在Python中比较像素值通常涉及到图像处理,常用的库包括OpenCV和Pillow。这些库提供了读取、处理和比较图像像素值的功能。比较像素值的方法主要有:逐像素比较、计算图像差异、使用结构相似性指数(SSIM)。下面将详细介绍逐像素比较这一方法。
逐像素比较是最直接的方法,通过逐像素地遍历图像,比较每个像素的值来判断图像的相似度或差异。这种方法适用于图像尺寸相同的情况,且可以具体到每个像素的RGB值进行比较。使用OpenCV库可以方便地实现逐像素比较:
import cv2
import numpy as np
def compare_images(img1_path, img2_path):
img1 = cv2.imread(img1_path)
img2 = cv2.imread(img2_path)
if img1.shape != img2.shape:
return False
difference = cv2.subtract(img1, img2)
b, g, r = cv2.split(difference)
if cv2.countNonZero(b) == 0 and cv2.countNonZero(g) == 0 and cv2.countNonZero(r) == 0:
return True
else:
return False
result = compare_images('image1.png', 'image2.png')
print("Images are identical:", result)
上述代码通过逐像素比较图像的RGB值,判断图像是否完全相同。接下来将详细介绍其他方法及其实现。
一、逐像素比较
逐像素比较是最基础和直观的比较方法,适用于图像大小一致的情况。我们可以使用OpenCV或Pillow库来实现逐像素比较。
OpenCV实现逐像素比较
OpenCV是一个强大的图像处理库,提供了多种图像操作函数。以下是使用OpenCV逐像素比较图像的详细步骤:
import cv2
import numpy as np
def compare_images(img1_path, img2_path):
img1 = cv2.imread(img1_path)
img2 = cv2.imread(img2_path)
if img1.shape != img2.shape:
return False
difference = cv2.subtract(img1, img2)
b, g, r = cv2.split(difference)
if cv2.countNonZero(b) == 0 and cv2.countNonZero(g) == 0 and cv2.countNonZero(r) == 0:
return True
else:
return False
result = compare_images('image1.png', 'image2.png')
print("Images are identical:", result)
在上述代码中,我们读取两幅图像,并检查它们的形状是否相同。如果形状不同,则直接返回False。然后通过cv2.subtract
函数计算图像差异,并分离出蓝色、绿色和红色通道。最后通过cv2.countNonZero
函数判断每个通道是否存在非零像素,如果所有通道都没有非零像素,则说明两幅图像完全相同。
Pillow实现逐像素比较
Pillow是一个轻量级的图像处理库,适合处理简单的图像操作。以下是使用Pillow逐像素比较图像的详细步骤:
from PIL import Image
import numpy as np
def compare_images(img1_path, img2_path):
img1 = Image.open(img1_path)
img2 = Image.open(img2_path)
if img1.size != img2.size:
return False
img1 = np.array(img1)
img2 = np.array(img2)
difference = np.subtract(img1, img2)
if not np.any(difference):
return True
else:
return False
result = compare_images('image1.png', 'image2.png')
print("Images are identical:", result)
在上述代码中,我们使用Pillow库读取图像并转换为NumPy数组。然后通过np.subtract
函数计算图像差异,并使用np.any
函数判断差异数组中是否存在非零元素。如果不存在非零元素,则说明两幅图像完全相同。
二、计算图像差异
计算图像差异是一种更为灵活的方法,可以用于判断图像之间的相似度或找出图像的不同区域。我们可以使用均方误差(MSE)和峰值信噪比(PSNR)来度量图像差异。
使用均方误差(MSE)计算图像差异
均方误差(MSE)是衡量图像差异的一种常用方法,计算两幅图像像素值差异的平方和的平均值。以下是使用OpenCV实现MSE的方法:
import cv2
import numpy as np
def mse(imageA, imageB):
err = np.sum((imageA.astype("float") - imageB.astype("float")) 2)
err /= float(imageA.shape[0] * imageA.shape[1])
return err
def compare_images(img1_path, img2_path):
img1 = cv2.imread(img1_path, cv2.IMREAD_GRAYSCALE)
img2 = cv2.imread(img2_path, cv2.IMREAD_GRAYSCALE)
