使用Python进行图像识别主要涉及使用图像处理库和深度学习框架,如OpenCV、Pillow、TensorFlow和PyTorch等。通过这些库,可以实现图像预处理、特征提取和分类等操作。以下是详细步骤和方法:
导入必要的库、加载和预处理图像、构建并训练深度学习模型、使用模型进行预测。 在这些步骤中,图像预处理和模型的选择与优化是关键,下面将详细介绍这些步骤。
一、导入必要的库
在进行图像识别之前,首先需要导入一些必要的Python库。常见的库包括OpenCV、Pillow、NumPy、TensorFlow和Keras。
import cv2
import numpy as np
from PIL import Image
import tensorflow as tf
from tensorflow.keras.models import Sequential
from tensorflow.keras.layers import Dense, Conv2D, Flatten, MaxPooling2D
from tensorflow.keras.preprocessing.image import ImageDataGenerator
二、加载和预处理图像
图像预处理是图像识别过程中非常重要的一步。常见的预处理操作包括图像缩放、归一化、灰度化和数据增强等。
1. 图像加载和缩放
使用OpenCV或Pillow库加载图像并进行缩放。
# 使用OpenCV加载图像
image = cv2.imread('path_to_image.jpg')
image = cv2.resize(image, (128, 128))
使用Pillow加载图像
image = Image.open('path_to_image.jpg')
image = image.resize((128, 128))
image = np.array(image)
2. 图像归一化
将图像像素值归一化到0到1之间,以便更好地训练深度学习模型。
image = image / 255.0
3. 数据增强
使用ImageDataGenerator进行数据增强,增加训练数据的多样性,提高模型的泛化能力。
datagen = ImageDataGenerator(
rotation_range=20,
width_shift_range=0.2,
height_shift_range=0.2,
shear_range=0.2,
zoom_range=0.2,
horizontal_flip=True,
fill_mode='nearest'
)
datagen.fit(image)
三、构建并训练深度学习模型
使用TensorFlow和Keras构建一个卷积神经网络(CNN)模型,并对其进行训练。
1. 构建模型
model = Sequential([
Conv2D(32, (3, 3), activation='relu', input_shape=(128, 128, 3)),
MaxPooling2D((2, 2)),
Conv2D(64, (3, 3), activation='relu'),
MaxPooling2D((2, 2)),
Conv2D(128, (3, 3), activation='relu'),
MaxPooling2D((2, 2)),
Flatten(),
Dense(512, activation='relu'),
Dense(10, activation='softmax') # 假设有10个类别
])
2. 编译模型
选择适当的优化器、损失函数和评估指标。
model.compile(optimizer='adam',
loss='sparse_categorical_crossentropy',
metrics=['accuracy'])
3. 训练模型
使用训练数据对模型进行训练。
model.fit(datagen.flow(train_images, train_labels, batch_size=32),
epochs=50,
validation_data=(test_images, test_labels))
四、使用模型进行预测
训练完成后,可以使用模型对新图像进行预测。
# 加载并预处理新图像
new_image = cv2.imread('path_to_new_image.jpg')
new_image = cv2.resize(new_image, (128, 128))
new_image = new_image / 255.0
new_image = np.expand_dims(new_image, axis=0)
进行预测
predictions = model.predict(new_image)
predicted_class = np.argmax(predictions)
print(f"Predicted class: {predicted_class}")
五、模型优化与提升
1. 调整模型架构
通过添加更多的卷积层、增加或减少神经元数量、使用不同的激活函数等方式调整模型架构,以提高模型性能。
2. 使用预训练模型
使用预训练模型(如VGG16、ResNet等)进行迁移学习,可以显著提高模型的准确性和收敛速度。
