader(trainset, batch_size=64, shuffle=True)
class Net(nn.Module):
def __init__(self):
super(Net, self).__init__()
self.conv1 = nn.Conv2d(1, 32, 3, 1)
self.conv2 = nn.Conv2d(32, 64, 3, 1)
self.fc1 = nn.Linear(9216, 128)
self.fc2 = nn.Linear(128, 10)
def forward(self, x):
x = self.conv1(x)
x = nn.functional.relu(x)
x = self.conv2(x)
x = nn.functional.relu(x)
x = nn.functional.max_pool2d(x, 2)
x = torch.flatten(x, 1)
x = self.fc1(x)
x = nn.functional.relu(x)
x = self.fc2(x)
return nn.functional.log_softmax(x, dim=1)
net = Net().to(device)
criterion = nn.CrossEntropyLoss()
optimizer = optim.Adam(net.parameters())
for epoch in range(5):
running_loss = 0.0
for i, data in enumerate(trainloader, 0):
inputs, labels = data[0].to(device), data[1].to(device)
optimizer.zero_grad()
outputs = net(inputs)
loss = criterion(outputs, labels)
loss.backward()
optimizer.step()
running_loss += loss.item()
if i % 100 == 99:
print('[%d, %5d] loss: %.3f' % (epoch + 1, i + 1, running_loss / 100))
running_loss = 0.0
print('Finished Training')
四、CuPy库
CuPy是一个与NumPy兼容的数组库,旨在利用NVIDIA GPU进行高效的并行计算。它可以大大加速数组运算。
-
安装CuPy:
使用pip安装CuPy:
pip install cupy-cuda11x # 选择适合你CUDA版本的安装包
-
使用CuPy进行数组计算:
使用CuPy编写一些简单的数组运算代码:
import cupy as cp
a = cp.array([1, 2, 3, 4, 5])
b = cp.array([6, 7, 8, 9, 10])
c = a + b
print(c) # Output: [ 7 9 11 13 15]
-
性能比较:
可以比较CuPy和NumPy的性能差异:
import numpy as np
import cupy as cp
import time
n = 1000000
a_np = np.random.rand(n)
b_np = np.random.rand(n)
start = time.time()
c_np = a_np + b_np
print('NumPy time:', time.time() - start)
a_cp = cp.random.rand(n)
b_cp = cp.random.rand(n)
start = time.time()
c_cp = a_cp + b_cp
cp.cuda.Stream.null.synchronize() # Ensure all operations are finished
print('CuPy time:', time.time() - start)
五、总结
Python使用GPU运算的方式多种多样,包括使用CUDA、TensorFlow、PyTorch和CuPy等方法。通过GPU加速,能够显著提高计算性能,特别是在深度学习和科学计算领域。具体选择哪种方法,可以根据实际需求和开发环境来决定。希望本文能帮助你更好地理解和应用Python中的GPU运算,提高你的计算效率。
相关问答FAQs:
1. 使用GPU运算能带来哪些性能提升?
GPU运算通常能够显著提高计算性能,尤其是在处理大规模数据和复杂计算任务时。与CPU相比,GPU具有更多的核心,能够并行处理多个任务。这种并行计算能力使得深度学习、图像处理和科学计算等领域的运算速度大幅提升,尤其是在处理矩阵运算和向量计算时,GPU的优势更加明显。
2. 在Python中如何配置和使用GPU?
要在Python中使用GPU,首先需要确保你的计算机配备了兼容的GPU,并安装了适当的驱动程序。接下来,可以使用深度学习框架如TensorFlow或PyTorch,这些框架提供了对GPU的支持。在TensorFlow中,可以通过tf.config.experimental.list_physical_devices('GPU')
来检查是否成功识别GPU。在PyTorch中,使用torch.cuda.is_available()
来确认GPU是否可用。之后,用户可以将模型和数据转移到GPU上,以实现更快的计算。
3. 哪些Python库支持GPU加速?
多种Python库支持GPU加速,最常用的包括TensorFlow和PyTorch,它们是深度学习领域的主流框架。此外,NumPy的CuPy库提供了类似于NumPy的接口,但利用GPU进行计算。其他如NVIDIA的 RAPIDS 也是针对数据科学的库,专门优化了GPU的计算性能。对于图像处理,OpenCV也提供了GPU加速的功能,用户可以根据需求选择合适的库来实现GPU运算。
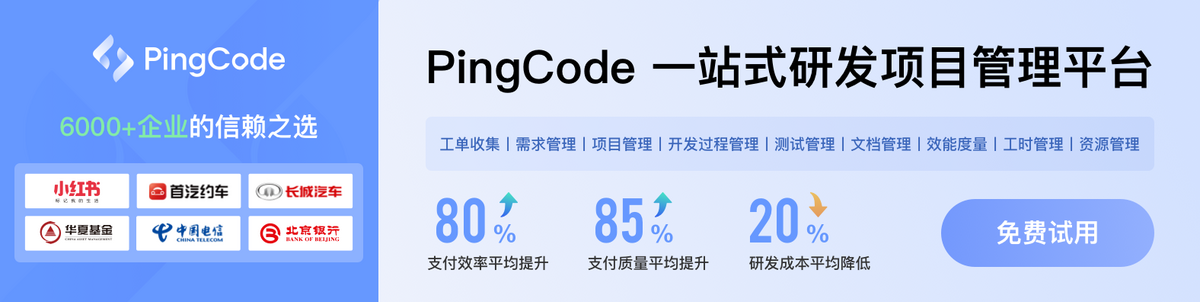