if img1.shape != img2.shape:
return False
error = mse(img1, img2)
return error
result = compare_images('image1.png', 'image2.png')
print("Mean Squared Error:", result)
在上述代码中,我们将图像转换为灰度图,并计算两幅图像的均方误差。如果均方误差为零,则说明两幅图像完全相同。
使用峰值信噪比(PSNR)计算图像差异
峰值信噪比(PSNR)是一种衡量图像质量的标准,通常用于图像压缩和恢复的质量评估。以下是使用OpenCV实现PSNR的方法:
import cv2
import numpy as np
def psnr(imageA, imageB):
mse_value = np.mean((imageA - imageB) 2)
if mse_value == 0:
return 100
max_pixel = 255.0
psnr_value = 20 * np.log10(max_pixel / np.sqrt(mse_value))
return psnr_value
def compare_images(img1_path, img2_path):
img1 = cv2.imread(img1_path)
img2 = cv2.imread(img2_path)
if img1.shape != img2.shape:
return False
psnr_value = psnr(img1, img2)
return psnr_value
result = compare_images('image1.png', 'image2.png')
print("PSNR:", result)
在上述代码中,我们计算两幅图像的均方误差,并根据均方误差计算PSNR值。如果PSNR值较高,则说明两幅图像的相似度较高。
三、使用结构相似性指数(SSIM)
结构相似性指数(SSIM)是一种衡量图像相似度的指标,考虑了图像的亮度、对比度和结构信息。SSIM在很多图像处理任务中被广泛使用,因为它能够更好地反映人类视觉感知的图像质量。以下是使用OpenCV和scikit-image库实现SSIM的方法:
import cv2
from skimage.metrics import structural_similarity as ssim
def compare_images(img1_path, img2_path):
img1 = cv2.imread(img1_path, cv2.IMREAD_GRAYSCALE)
img2 = cv2.imread(img2_path, cv2.IMREAD_GRAYSCALE)
if img1.shape != img2.shape:
return False
ssim_value, _ = ssim(img1, img2, full=True)
return ssim_value
result = compare_images('image1.png', 'image2.png')
print("SSIM:", result)
在上述代码中,我们将图像转换为灰度图,并使用ssim
函数计算两幅图像的SSIM值。SSIM值范围在-1到1之间,值越接近1表示两幅图像越相似。
四、使用模板匹配
模板匹配是一种在图像中查找模板图像的方法,通常用于目标检测和图像识别。以下是使用OpenCV实现模板匹配的方法:
import cv2
import numpy as np
def template_matching(image_path, template_path):
image = cv2.imread(image_path, cv2.IMREAD_GRAYSCALE)
template = cv2.imread(template_path, cv2.IMREAD_GRAYSCALE)
result = cv2.matchTemplate(image, template, cv2.TM_CCOEFF_NORMED)
_, max_val, _, max_loc = cv2.minMaxLoc(result)
return max_val, max_loc
result_val, result_loc = template_matching('image.png', 'template.png')
print("Template Matching Value:", result_val)
print("Template Matching Location:", result_loc)
在上述代码中,我们将图像和模板图像转换为灰度图,并使用cv2.matchTemplate
函数进行模板匹配。模板匹配结果中包含匹配值和匹配位置,匹配值越高表示模板图像与目标图像的相似度越高。
五、使用直方图比较
直方图比较是一种基于图像像素值分布的比较方法,常用于图像检索和分类。以下是使用OpenCV实现直方图比较的方法:
import cv2
def histogram_comparison(img1_path, img2_path):
img1 = cv2.imread(img1_path)
img2 = cv2.imread(img2_path)
hist1 = cv2.calcHist([img1], [0, 1, 2], None, [8, 8, 8], [0, 256, 0, 256, 0, 256])
hist2 = cv2.calcHist([img2], [0, 1, 2], None, [8, 8, 8], [0, 256, 0, 256, 0, 256])
cv2.normalize(hist1, hist1)
cv2.normalize(hist2, hist2)
similarity = cv2.compareHist(hist1, hist2, cv2.HISTCMP_CORREL)
