Python使用别人训练好的模型有以下几个步骤:加载模型、预处理数据、进行预测、后处理结果。其中,加载模型是最关键的一步,因为不同的框架有不同的加载方式。在这篇文章中,我们将深入探讨如何在Python中使用别人训练好的模型,包括详细的代码示例和注意事项。
一、加载模型
加载模型是使用别人训练好的模型的第一步。模型可以以多种格式保存,如HDF5、Pickle、ONNX等。根据不同的框架,加载方法也有所不同。
1.1 TensorFlow/Keras模型加载
TensorFlow和Keras是最流行的深度学习框架之一。通常情况下,模型以HDF5格式保存。
import tensorflow as tf
加载模型
model = tf.keras.models.load_model('path_to_model.h5')
1.2 PyTorch模型加载
PyTorch也是一个非常流行的深度学习框架,模型通常以.pth或.pt格式保存。
import torch
定义模型结构
model = MyModel()
加载模型参数
model.load_state_dict(torch.load('path_to_model.pth'))
切换到评估模式
model.eval()
1.3 Scikit-learn模型加载
Scikit-learn是一个用于机器学习的Python库,模型通常以Pickle格式保存。
import pickle
加载模型
with open('path_to_model.pkl', 'rb') as file:
model = pickle.load(file)
二、预处理数据
在进行预测之前,输入数据需要进行预处理。预处理步骤取决于模型的要求和输入数据的格式。常见的预处理步骤包括归一化、标准化、特征提取等。
2.1 归一化
归一化是将数据缩放到一个特定的范围,通常是[0, 1]。
from sklearn.preprocessing import MinMaxScaler
scaler = MinMaxScaler()
data_normalized = scaler.fit_transform(data)
2.2 标准化
标准化是将数据转换为均值为0,标准差为1的分布。
from sklearn.preprocessing import StandardScaler
scaler = StandardScaler()
data_standardized = scaler.fit_transform(data)
2.3 特征提取
特征提取是从数据中提取有用的特征,以便模型更好地理解数据。
from sklearn.feature_extraction.text import TfidfVectorizer
vectorizer = TfidfVectorizer()
data_vectorized = vectorizer.fit_transform(data)
三、进行预测
加载模型和预处理数据后,就可以进行预测了。预测方法因模型和框架而异。
3.1 TensorFlow/Keras预测
# 进行预测
predictions = model.predict(data_normalized)
3.2 PyTorch预测
# 将数据转换为Tensor
data_tensor = torch.tensor(data_normalized, dtype=torch.float32)
进行预测
with torch.no_grad():
predictions = model(data_tensor)
3.3 Scikit-learn预测
# 进行预测
predictions = model.predict(data_vectorized)
四、后处理结果
预测结果通常需要后处理才能得到有用的信息。后处理步骤包括反归一化、解码、计算性能指标等。
4.1 反归一化
如果数据在预处理中进行了归一化,预测结果也需要反归一化。
predictions_original = scaler.inverse_transform(predictions)
4.2 解码
如果预测结果是编码形式,需要解码为原始形式。
from sklearn.preprocessing import LabelEncoder
encoder = LabelEncoder()
predictions_decoded = encoder.inverse_transform(predictions)
4.3 计算性能指标
计算性能指标是评估模型性能的重要步骤。常见的性能指标有准确率、精确率、召回率、F1得分等。
from sklearn.metrics import accuracy_score, precision_score, recall_score, f1_score
