Python处理验证码问题的方法主要包括:使用OCR技术识别验证码、绕过验证码、请求获取验证码并模拟输入、使用打码平台。 在这四种方法中,使用OCR技术识别验证码是较为常见的一种。OCR(Optical Character Recognition)技术可以将图片中的文字提取出来,处理验证码时,通常会先下载验证码图片,然后利用OCR技术对其进行识别,提取出其中的文字。下面我们详细探讨如何使用Python处理验证码问题。
一、使用OCR技术识别验证码
OCR技术在处理验证码时,通常会遇到一些挑战,比如验证码的复杂性、干扰线、噪点等。为了提高识别率,我们可以对验证码图片进行预处理。
1. 安装必要的库
首先,我们需要安装一些必要的库,如pytesseract、Pillow等。
pip install pytesseract Pillow
2. 下载并配置Tesseract-OCR
pytesseract是Python的Tesseract-OCR的封装。我们需要下载并安装Tesseract-OCR,并配置环境变量。安装完成后,可以使用以下代码验证安装:
import pytesseract
from PIL import Image
验证安装
print(pytesseract.image_to_string(Image.open('test.png')))
3. 预处理验证码图片
预处理验证码图片可以提高识别率,常见的预处理方法包括灰度化、二值化、中值滤波等。
from PIL import Image
import pytesseract
import cv2
import numpy as np
def preprocess_image(image_path):
# 读取图片
image = cv2.imread(image_path)
# 转为灰度图
gray = cv2.cvtColor(image, cv2.COLOR_BGR2GRAY)
# 二值化处理
_, binary = cv2.threshold(gray, 127, 255, cv2.THRESH_BINARY)
# 中值滤波去噪
denoised = cv2.medianBlur(binary, 3)
return denoised
def recognize_captcha(image_path):
# 预处理图片
processed_image = preprocess_image(image_path)
# 使用pytesseract识别
text = pytesseract.image_to_string(processed_image, config='--psm 7')
return text
示例
captcha_text = recognize_captcha('captcha.png')
print(f'识别结果: {captcha_text}')
二、绕过验证码
绕过验证码的方法有很多种,最常见的是通过分析网页请求,找到无需验证码的接口,或者通过Session保持登录态,避免重复输入验证码。
1. 分析网页请求
使用浏览器的开发者工具,分析验证码请求和表单提交的请求,找到无需验证码的接口。
2. 使用Session保持登录态
import requests
创建Session
session = requests.Session()
获取验证码图片
captcha_url = 'https://example.com/captcha'
response = session.get(captcha_url)
with open('captcha.png', 'wb') as f:
f.write(response.content)
识别验证码
captcha_text = recognize_captcha('captcha.png')
模拟登录
login_url = 'https://example.com/login'
data = {
'username': 'your_username',
'password': 'your_password',
'captcha': captcha_text
}
response = session.post(login_url, data=data)
检查登录状态
if '登录成功' in response.text:
print('登录成功')
else:
print('登录失败')
三、请求获取验证码并模拟输入
在某些情况下,我们需要请求获取验证码,并将识别结果模拟输入提交表单。
1. 获取验证码
import requests
def get_captcha(session, url):
response = session.get(url)
with open('captcha.png', 'wb') as f:
f.write(response.content)
示例
session = requests.Session()
captcha_url = 'https://example.com/captcha'
get_captcha(session, captcha_url)
2. 模拟输入并提交表单
def submit_form(session, url, data):
response = session.post(url, data=data)
return response
示例
login_url = 'https://example.com/login'
captcha_text = recognize_captcha('captcha.png')
data = {
'username': 'your_username',
'password': 'your_password',
'captcha': captcha_text
}
response = submit_form(session, login_url, data)
检查提交结果
if '登录成功' in response.text:
print('登录成功')
else:
print('登录失败')
四、使用打码平台
打码平台是一些提供验证码识别服务的第三方平台,我们可以调用其API来识别验证码。
1. 注册并获取API Key
首先需要在打码平台注册账号,并获取API Key。
2. 调用API识别验证码
import requests
def recognize_captcha_with_api(api_key, image_path):
with open(image_path, 'rb') as f:
files = {'file': f}
data = {'apikey': api_key}
response = requests.post('https://api.example.com/recognize', files=files, data=data)
result = response.json()
return result['text']
示例
api_key = 'your_api_key'
captcha_text = recognize_captcha_with_api(api_key, 'captcha.png')
print(f'识别结果: {captcha_text}')
五、验证码识别的挑战与解决方案
1. 复杂验证码
对于一些复杂的验证码,可能需要结合多种预处理方法,甚至使用深度学习模型来提高识别率。
2. 干扰线与噪点
对于含有干扰线与噪点的验证码,可以使用图像处理方法,如形态学操作、去噪算法等,进行预处理。
