Python对比两个点云的方法包括:使用KD树、计算Hausdorff距离、使用ICP算法。 其中,使用ICP(Iterative Closest Point)算法是最常用和详细的方法。ICP算法通过迭代的方法,将两组点云对齐,使它们之间的距离最小化。这个过程包括最近邻匹配、变换计算和误差评估。下面将详细介绍如何使用ICP算法对比两个点云。
一、使用KD树
KD树(K-Dimensional Tree)是一种数据结构,用于在K维空间中对点进行组织和查询。它可以用来快速找到点云中的最近邻点,从而计算两组点云之间的距离。
1、构建KD树
KD树的构建是递归的,将点云划分成左右子树,每次选择一个维度进行划分。可以使用SciPy库中的KDTree类来构建KD树。
import numpy as np
from scipy.spatial import KDTree
生成随机点云数据
points1 = np.random.rand(100, 3)
points2 = np.random.rand(100, 3)
构建KD树
tree1 = KDTree(points1)
tree2 = KDTree(points2)
2、查找最近邻点
使用KD树查找最近邻点,可以快速找到点云中与给定点最接近的点。
# 查找最近邻点
distances, indices = tree1.query(points2)
print("最近邻距离:", distances)
print("最近邻索引:", indices)
3、计算距离
通过查找最近邻点,可以计算两组点云之间的距离。可以使用均方误差(MSE)或其他距离度量方法。
# 计算均方误差
mse = np.mean(distances2)
print("均方误差:", mse)
二、计算Hausdorff距离
Hausdorff距离是一种衡量两组点集之间相似性的度量,定义为集合A中每个点到集合B中最近点的最大距离。它可以用来比较两组点云的相似性。
1、计算Hausdorff距离
可以使用SciPy库中的directed_hausdorff函数来计算Hausdorff距离。
from scipy.spatial.distance import directed_hausdorff
计算Hausdorff距离
hausdorff_distance = directed_hausdorff(points1, points2)[0]
print("Hausdorff距离:", hausdorff_distance)
三、使用ICP算法
ICP(Iterative Closest Point)算法是一种迭代优化算法,用于将两组点云对齐,使它们之间的距离最小化。它是点云对比的常用方法。
1、安装PCL库
PCL(Point Cloud Library)是一个流行的点云处理库,可以使用python-pcl来调用PCL库中的ICP算法。
pip install python-pcl
2、加载点云数据
使用PCL库加载点云数据。
import pcl
加载点云数据
cloud1 = pcl.load('cloud1.pcd')
cloud2 = pcl.load('cloud2.pcd')
3、执行ICP算法
使用PCL库中的ICP类执行ICP算法,将两组点云对齐。
# 创建ICP对象
icp = cloud1.make_IterativeClosestPoint()
icp.set_MaxCorrespondenceDistance(0.05)
icp.set_MaxIterations(50)
icp.set_TransformationEpsilon(1e-8)
icp.set_EuclideanFitnessEpsilon(1)
执行ICP算法
converged, transf, estimate, fitness = icp.icp(cloud2)
print('是否收敛:', converged)
print('变换矩阵:\n', transf)
print('估计点云:\n', estimate)
print('拟合度:', fitness)
四、其他方法
除了KD树、Hausdorff距离和ICP算法,还有其他一些方法可以用于对比点云。
1、使用Open3D库
Open3D是一个开源的3D数据处理库,提供了丰富的点云处理功能,包括ICP算法。
import open3d as o3d
加载点云数据
pcd1 = o3d.io.read_point_cloud('cloud1.ply')
pcd2 = o3d.io.read_point_cloud('cloud2.ply')
执行ICP算法
threshold = 0.02
trans_init = np.eye(4)
reg_p2p = o3d.pipelines.registration.registration_icp(
pcd2, pcd1, threshold, trans_init,
o3d.pipelines.registration.TransformationEstimationPointToPoint())
print('变换矩阵:\n', reg_p2p.transformation)
2、使用Farthest Point Sampling(FPS)
FPS是一种点云简化方法,通过选择距离最远的点来简化点云。可以使用FPS来对比简化后的点云。
def farthest_point_sampling(points, k):
n, d = points.shape
centroids = np.zeros((k, d))
distances = np.full(n, np.inf)
farthest = np.random.randint(0, n)
for i in range(k):
centroids[i] = points[farthest]
dist = np.linalg.norm(points - centroids[i], axis=1)
distances = np.minimum(distances, dist)
farthest = np.argmax(distances)
return centroids
采样点云
sampled_points1 = farthest_point_sampling(points1, 50)
sampled_points2 = farthest_point_sampling(points2, 50)
通过以上方法,可以在Python中对比两个点云。根据具体需求,可以选择不同的方法进行点云对比。
相关问答FAQs:
如何在Python中加载和处理点云数据?
在Python中,可以使用open3d
、PCL
(Point Cloud Library)或numpy
库来加载和处理点云数据。open3d
库提供了简单易用的接口,可以轻松读取不同格式的点云文件,例如PLY或PCD格式。加载数据后,可以使用各种方法对点云进行预处理,比如去噪、下采样和法线估计,以便后续进行对比分析。
点云对比的常用算法有哪些?
在Python中,对比两个点云的常用算法包括ICP(Iterative Closest Point)、FFD(Free-Form Deformation)和Hausdorff距离等。ICP算法通过迭代优化来对齐两个点云,非常适合在初始位置接近的情况下使用。而Hausdorff距离则可以用来量化两个点云之间的最大距离差异,适用于评估点云的整体相似度。
如何可视化对比结果以便更好地理解点云之间的差异?
使用matplotlib
或open3d
库,可以将对比结果可视化。在open3d
中,可以通过draw_geometries
函数直接展示点云,还可以使用不同的颜色标记出相似和不同的区域。此外,利用matplotlib
的3D绘图功能,能够更直观地展示点云的空间分布及其差异,帮助分析和理解两个点云之间的关系。
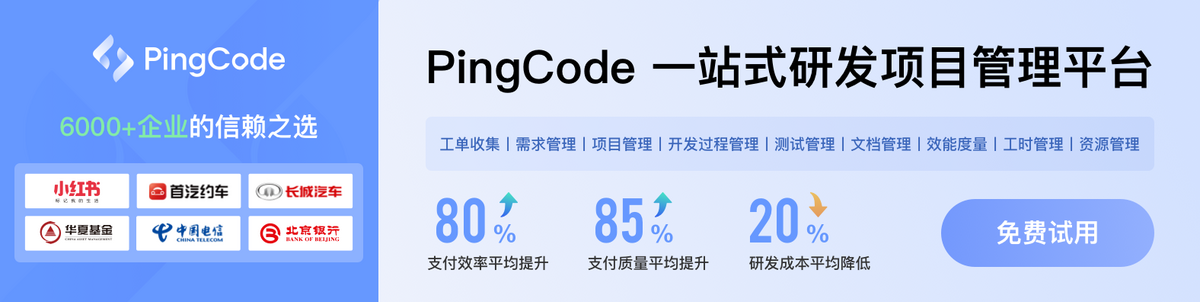