Python处理法律文本的方法包括:文本预处理、自然语言处理(NLP)工具使用、正则表达式匹配、命名实体识别(NER)、主题建模。 其中,文本预处理是所有文本处理的基础,它包括去除噪音、分词、词干提取等步骤。文本预处理是确保后续的自然语言处理任务能够顺利进行的重要步骤。
法律文本通常含有大量的专业术语、长句和复杂的结构,处理这些文本需要使用适当的方法来确保信息的准确性和完整性。下面将详细探讨如何使用Python处理法律文本的不同方法和步骤。
一、文本预处理
去除噪音
法律文本中可能包含一些无关的符号、数字和标点符号,这些都需要在预处理阶段去除。Python中可以使用正则表达式(re库)来实现这一点。
import re
def remove_noise(text):
text = re.sub(r'\d+', '', text) # 移除数字
text = re.sub(r'[^\w\s]', '', text) # 移除标点符号
text = text.lower() # 转化为小写
return text
分词
分词是将文本分割成单个单词的过程。Python的NLTK库和spaCy库都提供了强大的分词功能。
import nltk
from nltk.tokenize import word_tokenize
nltk.download('punkt')
def tokenize(text):
return word_tokenize(text)
import spacy
nlp = spacy.load("en_core_web_sm")
def tokenize(text):
doc = nlp(text)
return [token.text for token in doc]
词干提取和词形还原
词干提取是将单词还原到其词根形式,而词形还原是将单词还原到其标准形式。NLTK和spaCy同样提供了这些功能。
from nltk.stem import PorterStemmer
stemmer = PorterStemmer()
def stemming(tokens):
return [stemmer.stem(token) for token in tokens]
from nltk.stem import WordNetLemmatizer
lemmatizer = WordNetLemmatizer()
def lemmatizing(tokens):
return [lemmatizer.lemmatize(token) for token in tokens]
二、自然语言处理(NLP)工具使用
TF-IDF(词频-逆文档频率)
TF-IDF是一种常用的文本表示方法,用于评估单词在文档中的重要性。可以使用scikit-learn库来实现TF-IDF。
from sklearn.feature_extraction.text import TfidfVectorizer
def tfidf_vectorize(corpus):
vectorizer = TfidfVectorizer()
X = vectorizer.fit_transform(corpus)
return X, vectorizer
词向量(Word Embeddings)
词向量是将单词映射到一个连续的向量空间中,常用的词向量模型有Word2Vec、GloVe等。可以使用gensim库来训练或加载预训练的词向量模型。
from gensim.models import Word2Vec
def train_word2vec(sentences):
model = Word2Vec(sentences, vector_size=100, window=5, min_count=1, workers=4)
return model
三、正则表达式匹配
正则表达式是一种强大的文本匹配工具,适用于从文本中提取特定模式的信息。法律文本中常见的模式包括日期、条款编号等。
import re
def find_dates(text):
pattern = r'\b\d{4}-\d{2}-\d{2}\b' # 匹配YYYY-MM-DD格式的日期
return re.findall(pattern, text)
四、命名实体识别(NER)
命名实体识别是从文本中识别出特定实体(如人名、地名、组织名等)的过程。可以使用spaCy库来实现NER。
import spacy
nlp = spacy.load("en_core_web_sm")
def named_entity_recognition(text):
doc = nlp(text)
entities = [(ent.text, ent.label_) for ent in doc.ents]
return entities
五、主题建模
主题建模是一种无监督的机器学习方法,用于从文档集中发现隐藏的主题。常用的主题建模方法有LDA(Latent Dirichlet Allocation),可以使用gensim库来实现。
from gensim import corpora
from gensim.models.ldamodel import LdaModel
def lda_topic_modeling(texts, num_topics=5, passes=10):
dictionary = corpora.Dictionary(texts)
corpus = [dictionary.doc2bow(text) for text in texts]
lda_model = LdaModel(corpus, num_topics=num_topics, id2word=dictionary, passes=passes)
return lda_model
示例用法
以下是一个完整的示例,展示了如何使用上述方法来处理法律文本。
import spacy
from nltk.tokenize import sent_tokenize
加载法律文本
text = """
In the Supreme Court of the United States
Oct. Term, 2021
No. 20-1199
NORTH CAROLINA STATE CONFERENCE OF THE NAACP, et al., Petitioners
v.