accuracy = accuracy_score(y_true, predictions)
precision = precision_score(y_true, predictions, average='weighted')
recall = recall_score(y_true, predictions, average='weighted')
f1 = f1_score(y_true, predictions, average='weighted')
五、注意事项
5.1 模型兼容性
确保加载的模型与框架版本兼容。例如,TensorFlow 1.x和TensorFlow 2.x之间存在较大的不兼容性。
5.2 数据格式
确保输入数据的格式与模型训练时的数据格式一致。例如,图像数据通常需要调整大小和形状。
5.3 环境依赖
确保运行环境与模型训练时的环境一致。例如,某些模型可能依赖特定版本的库。
5.4 安全性
从不可信来源加载模型时要谨慎,可能存在安全风险。建议验证模型的来源和完整性。
六、实例演示
6.1 使用TensorFlow/Keras加载和预测
import tensorflow as tf
import numpy as np
加载模型
model = tf.keras.models.load_model('path_to_model.h5')
创建示例数据
data = np.random.rand(1, 28, 28, 1)
进行预测
predictions = model.predict(data)
print(predictions)
6.2 使用PyTorch加载和预测
import torch
import numpy as np
定义模型结构
class MyModel(torch.nn.Module):
def __init__(self):
super(MyModel, self).__init__()
self.layer = torch.nn.Linear(10, 1)
def forward(self, x):
return self.layer(x)
加载模型参数
model = MyModel()
model.load_state_dict(torch.load('path_to_model.pth'))
model.eval()
创建示例数据
data = np.random.rand(1, 10)
data_tensor = torch.tensor(data, dtype=torch.float32)
进行预测
with torch.no_grad():
predictions = model(data_tensor)
print(predictions)
6.3 使用Scikit-learn加载和预测
import pickle
import numpy as np
加载模型
with open('path_to_model.pkl', 'rb') as file:
model = pickle.load(file)
创建示例数据
data = np.random.rand(10, 5)
进行预测
predictions = model.predict(data)
print(predictions)
通过本文的详细介绍,相信您已经掌握了在Python中使用别人训练好的模型的基本步骤和注意事项。无论是TensorFlow/Keras、PyTorch还是Scikit-learn,只要按照本文的方法进行操作,就能顺利地加载和使用别人训练好的模型进行预测。
相关问答FAQs:
如何找到可以使用的训练好的模型?
在Python中,可以通过多个平台和库找到训练好的模型。常见的资源包括TensorFlow Hub、PyTorch Hub以及Kaggle等数据科学社区。这些平台提供了各种预训练模型,用户可以根据自己的需求进行下载和使用。此外,GitHub上也有许多开源项目分享了训练好的模型,用户可以搜索相关的关键词来找到适合的模型。
使用训练好的模型需要哪些依赖库?
使用别人训练好的模型通常需要特定的深度学习框架,如TensorFlow或PyTorch。确保安装相应版本的库,以及模型所需的任何其他依赖项。可以查看模型的文档,了解需要安装哪些额外的库和工具,这将有助于避免在使用过程中出现兼容性问题。
如何在Python中加载和使用预训练模型?
加载和使用预训练模型的过程因框架而异。在TensorFlow中,通常使用tf.keras.models.load_model()
函数来加载模型,而在PyTorch中,可以使用torch.load()
函数。加载模型后,可以使用它进行预测、微调或进一步训练。具体的代码实现可以参考模型提供的文档或示例,以确保正确使用模型的功能。
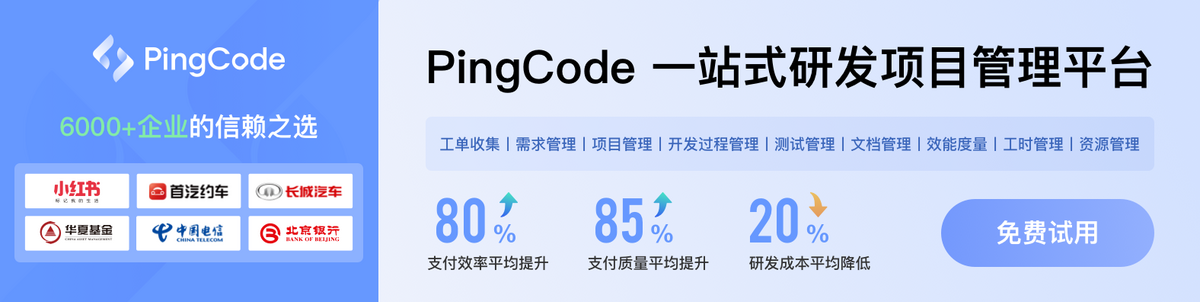