在Python中抓取网页时处理验证码的常见方法包括:使用第三方服务、图像识别、基于机器学习的解决方案、绕过验证码设计。这些方法各有优劣,具体选择取决于验证码的复杂性和项目需求。
使用第三方服务是最常见且便捷的方法。第三方服务如2Captcha、Anti-Captcha等可以自动处理大部分常见的验证码类型。这些服务通常收费,但它们提供了简单的API接口,可以方便地集成到Python爬虫中。使用第三方服务的一个主要优点是省去了自己识别验证码的麻烦,节省了开发时间和资源。
为了更好地理解上述方法,下面我们将详细探讨Python抓取网页时处理验证码的几个常见方法:
一、使用第三方服务
第三方服务如2Captcha、Anti-Captcha等提供了强大的验证码识别功能。它们通常通过API接口提供服务,用户只需将验证码图片上传,服务会返回识别结果。
1、集成2Captcha服务
2Captcha是一个广泛使用的验证码识别服务。它支持多种验证码类型,包括图片验证码、reCAPTCHA等。集成2Captcha到Python项目中非常简单,下面是一个基本示例:
import requests
def solve_captcha(api_key, captcha_image):
url = "http://2captcha.com/in.php"
files = {'file': ('captcha.jpg', captcha_image)}
data = {'key': api_key, 'method': 'post'}
# 上传验证码图片
response = requests.post(url, files=files, data=data)
captcha_id = response.text.split('|')[1]
# 获取识别结果
result_url = f"http://2captcha.com/res.php?key={api_key}&action=get&id={captcha_id}"
while True:
result = requests.get(result_url).text
if result.startswith('OK'):
captcha_text = result.split('|')[1]
return captcha_text
使用示例
api_key = "YOUR_2CAPTCHA_API_KEY"
captcha_image = open("path_to_captcha_image.jpg", "rb").read()
captcha_text = solve_captcha(api_key, captcha_image)
print("Captcha text:", captcha_text)
通过这种方式,你可以轻松地将验证码识别功能集成到你的Python爬虫中。
2、使用Anti-Captcha服务
Anti-Captcha也是一种流行的验证码识别服务。与2Captcha类似,它提供了简单的API接口,用户只需上传验证码图片即可获得识别结果。下面是一个基本示例:
import requests
def solve_captcha(api_key, captcha_image):
url = "https://api.anti-captcha.com/createTask"
headers = {'Content-Type': 'application/json'}
data = {
'clientKey': api_key,
'task': {
'type': 'ImageToTextTask',
'body': captcha_image
}
}
# 上传验证码图片
response = requests.post(url, headers=headers, json=data)
task_id = response.json()['taskId']
# 获取识别结果
result_url = "https://api.anti-captcha.com/getTaskResult"
while True:
result = requests.post(result_url, headers=headers, json={'clientKey': api_key, 'taskId': task_id}).json()
if result['status'] == 'ready':
captcha_text = result['solution']['text']
return captcha_text
使用示例
api_key = "YOUR_ANTI_CAPTCHA_API_KEY"
captcha_image = open("path_to_captcha_image.jpg", "rb").read().encode('base64')
captcha_text = solve_captcha(api_key, captcha_image)
print("Captcha text:", captcha_text)
通过这种方式,你可以轻松地将Anti-Captcha服务集成到你的Python爬虫中。
二、图像识别
