Python中应用随机森林训练数据的方法包括:导入必要的库、准备数据、分割数据集、初始化和训练模型、评估模型性能、调优参数和可视化结果。 其中,数据的准备和模型的初始化与训练尤为关键。随机森林是一种集成学习方法,通过构建多个决策树并结合其结果来提高预测的准确性和稳定性。接下来,我们将详细介绍在Python中如何应用随机森林训练数据。
一、导入必要的库
在开始使用随机森林之前,我们需要导入一些必要的Python库。主要的库包括pandas
、numpy
、scikit-learn
等。pandas
用于数据操作和分析,numpy
用于数值计算,而scikit-learn
则提供了随机森林模型和其他机器学习工具。
import pandas as pd
import numpy as np
from sklearn.model_selection import train_test_split
from sklearn.ensemble import RandomForestClassifier
from sklearn.metrics import accuracy_score, confusion_matrix, classification_report
二、准备数据
在实际应用中,我们通常会使用一个数据集来训练和评估模型。首先,我们需要加载数据集,并进行必要的预处理,如处理缺失值、编码分类变量等。
# 读取数据
data = pd.read_csv('path/to/your/data.csv')
查看数据基本信息
print(data.head())
print(data.info())
处理缺失值
data.fillna(data.mean(), inplace=True)
编码分类变量
data = pd.get_dummies(data, drop_first=True)
三、分割数据集
为了评估模型的性能,我们通常将数据集分为训练集和测试集。训练集用于训练模型,而测试集用于评估模型的泛化能力。
# 定义特征和目标变量
X = data.drop('target', axis=1)
y = data['target']
分割数据集
X_train, X_test, y_train, y_test = train_test_split(X, y, test_size=0.3, random_state=42)
四、初始化和训练模型
接下来,我们将初始化随机森林模型,并使用训练集进行训练。RandomForestClassifier
是scikit-learn
中提供的用于分类任务的随机森林模型。
# 初始化随机森林模型
rf_model = RandomForestClassifier(n_estimators=100, random_state=42)
训练模型
rf_model.fit(X_train, y_train)
五、评估模型性能
训练完成后,我们需要评估模型在测试集上的表现。常用的评估指标包括准确率、混淆矩阵和分类报告。
# 预测测试集
y_pred = rf_model.predict(X_test)
计算准确率
accuracy = accuracy_score(y_test, y_pred)
print(f'Accuracy: {accuracy:.2f}')
混淆矩阵
conf_matrix = confusion_matrix(y_test, y_pred)
print('Confusion Matrix:')
print(conf_matrix)
分类报告
class_report = classification_report(y_test, y_pred)
print('Classification Report:')
print(class_report)
六、调优参数
为了进一步提高模型的性能,我们可以调优随机森林的参数。常见的调优参数包括n_estimators
(树的数量)、max_depth
(树的最大深度)等。我们可以使用网格搜索(Grid Search)或随机搜索(Random Search)来自动化参数调优。
from sklearn.model_selection import GridSearchCV
定义参数网格
param_grid = {
'n_estimators': [50, 100, 200],
'max_depth': [None, 10, 20, 30],
'min_samples_split': [2, 5, 10],
'min_samples_leaf': [1, 2, 4]
}
初始化网格搜索
grid_search = GridSearchCV(estimator=rf_model, param_grid=param_grid, cv=3, n_jobs=-1, verbose=2)
进行网格搜索
grid_search.fit(X_train, y_train)
输出最佳参数
print(f'Best Parameters: {grid_search.best_params_}')
七、可视化结果
为了更直观地理解模型的表现,我们可以使用一些可视化工具。常见的可视化方法包括特征重要性图、ROC曲线等。
import matplotlib.pyplot as plt
import seaborn as sns
from sklearn.metrics import roc_curve, auc
特征重要性
feature_importances = rf_model.feature_importances_
features = X.columns
indices = np.argsort(feature_importances)[::-1]
plt.figure(figsize=(10, 6))
plt.title("Feature Importance")
plt.bar(range(X.shape[1]), feature_importances[indices], align="center")
plt.xticks(range(X.shape[1]), features[indices], rotation=90)
plt.tight_layout()
plt.show()
ROC曲线
fpr, tpr, _ = roc_curve(y_test, rf_model.predict_proba(X_test)[:, 1])
roc_auc = auc(fpr, tpr)
plt.figure(figsize=(8, 6))
plt.plot(fpr, tpr, color='darkorange', lw=2, label=f'ROC curve (area = {roc_auc:.2f})')
plt.plot([0, 1], [0, 1], color='navy', lw=2, linestyle='--')
plt.xlim([0.0, 1.0])
plt.ylim([0.0, 1.05])
plt.xlabel('False Positive Rate')
plt.ylabel('True Positive Rate')
plt.title('Receiver Operating Characteristic (ROC)')
plt.legend(loc="lower right")
plt.tight_layout()
plt.show()
通过以上步骤,我们可以在Python中应用随机森林来训练数据,并对模型的性能进行评估和优化。随机森林作为一种强大的集成学习方法,能够有效地处理高维数据和复杂的非线性关系,广泛应用于分类和回归任务。
相关问答FAQs:
如何在Python中实现随机森林算法?
在Python中,可以通过使用scikit-learn
库来实现随机森林算法。首先,确保你已经安装了这个库。使用RandomForestClassifier
或RandomForestRegressor
类来创建模型。接下来,加载数据集,进行数据预处理,然后使用fit
方法训练模型,最后利用predict
方法进行预测。
随机森林模型的优缺点是什么?
随机森林是一种强大的集成学习方法,具有许多优点,比如处理高维数据的能力强、对缺失值的鲁棒性好、模型的可解释性较强。然而,它也有一些缺点,如模型较大、训练时间较长、在实时预测中可能不够灵活,此外,在某些情况下,可能会过拟合。
如何评估随机森林模型的性能?
可以通过多种方式评估随机森林模型的性能。常见的评估指标包括准确率、精确率、召回率和F1分数等。使用cross_val_score
函数可以进行交叉验证,确保模型的稳健性。此外,可以通过绘制ROC曲线或混淆矩阵来直观了解模型的分类效果。
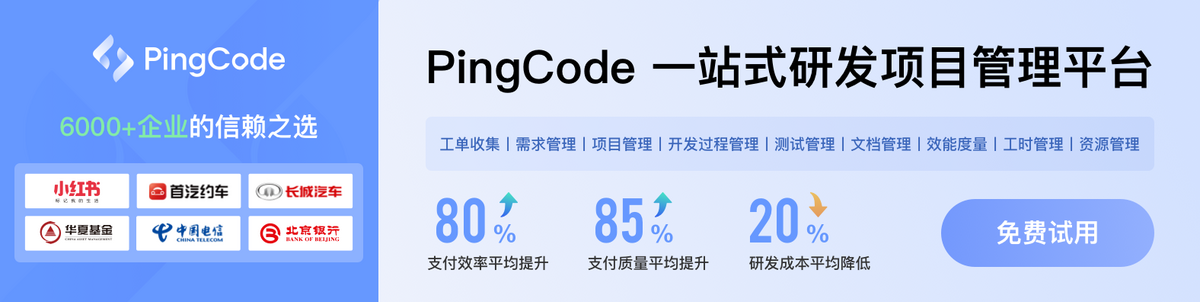