使用Python编写搜索引擎的核心步骤包括:数据收集、数据处理和存储、索引构建、查询处理和排名算法。 本文将详细介绍这些步骤,并提供相应的代码示例和技术细节。
一、数据收集
数据收集是搜索引擎的基础。我们需要从互联网上抓取网页内容,然后存储在本地数据库或文件系统中。Python的requests
库和BeautifulSoup
库是常用的网页抓取工具。
import requests
from bs4 import BeautifulSoup
def fetch_webpage(url):
try:
response = requests.get(url)
if response.status_code == 200:
return response.text
else:
print(f"Failed to fetch webpage: {url}")
return None
except Exception as e:
print(f"Error fetching webpage: {url}, {e}")
return None
def parse_html(html):
soup = BeautifulSoup(html, 'html.parser')
return soup.get_text()
url = 'https://example.com'
html_content = fetch_webpage(url)
if html_content:
page_text = parse_html(html_content)
print(page_text)
二、数据处理和存储
抓取的数据需要进行清洗和预处理,如去除HTML标签、去除停用词、词形还原等。预处理后的数据可以存储在数据库中,如SQLite、MongoDB等。
import re
import sqlite3
from nltk.corpus import stopwords
from nltk.stem import WordNetLemmatizer
def clean_text(text):
# 去除HTML标签
text = re.sub(r'<[^>]+>', '', text)
# 去除非字母字符
text = re.sub(r'[^a-zA-Z\s]', '', text)
# 转换为小写
text = text.lower()
# 去除停用词
stop_words = set(stopwords.words('english'))
words = text.split()
words = [word for word in words if word not in stop_words]
# 词形还原
lemmatizer = WordNetLemmatizer()
words = [lemmatizer.lemmatize(word) for word in words]
return ' '.join(words)
def store_data(url, text, db_conn):
cursor = db_conn.cursor()
cursor.execute("INSERT INTO webpages (url, content) VALUES (?, ?)", (url, text))
db_conn.commit()
数据库连接
conn = sqlite3.connect('search_engine.db')
conn.execute('CREATE TABLE IF NOT EXISTS webpages (id INTEGER PRIMARY KEY, url TEXT, content TEXT)')
清洗并存储数据
cleaned_text = clean_text(page_text)
store_data(url, cleaned_text, conn)
conn.close()
三、索引构建
索引是搜索引擎的核心,它将文档中的词映射到包含这些词的文档。倒排索引是一种常用的数据结构,可以高效地支持全文搜索。
from collections import defaultdict
def build_index(db_conn):
cursor = db_conn.cursor()
cursor.execute("SELECT id, content FROM webpages")
documents = cursor.fetchall()
index = defaultdict(list)
for doc_id, content in documents:
words = content.split()
for word in words:
index[word].append(doc_id)
return index
构建索引
conn = sqlite3.connect('search_engine.db')
index = build_index(conn)
conn.close()
四、查询处理
查询处理包括对用户查询进行解析、转换为内部表示形式、查找匹配的文档以及对结果进行排序。