3. 动态验证码
对于动态验证码,可以使用帧提取技术,将每一帧保存并识别,最后合并识别结果。
import cv2
def extract_frames(video_path):
cap = cv2.VideoCapture(video_path)
frames = []
while cap.isOpened():
ret, frame = cap.read()
if not ret:
break
frames.append(frame)
cap.release()
return frames
示例
frames = extract_frames('captcha.mp4')
for i, frame in enumerate(frames):
cv2.imwrite(f'frame_{i}.png', frame)
六、深度学习与验证码识别
深度学习模型在验证码识别中表现出色,尤其是卷积神经网络(CNN)。我们可以使用Keras或PyTorch等深度学习框架,训练一个验证码识别模型。
1. 数据集准备
首先需要准备大量的验证码图片及其对应的标签,用于训练模型。
2. 模型训练
import keras
from keras.models import Sequential
from keras.layers import Conv2D, MaxPooling2D, Flatten, Dense
def build_model(input_shape, num_classes):
model = Sequential()
model.add(Conv2D(32, (3, 3), activation='relu', input_shape=input_shape))
model.add(MaxPooling2D((2, 2)))
model.add(Conv2D(64, (3, 3), activation='relu'))
model.add(MaxPooling2D((2, 2)))
model.add(Flatten())
model.add(Dense(128, activation='relu'))
model.add(Dense(num_classes, activation='softmax'))
return model
示例
input_shape = (50, 200, 1)
num_classes = 36
model = build_model(input_shape, num_classes)
model.compile(optimizer='adam', loss='categorical_crossentropy', metrics=['accuracy'])
model.summary()
3. 模型训练与评估
from keras.preprocessing.image import ImageDataGenerator
def train_model(model, train_data, train_labels, val_data, val_labels, batch_size, epochs):
datagen = ImageDataGenerator(rescale=1./255, validation_split=0.2)
train_generator = datagen.flow(train_data, train_labels, batch_size=batch_size, subset='training')
validation_generator = datagen.flow(val_data, val_labels, batch_size=batch_size, subset='validation')
history = model.fit(train_generator, epochs=epochs, validation_data=validation_generator)
return history
示例
train_data, train_labels, val_data, val_labels = load_data() # 自定义数据加载函数
history = train_model(model, train_data, train_labels, val_data, val_labels, batch_size=32, epochs=10)
4. 模型评估与预测
def evaluate_model(model, test_data, test_labels):
test_generator = ImageDataGenerator(rescale=1./255).flow(test_data, test_labels)
loss, accuracy = model.evaluate(test_generator)
return loss, accuracy
def predict_captcha(model, image):
image = image / 255.0
image = image.reshape(1, *image.shape)
prediction = model.predict(image)
return prediction.argmax(axis=-1)
示例
test_data, test_labels = load_test_data() # 自定义数据加载函数
loss, accuracy = evaluate_model(model, test_data, test_labels)
print(f'测试损失: {loss}, 测试准确率: {accuracy}')
captcha_image = preprocess_image('captcha.png')
predicted_label = predict_captcha(model, captcha_image)
print(f'预测结果: {predicted_label}')
七、总结
Python处理验证码问题的方法多种多样,从简单的OCR识别到复杂的深度学习模型,每种方法都有其适用场景和挑战。使用OCR技术识别验证码、绕过验证码、请求获取验证码并模拟输入、使用打码平台是常见的处理方法。在实际应用中,我们可以根据具体需求选择合适的方法,同时结合多种技术手段,提高验证码识别的准确率和效率。无论选择哪种方法,都需要充分理解验证码的特性和挑战,灵活运用图像处理、机器学习等技术,实现高效的验证码处理。
相关问答FAQs:
如何在Python中识别图像验证码?
识别图像验证码通常可以通过使用OCR(光学字符识别)库来实现。Tesseract是一个流行的OCR工具,可以与Python结合使用。可以通过Pillow库处理图像,调整对比度和清晰度,以提高识别准确率。安装相关库后,可以加载验证码图像并使用Tesseract进行识别。
使用Python处理动态验证码的最佳方法是什么?
动态验证码通常涉及时间限制或用户交互。这类验证码的处理较为复杂,可能需要自动化浏览器工具如Selenium,模拟用户行为以获取验证码。在获取验证码后,可以使用OCR技术进行识别,或者通过API请求获取验证码的正确答案。
如何防止验证码被自动识别?
为了保护验证码免受自动识别,开发者可以采取多种措施。例如,添加干扰线、使用复杂的字体、改变背景颜色等。此外,适当的使用时间限制、IP限制和用户行为分析也可以增强验证码的安全性,降低被自动识别的风险。
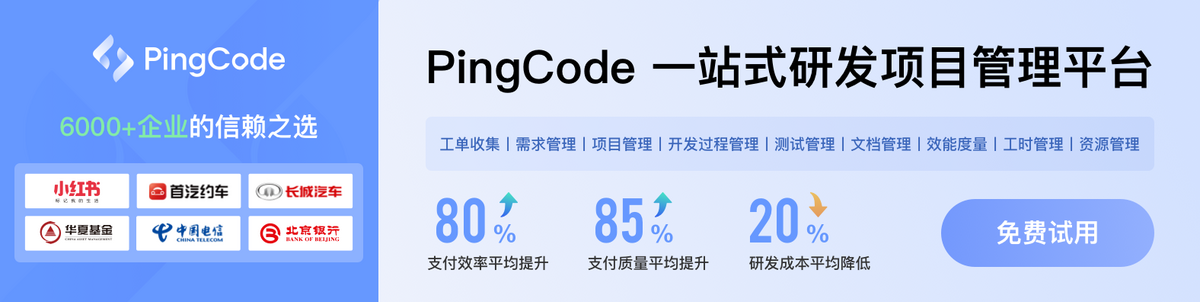