PATRICK MCCRORY, in His Official Capacity as the Governor of North Carolina, et al.
On Writ of Certiorari to the United States Court of Appeals for the Fourth Circuit
BRIEF FOR THE UNITED STATES AS AMICUS CURIAE SUPPORTING PETITIONERS
INTEREST OF THE UNITED STATES
This case concerns the constitutionality of North Carolina’s House Bill 589 (HB 589), which imposes certain voting restrictions, including a photo identification requirement. The United States has a significant interest in the enforcement of federal voting rights laws, including the Voting Rights Act of 1965, 52 U.S.C. 10301 et seq., and in ensuring that all eligible citizens have the opportunity to participate in the political process on an equal basis.
"""
文本预处理
def preprocess_text(text):
text = remove_noise(text)
tokens = tokenize(text)
lemmas = lemmatizing(tokens)
return lemmas
分词
sentences = sent_tokenize(text)
preprocessed_texts = [preprocess_text(sent) for sent in sentences]
主题建模
lda_model = lda_topic_modeling(preprocessed_texts)
for idx, topic in lda_model.print_topics(-1):
print(f"Topic: {idx} \nWords: {topic}")
命名实体识别
entities = named_entity_recognition(text)
print(entities)
通过上述步骤,我们可以有效地处理和分析法律文本,提取有价值的信息并进行进一步的分析。Python提供了丰富的工具和库,使得处理复杂的法律文本变得更加高效和便捷。
相关问答FAQs:
如何使用Python提取法律文本中的关键信息?
Python提供了多种库和工具,如NLTK、spaCy和PyPDF2,可以帮助用户提取法律文本中的关键信息。使用这些工具,可以实现信息提取、文本摘要和关键词识别等功能。例如,利用正则表达式可以从法律文件中提取特定条款,而自然语言处理库则能帮助分析和理解文本的语义。
Python中有哪些库适合进行法律文本分析?
在进行法律文本分析时,一些常用的Python库包括NLTK(自然语言工具包)、spaCy(快速且高效的自然语言处理库)、以及PyPDF2(用于处理PDF文档的库)。这些工具能够帮助用户进行文本预处理、词性标注、实体识别和情感分析等操作,适合法律文本的深入分析。
如何处理法律文本的格式化问题?
法律文本往往包含多种格式,如PDF、Word文档或纯文本文件。使用Python中的PyPDF2和python-docx等库,可以有效地读取和处理这些不同格式的法律文件。对于格式不规范的文本,可以利用Python的字符串处理功能进行清洗和标准化,以便于后续的数据分析和处理。
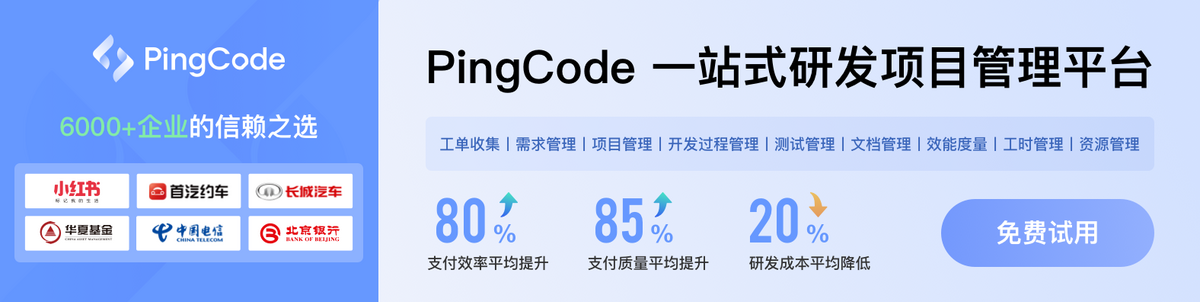