from tensorflow.keras.applications import VGG16
base_model = VGG16(weights='imagenet', include_top=False, input_shape=(128, 128, 3))
model = Sequential([
base_model,
Flatten(),
Dense(512, activation='relu'),
Dense(10, activation='softmax')
])
base_model.trainable = False # 冻结预训练模型的权重
model.compile(optimizer='adam',
loss='sparse_categorical_crossentropy',
metrics=['accuracy'])
model.fit(datagen.flow(train_images, train_labels, batch_size=32),
epochs=50,
validation_data=(test_images, test_labels))
3. 超参数调优
使用网格搜索或随机搜索等方法对模型的超参数进行调优,包括学习率、批量大小、优化器等。
from sklearn.model_selection import GridSearchCV
from tensorflow.keras.wrappers.scikit_learn import KerasClassifier
def create_model(optimizer='adam', activation='relu'):
model = Sequential([
Conv2D(32, (3, 3), activation=activation, input_shape=(128, 128, 3)),
MaxPooling2D((2, 2)),
Conv2D(64, (3, 3), activation=activation),
MaxPooling2D((2, 2)),
Conv2D(128, (3, 3), activation=activation),
MaxPooling2D((2, 2)),
Flatten(),
Dense(512, activation=activation),
Dense(10, activation='softmax')
])
model.compile(optimizer=optimizer,
loss='sparse_categorical_crossentropy',
metrics=['accuracy'])
return model
model = KerasClassifier(build_fn=create_model, epochs=50, batch_size=32, verbose=0)
param_grid = {
'optimizer': ['adam', 'sgd'],
'activation': ['relu', 'tanh'],
'batch_size': [32, 64],
'epochs': [50, 100]
}
grid = GridSearchCV(estimator=model, param_grid=param_grid, n_jobs=-1, cv=3)
grid_result = grid.fit(train_images, train_labels)
print(f"Best: {grid_result.best_score_} using {grid_result.best_params_}")
六、总结
使用Python进行图像识别涉及多个步骤,包括导入必要的库、加载和预处理图像、构建并训练深度学习模型以及使用模型进行预测。此外,通过调整模型架构、使用预训练模型和进行超参数调优,可以进一步提升模型的性能。在实际应用中,选择合适的图像处理和深度学习技术是成功实现图像识别的关键。希望这篇文章能为您在Python图像识别方面的实践提供有价值的参考。
相关问答FAQs:
如何选择合适的图像识别库?
在Python中,有多种库可以用于图像识别,如OpenCV、TensorFlow、Keras和PyTorch等。选择合适的库取决于你的项目需求。例如,如果你需要快速处理和分析图像,OpenCV是一个不错的选择;而如果你的目标是构建深度学习模型,TensorFlow或PyTorch会更适合。
图像识别的基本流程是什么?
图像识别的基本流程通常包括图像预处理、特征提取、模型训练和预测。在预处理阶段,常常需要对图像进行缩放、裁剪、去噪等操作。特征提取则是从图像中提取有意义的信息,通常使用卷积神经网络(CNN)来完成。接下来,使用标记好的数据训练模型,最后用训练好的模型对新图像进行分类或识别。
如何提高图像识别的准确率?
提高图像识别准确率的方法有很多,包括数据增强、使用更复杂的模型、调整超参数以及进行迁移学习。数据增强可以通过旋转、翻转、缩放等方式增加训练数据的多样性。选择合适的模型架构和优化算法,并进行超参数调整,可以提升模型的性能。此外,迁移学习可以利用已有的预训练模型,加速训练过程并提高准确率。
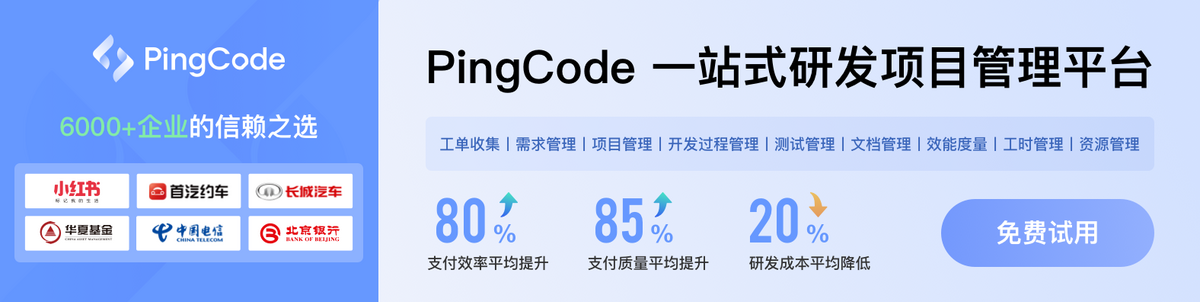