开头段落:
通过Python将用户地点转换为矩阵,可以使用地理编码API、处理地理坐标数据、构建矩阵。首先,使用地理编码API(如Google Geocoding API)将用户提供的地点转换为经纬度坐标。其次,处理这些地理坐标数据,并将其存储在数据结构中。最后,根据这些数据构建矩阵,以便进行进一步的计算和分析。下面将详细介绍如何使用Python实现这些步骤中的每一步。
一、使用地理编码API获取经纬度
在将用户地点转换为矩阵之前,首先需要将用户提供的地点(例如城市名称或具体地址)转换为经纬度坐标。为此,可以使用地理编码API,如Google Geocoding API、OpenCage Geocoder或Nominatim。
- Google Geocoding API的使用
Google Geocoding API是一个强大的工具,可以将地址转换为地理坐标。要使用它,你需要一个API密钥,并按照以下步骤进行操作:
import requests
def get_coordinates(address, api_key):
base_url = 'https://maps.googleapis.com/maps/api/geocode/json'
params = {
'address': address,
'key': api_key
}
response = requests.get(base_url, params=params)
result = response.json()
if result['status'] == 'OK':
location = result['results'][0]['geometry']['location']
return (location['lat'], location['lng'])
else:
return None
示例调用
api_key = 'YOUR_API_KEY'
address = '1600 Amphitheatre Parkway, Mountain View, CA'
coordinates = get_coordinates(address, api_key)
print(coordinates)
- 处理API响应
处理API响应,提取地理坐标,并将其存储在适当的数据结构中。例如,可以将每个用户地点及其对应的坐标存储在字典中:
user_locations = ['1600 Amphitheatre Parkway, Mountain View, CA', '1 Infinite Loop, Cupertino, CA']
location_coordinates = {}
for location in user_locations:
coordinates = get_coordinates(location, api_key)
if coordinates:
location_coordinates[location] = coordinates
print(location_coordinates)
二、处理地理坐标数据
一旦获得了用户地点的地理坐标,就可以进一步处理这些数据,以便构建矩阵。
- 将坐标转换为二维矩阵
将用户地点的地理坐标存储在二维矩阵中,每行代表一个地点,每列代表纬度或经度。例如:
import numpy as np
coordinates_matrix = np.array(list(location_coordinates.values()))
print(coordinates_matrix)
- 计算地点之间的距离
可以使用地理坐标计算地点之间的距离,例如使用Haversine公式。以下是一个示例函数,用于计算两个地理坐标之间的距离:
import math
def haversine(coord1, coord2):
lat1, lon1 = coord1
lat2, lon2 = coord2
R = 6371 # 地球半径,单位为千米
phi1 = math.radians(lat1)
phi2 = math.radians(lat2)
delta_phi = math.radians(lat2 - lat1)
delta_lambda = math.radians(lon2 - lon1)
a = math.sin(delta_phi / 2.0) 2 + \
math.cos(phi1) * math.cos(phi2) * \
math.sin(delta_lambda / 2.0) 2
c = 2 * math.atan2(math.sqrt(a), math.sqrt(1 - a))
return R * c
示例调用
distance = haversine((37.4219999, -122.0840575), (37.33182, -122.03118))
print(f"距离: {distance} 千米")
三、构建矩阵
将处理好的地理坐标和计算出的距离数据构建成矩阵,便于进一步分析和计算。
- 距离矩阵
通过计算所有用户地点之间的距离,构建距离矩阵。矩阵中的每个元素表示两个地点之间的距离:
num_locations = len(coordinates_matrix)
distance_matrix = np.zeros((num_locations, num_locations))
for i in range(num_locations):
for j in range(i + 1, num_locations):
distance = haversine(coordinates_matrix[i], coordinates_matrix[j])
distance_matrix[i, j] = distance
distance_matrix[j, i] = distance
print(distance_matrix)
- 构建其他类型的矩阵
根据具体需求,还可以构建其他类型的矩阵。例如,可以构建包含用户地点及其属性的矩阵,便于进行聚类分析或其他机器学习任务。
# 示例:构建包含用户地点及其属性的矩阵
import pandas as pd
user_data = {
'Location': user_locations,
'Latitude': [coord[0] for coord in coordinates_matrix],
'Longitude': [coord[1] for coord in coordinates_matrix]
}
user_df = pd.DataFrame(user_data)
print(user_df)
通过以上步骤,可以使用Python将用户地点转换为矩阵,并根据具体需求进行进一步处理和分析。这个过程包括使用地理编码API获取经纬度坐标、处理地理坐标数据、构建矩阵。无论是距离矩阵还是其他类型的矩阵,都可以根据实际需求进行定制和扩展。
相关问答FAQs:
如何在Python中获取用户的地理位置?
在Python中,获取用户的地理位置通常可以使用Geopy库。通过GeoPy的Geocoder功能,可以将用户输入的地址转换为经纬度坐标。安装GeoPy库后,可以使用geocode
方法来获取用户位置的坐标信息。这样,您就可以为后续的矩阵转换做好准备。
如何将经纬度数据转换为矩阵形式?
将经纬度数据转换为矩阵形式,可以使用NumPy库。首先,将经纬度数据存储为列表或数组,然后使用numpy.array()
方法将其转换为矩阵形式。这样,可以方便地进行数学运算或数据分析,比如计算距离、聚类等。
在Python中如何处理缺失的地理位置信息?
处理缺失的地理位置信息可以通过多种方式完成。常用的方法包括填补缺失值、删除缺失值或使用默认值(如城市中心坐标)。如果使用GeoPy获取位置时返回空结果,可以考虑使用其他地理编码API,或为用户提供一个反馈机制,以便他们可以手动输入缺失的信息。
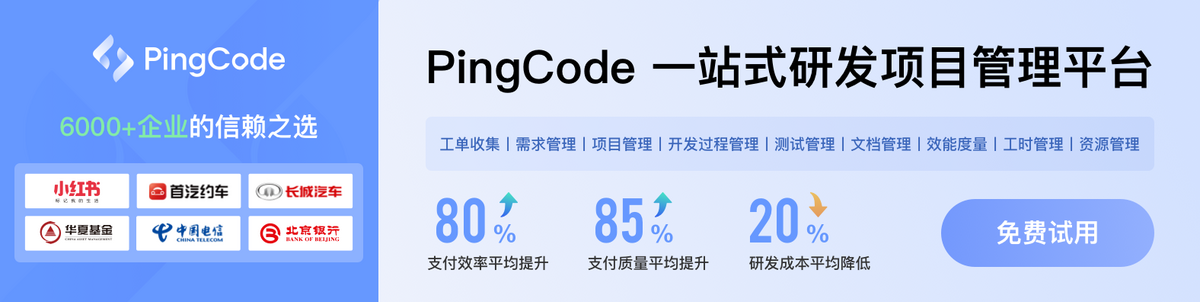