如何用Python编程来计算人口增长
Python是一种功能强大、灵活且易于学习的编程语言,适用于各种数据分析任务,包括人口增长计算。通过数据导入、公式应用、可视化工具、预测模型,我们可以对人口增长进行全面的分析。本文将详细介绍如何使用Python编程来计算和预测人口增长。
一、数据导入
在进行任何数据分析之前,首先需要导入和清理数据。数据可以来自各种来源,如CSV文件、SQL数据库或API接口。
1. 使用Pandas导入数据
Pandas是一个强大的数据操作库,适用于数据导入、清理和分析。以下是一个基本的导入CSV文件的示例:
import pandas as pd
导入CSV文件
data = pd.read_csv('population_data.csv')
查看数据前几行
print(data.head())
2. 数据清理
导入数据后,通常需要进行一些数据清理工作,如处理缺失值、数据类型转换等。
# 处理缺失值
data = data.dropna()
数据类型转换
data['Year'] = data['Year'].astype(int)
data['Population'] = data['Population'].astype(float)
二、公式应用
计算人口增长率需要用到一些基本的数学公式。常用的公式包括年增长率、复合年增长率等。
1. 年增长率
年增长率的公式为:
[ \text{年增长率} = \left( \frac{\text{当前人口}}{\text{上一年人口}} – 1 \right) \times 100 ]
# 计算年增长率
data['Growth_Rate'] = data['Population'].pct_change() * 100
print(data.head())
2. 复合年增长率(CAGR)
复合年增长率的公式为:
[ \text{CAGR} = \left( \frac{\text{最终人口}}{\text{初始人口}} \right)^{\frac{1}{n}} – 1 ]
其中,n为年份数。
# 计算复合年增长率
initial_population = data['Population'].iloc[0]
final_population = data['Population'].iloc[-1]
years = len(data)
CAGR = (final_population / initial_population) (1/years) - 1
print(f'复合年增长率: {CAGR*100:.2f}%')
三、可视化工具
数据可视化是理解和展示人口增长的重要手段。Python提供了多种可视化工具,如Matplotlib、Seaborn等。
1. 使用Matplotlib进行可视化
Matplotlib是一个基础且功能强大的可视化库。
import matplotlib.pyplot as plt
绘制人口增长曲线
plt.figure(figsize=(10, 6))
plt.plot(data['Year'], data['Population'], marker='o')
plt.title('Population Growth Over Years')
plt.xlabel('Year')
plt.ylabel('Population')
plt.grid(True)
plt.show()
2. 使用Seaborn进行高级可视化
Seaborn基于Matplotlib,提供了更高级的可视化功能。
import seaborn as sns
绘制带有回归线的散点图
plt.figure(figsize=(10, 6))
sns.regplot(x='Year', y='Population', data=data)
plt.title('Population Growth with Regression Line')
plt.xlabel('Year')
plt.ylabel('Population')
plt.grid(True)
plt.show()
四、预测模型
为了预测未来的人口增长,可以使用多种机器学习和统计模型。常用的方法包括线性回归、时间序列分析和机器学习模型。
1. 线性回归
线性回归是一种简单且常用的预测模型。
from sklearn.linear_model import LinearRegression
import numpy as np
准备数据
X = data['Year'].values.reshape(-1, 1)
y = data['Population'].values
建立模型
model = LinearRegression()
model.fit(X, y)
预测未来人口
future_years = np.array([2025, 2030, 2035]).reshape(-1, 1)
predictions = model.predict(future_years)
print(f'未来人口预测: {predictions}')
2. 时间序列分析
时间序列分析是处理时间序列数据的强大工具,如ARIMA模型。
from statsmodels.tsa.arima_model import ARIMA
建立时间序列模型
model = ARIMA(data['Population'], order=(5, 1, 0))
model_fit = model.fit(disp=0)
预测未来人口