def search(query, index, db_conn):
query_words = clean_text(query).split()
doc_scores = defaultdict(int)
for word in query_words:
if word in index:
doc_ids = index[word]
for doc_id in doc_ids:
doc_scores[doc_id] += 1
sorted_docs = sorted(doc_scores.items(), key=lambda x: x[1], reverse=True)
# 获取文档内容
cursor = db_conn.cursor()
results = []
for doc_id, score in sorted_docs:
cursor.execute("SELECT url, content FROM webpages WHERE id=?", (doc_id,))
result = cursor.fetchone()
results.append((result[0], result[1], score))
return results
处理查询
query = "example query"
conn = sqlite3.connect('search_engine.db')
results = search(query, index, conn)
for url, content, score in results:
print(f"URL: {url}, Score: {score}")
print(content[:500]) # 打印前500个字符
conn.close()
五、排名算法
排名算法决定了搜索结果的排序。常用的排名算法包括TF-IDF、BM25、PageRank等。这里我们简单介绍TF-IDF和BM25。
- TF-IDF
TF-IDF(Term Frequency-Inverse Document Frequency)是一种常用的文本检索方法。TF表示词频,IDF表示逆文档频率。TF-IDF的计算公式为:
[ \text{TF-IDF}(t, d) = \text{TF}(t, d) \times \text{IDF}(t) ]
其中:
[ \text{TF}(t, d) = \frac{\text{词语}t\text{在文档}d\text{中的出现次数}}{\text{文档}d\text{的总词数}} ]
[ \text{IDF}(t) = \log\frac{\text{文档总数}}{\text{包含词语}t\text{的文档数}} ]
- BM25
BM25是一种改进的TF-IDF算法,考虑了文档长度对词频的影响。BM25的计算公式为:
[ \text{BM25}(t, d) = \frac{\text{TF}(t, d) \times (\text{k}_1 + 1)}{\text{TF}(t, d) + \text{k}_1 \times (1 – \text{b} + \text{b} \times \frac{|d|}{\text{avgdl}})} \times \text{IDF}(t) ]
其中:
- ( \text{k}_1 ) 和 ( \text{b} ) 是可调参数
- ( |d| ) 是文档的长度
- ( \text{avgdl} ) 是文档集合的平均长度
总结
本文介绍了如何使用Python编写一个简单的搜索引擎,包括数据收集、数据处理和存储、索引构建、查询处理和排名算法。通过这些步骤,我们可以实现一个基本的全文搜索功能。当然,实际应用中,搜索引擎还需要考虑更多的因素,如分布式爬虫、数据存储和检索的效率、复杂的排名算法等。希望本文能为你提供一个基本的思路和实现方法。
相关问答FAQs:
如何开始使用Python构建一个简单的搜索引擎?
构建搜索引擎的第一步是了解其基本构成,包括爬虫、索引和查询处理。您可以使用库如Scrapy来编写爬虫,从网页中提取数据。接着,使用SQLite或其他数据库来存储和索引抓取到的信息。最后,编写一个查询处理模块,接受用户输入并从索引中返回相关结果。
有哪些Python库可以帮助我构建搜索引擎?
有许多Python库可以帮助您构建搜索引擎。Scrapy是一个强大的网页抓取框架,Beautiful Soup可以用于解析HTML文档,NLTK或spaCy则可以帮助处理和分析自然语言。Elasticsearch和Whoosh是一些用于实现搜索功能的优秀库,它们提供了强大的索引和查询能力。
在创建搜索引擎时,如何提高搜索结果的相关性?
提高搜索结果的相关性可以通过多种方式实现。首先,确保在索引过程中提取了足够的关键词和元数据。其次,可以实现TF-IDF(词频-逆文档频率)算法来评估文档的重要性。此外,考虑使用机器学习技术来分析用户行为和偏好,从而不断优化搜索结果的排名。
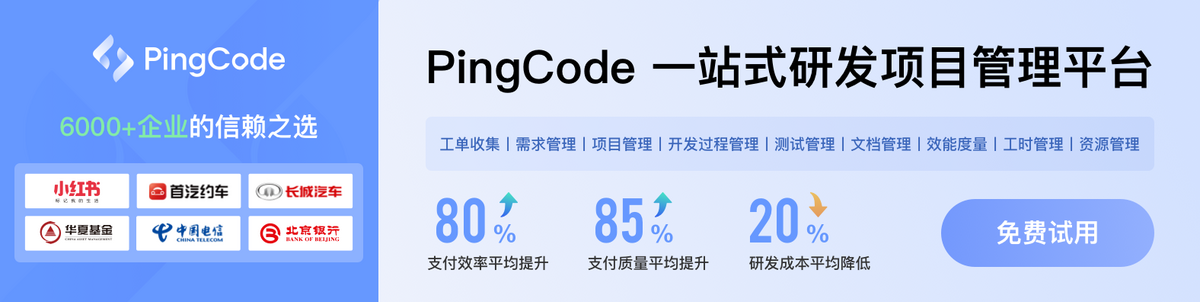