图像识别是一种常见的验证码处理方法,尤其适用于简单的图片验证码。使用图像识别技术,用户可以通过OCR(光学字符识别)工具自动识别验证码文本。
1、使用Tesseract OCR
Tesseract是一个开源的OCR工具,支持多种语言和字符集。它可以方便地与Python结合使用,通过Pytesseract库来调用。下面是一个基本示例:
import pytesseract
from PIL import Image
def solve_captcha(captcha_image_path):
# 打开验证码图片
image = Image.open(captcha_image_path)
# 使用Tesseract OCR识别验证码文本
captcha_text = pytesseract.image_to_string(image)
return captcha_text
使用示例
captcha_image_path = "path_to_captcha_image.jpg"
captcha_text = solve_captcha(captcha_image_path)
print("Captcha text:", captcha_text)
尽管Tesseract在处理简单的图片验证码时表现良好,但面对复杂的验证码(如扭曲、噪声、干扰线等)时,它的识别准确率可能较低。因此,对于复杂的验证码,通常需要结合图像预处理技术来提高识别准确率。
2、图像预处理技术
图像预处理是提高OCR识别准确率的有效方法。常见的图像预处理技术包括去噪、二值化、倾斜校正等。下面是一个示例,展示如何使用OpenCV进行图像预处理:
import cv2
import pytesseract
from PIL import Image
def preprocess_image(image_path):
# 读取图片
image = cv2.imread(image_path)
# 转换为灰度图像
gray = cv2.cvtColor(image, cv2.COLOR_BGR2GRAY)
# 二值化处理
_, binary = cv2.threshold(gray, 128, 255, cv2.THRESH_BINARY)
# 去噪处理
denoised = cv2.fastNlMeansDenoising(binary, h=30)
# 保存预处理后的图片
preprocessed_image_path = "preprocessed_image.jpg"
cv2.imwrite(preprocessed_image_path, denoised)
return preprocessed_image_path
def solve_captcha(captcha_image_path):
# 预处理图片
preprocessed_image_path = preprocess_image(captcha_image_path)
# 使用Tesseract OCR识别验证码文本
image = Image.open(preprocessed_image_path)
captcha_text = pytesseract.image_to_string(image)
return captcha_text
使用示例
captcha_image_path = "path_to_captcha_image.jpg"
captcha_text = solve_captcha(captcha_image_path)
print("Captcha text:", captcha_text)
通过结合图像预处理和OCR技术,可以显著提高验证码识别的准确率。
三、基于机器学习的解决方案
基于机器学习的解决方案是验证码识别的高级方法,尤其适用于复杂的验证码。通过训练神经网络模型,可以自动识别各种类型的验证码。
1、构建数据集
构建高质量的训练数据集是机器学习方法的关键。通常需要收集大量带标签的验证码图片,并进行数据增强处理,如旋转、缩放、噪声添加等,以提高模型的泛化能力。
2、训练神经网络模型
训练神经网络模型可以使用深度学习框架,如TensorFlow、PyTorch等。下面是一个基本示例,展示如何使用TensorFlow训练验证码识别模型:
import tensorflow as tf
from tensorflow.keras.models import Sequential
from tensorflow.keras.layers import Conv2D, MaxPooling2D, Flatten, Dense
from tensorflow.keras.preprocessing.image import ImageDataGenerator
def build_model():
model = Sequential([
Conv2D(32, (3, 3), activation='relu', input_shape=(60, 160, 1)),
MaxPooling2D((2, 2)),
Conv2D(64, (3, 3), activation='relu'),
MaxPooling2D((2, 2)),
Flatten(),