forecast = model_fit.forecast(steps=10)[0]
print(f'未来10年人口预测: {forecast}')
五、案例分析
为了更好地理解如何使用Python计算人口增长,我们可以通过一个具体案例进行详细分析。
1. 案例背景
假设我们有一个国家从2000年到2020年的人口数据,我们希望计算其年增长率、复合年增长率,并预测未来10年的人口。
2. 数据准备
首先,我们需要准备数据并进行清理。
import pandas as pd
模拟数据
data = {
'Year': list(range(2000, 2021)),
'Population': [1000, 1020, 1045, 1070, 1100, 1130, 1165, 1200, 1235, 1270, 1300, 1330, 1365, 1400, 1435, 1470, 1505, 1540, 1580, 1620, 1660]
}
df = pd.DataFrame(data)
查看数据
print(df.head())
3. 计算年增长率和复合年增长率
# 计算年增长率
df['Growth_Rate'] = df['Population'].pct_change() * 100
计算复合年增长率
initial_population = df['Population'].iloc[0]
final_population = df['Population'].iloc[-1]
years = len(df)
CAGR = (final_population / initial_population) (1/years) - 1
print(f'复合年增长率: {CAGR*100:.2f}%')
4. 数据可视化
import matplotlib.pyplot as plt
import seaborn as sns
绘制人口增长曲线
plt.figure(figsize=(10, 6))
plt.plot(df['Year'], df['Population'], marker='o')
plt.title('Population Growth Over Years')
plt.xlabel('Year')
plt.ylabel('Population')
plt.grid(True)
plt.show()
绘制带有回归线的散点图
plt.figure(figsize=(10, 6))
sns.regplot(x='Year', y='Population', data=df)
plt.title('Population Growth with Regression Line')
plt.xlabel('Year')
plt.ylabel('Population')
plt.grid(True)
plt.show()
5. 预测未来人口
from sklearn.linear_model import LinearRegression
import numpy as np
准备数据
X = df['Year'].values.reshape(-1, 1)
y = df['Population'].values
建立模型
model = LinearRegression()
model.fit(X, y)
预测未来人口
future_years = np.array([2025, 2030, 2035]).reshape(-1, 1)
predictions = model.predict(future_years)
print(f'未来人口预测: {predictions}')
六、总结
通过本文的详细介绍,我们学习了如何使用Python编程来计算和预测人口增长。数据导入、公式应用、可视化工具、预测模型是实现这一目标的关键步骤。通过这些步骤,我们不仅可以计算当前的人口增长率,还可以预测未来的人口变化趋势,为决策提供有力的数据支持。
Python的灵活性和强大功能,使其成为数据分析和预测的理想工具。无论是简单的年增长率计算,还是复杂的时间序列分析,Python都能胜任。希望本文能够帮助你更好地理解和掌握如何使用Python进行人口增长的计算和预测。
相关问答FAQs:
如何使用Python编程来模拟人口增长的模型?
模拟人口增长通常涉及使用数学模型,例如指数增长模型或逻辑增长模型。可以通过定义一个函数,使用这些模型的公式来计算特定时间段内的人口变化。例如,使用公式 P(t) = P0 * e^(rt) 来表示人口的指数增长,其中 P0 是初始人口,r 是增长率,t 是时间。可以通过编写代码来实现这些公式,并使用 matplotlib 库可视化人口变化。
在Python中如何处理人口增长数据?
在处理人口增长数据时,可以使用 pandas 库来读取和分析数据集。通过导入数据文件(如CSV),可以计算不同时间段的人口增长率,绘制图表,甚至进行预测分析。同时,利用 NumPy 和 SciPy 库可以进行更复杂的数学运算和统计分析,帮助理解人口增长的趋势。
如何在Python中绘制人口增长的图表?
为了在Python中绘制人口增长图表,可以使用 matplotlib 或 seaborn 库。这些库允许用户创建直观的可视化效果,帮助展示人口随时间的变化。例如,可以绘制时间与人口数量的折线图,清晰显示增长趋势。通过设置合适的坐标轴标签和标题,可以使图表更具信息性和可读性。
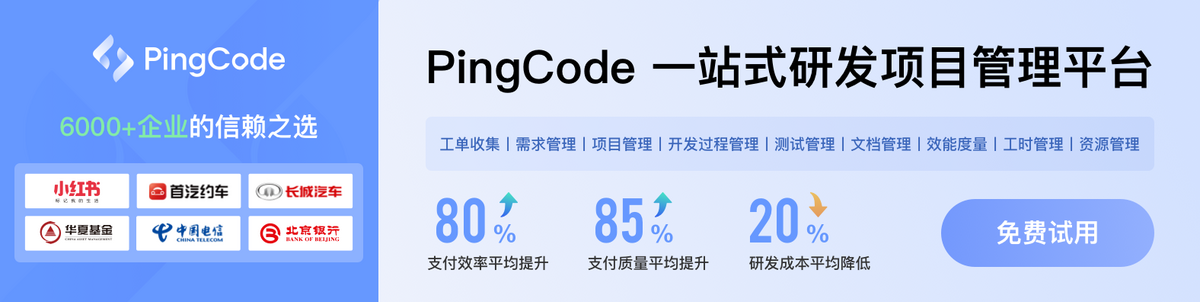