Dense(128, activation='relu'),
Dense(36, activation='softmax') # 假设验证码只有数字和字母
])
model.compile(optimizer='adam', loss='categorical_crossentropy', metrics=['accuracy'])
return model
def train_model(model, train_data_dir, val_data_dir):
train_datagen = ImageDataGenerator(rescale=0.1/255)
val_datagen = ImageDataGenerator(rescale=0.1/255)
train_generator = train_datagen.flow_from_directory(train_data_dir, target_size=(60, 160), color_mode='grayscale', batch_size=32, class_mode='categorical')
val_generator = val_datagen.flow_from_directory(val_data_dir, target_size=(60, 160), color_mode='grayscale', batch_size=32, class_mode='categorical')
model.fit(train_generator, epochs=50, validation_data=val_generator)
使用示例
model = build_model()
train_data_dir = "path_to_train_data"
val_data_dir = "path_to_val_data"
train_model(model, train_data_dir, val_data_dir)
通过这种方式,你可以训练一个专门用于识别验证码的神经网络模型,显著提高验证码识别的准确率。
四、绕过验证码设计
在某些情况下,绕过验证码设计是处理验证码的有效方法。绕过验证码设计的方法包括利用网站漏洞、使用无验证码接口等。
1、利用网站漏洞
有些网站的验证码实现存在漏洞,例如验证码图片地址可预测、验证码验证逻辑存在缺陷等。通过利用这些漏洞,可以绕过验证码验证。
2、使用无验证码接口
有些网站提供无验证码的API接口,使用这些接口可以避免验证码验证。例如,一些网站的移动端API接口可能没有验证码验证,通过模拟移动端请求可以绕过验证码。
import requests
def get_data_without_captcha(api_url, headers):
response = requests.get(api_url, headers=headers)
return response.json()
使用示例
api_url = "https://example.com/api/data"
headers = {'User-Agent': 'Mozilla/5.0 (Linux; Android 9; Mobile Safari/537.36'}
data = get_data_without_captcha(api_url, headers)
print("Data:", data)
通过这种方式,你可以绕过验证码设计,直接获取所需数据。
结论
Python抓取网页时处理验证码的方法多种多样,具体选择取决于验证码的复杂性和项目需求。使用第三方服务是最便捷的方法,图像识别适用于简单的图片验证码,基于机器学习的解决方案适用于复杂的验证码,绕过验证码设计在某些情况下也是有效的解决方案。
通过合理选择和组合这些方法,可以有效地处理各种类型的验证码,提高网页抓取的效率和准确性。
相关问答FAQs:
如何在使用Python抓取网页时识别验证码?
在抓取网页时,验证码通常是为了防止自动化访问而设置的。针对这一挑战,有几种方法可以尝试识别验证码。使用OCR(光学字符识别)库如Tesseract,可以帮助识别验证码中的字符。首先,将验证码图像保存下来,然后使用OCR工具对图像进行处理,提取出文本。需要注意的是,OCR的准确性可能会受到验证码复杂性的影响。
使用Python抓取网页时,有哪些库可以处理验证码?
处理验证码的库有多种选择。常用的有Selenium,它不仅可以模拟浏览器行为,还可以处理动态加载的内容。在Selenium中,可以通过截图获取验证码并结合OCR进行识别。还有一些专门的验证码识别API,如Anti-Captcha和2Captcha,可以通过调用API来识别验证码,这样可以减少开发的复杂性。
如果验证码无法自动识别,应该如何手动处理?
在某些情况下,自动识别验证码可能会失败。这时,可以考虑手动干预。可以在抓取的脚本中引入一个暂停,提示用户手动输入验证码。这样可以确保数据的准确性。此外,设计一个简易的用户界面(如使用Tkinter)来让用户输入验证码,也是一个不错的解决方案。这样可以兼顾自动化与人工干预,提高抓取的成功率。
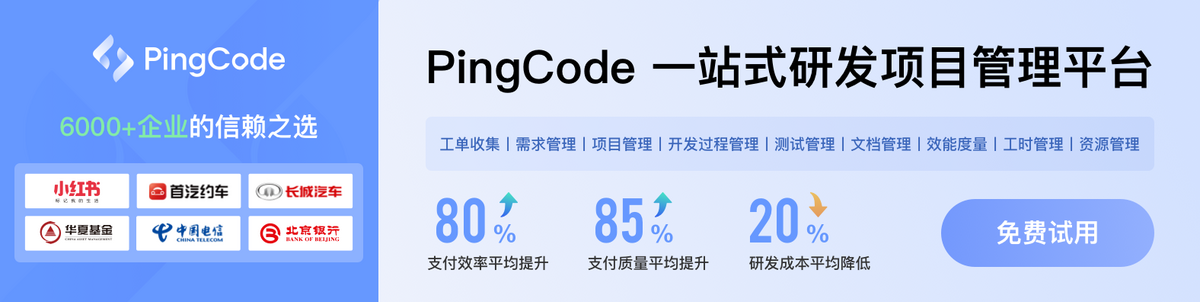