return similarity
result = histogram_comparison('image1.png', 'image2.png')
print("Histogram Similarity:", result)
在上述代码中,我们计算两幅图像的直方图,并使用cv2.compareHist
函数比较直方图的相似度。相似度值越高表示两幅图像的像素值分布越相似。
六、使用特征点匹配
特征点匹配是一种基于图像关键点的比较方法,常用于图像配准和目标检测。以下是使用OpenCV实现特征点匹配的方法:
import cv2
def feature_matching(img1_path, img2_path):
img1 = cv2.imread(img1_path, cv2.IMREAD_GRAYSCALE)
img2 = cv2.imread(img2_path, cv2.IMREAD_GRAYSCALE)
orb = cv2.ORB_create()
keypoints1, descriptors1 = orb.detectAndCompute(img1, None)
keypoints2, descriptors2 = orb.detectAndCompute(img2, None)
bf = cv2.BFMatcher(cv2.NORM_HAMMING, crossCheck=True)
matches = bf.match(descriptors1, descriptors2)
matches = sorted(matches, key=lambda x: x.distance)
return matches
matches = feature_matching('image1.png', 'image2.png')
print("Number of Matches:", len(matches))
在上述代码中,我们使用ORB特征点检测器提取图像的关键点和描述符,并使用BFMatcher进行特征点匹配。匹配结果中包含匹配的特征点对数,特征点对数越多表示两幅图像的相似度越高。
七、使用深度学习模型
深度学习模型可以用于图像分类、检索和比较,常用于复杂图像处理任务。以下是使用预训练的深度学习模型(如VGG16)进行图像比较的方法:
import numpy as np
from keras.applications.vgg16 import VGG16, preprocess_input
from keras.preprocessing import image
from keras.models import Model
def deep_learning_comparison(img1_path, img2_path):
base_model = VGG16(weights='imagenet')
model = Model(inputs=base_model.input, outputs=base_model.get_layer('fc1').output)
img1 = image.load_img(img1_path, target_size=(224, 224))
img2 = image.load_img(img2_path, target_size=(224, 224))
img1 = image.img_to_array(img1)
img2 = image.img_to_array(img2)
img1 = np.expand_dims(img1, axis=0)
img2 = np.expand_dims(img2, axis=0)
img1 = preprocess_input(img1)
img2 = preprocess_input(img2)
features1 = model.predict(img1)
features2 = model.predict(img2)
similarity = np.dot(features1, features2.T) / (np.linalg.norm(features1) * np.linalg.norm(features2))
return similarity
result = deep_learning_comparison('image1.png', 'image2.png')
print("Deep Learning Similarity:", result)
在上述代码中,我们使用预训练的VGG16模型提取图像的高层次特征,并计算特征向量的余弦相似度。余弦相似度值范围在-1到1之间,值越接近1表示两幅图像的相似度越高。
八、图像哈希比较
图像哈希比较是一种将图像转换为简洁哈希值的方法,适用于图像检索和去重。以下是使用imagehash库实现图像哈希比较的方法:
from PIL import Image
import imagehash
def hash_comparison(img1_path, img2_path):
img1 = Image.open(img1_path)
img2 = Image.open(img2_path)
hash1 = imagehash.average_hash(img1)
hash2 = imagehash.average_hash(img2)
return hash1 - hash2
result = hash_comparison('image1.png', 'image2.png')
print("Hash Difference:", result)
在上述代码中,我们使用average_hash方法计算图像的哈希值,并计算哈希值之间的差异。哈希值差异越小表示两幅图像越相似。
九、颜色空间转换比较
颜色空间转换比较是一种将图像转换为不同颜色空间后进行比较的方法,适用于图像处理和分析。以下是使用OpenCV实现颜色空间转换比较的方法:
import cv2
def color_space_comparison(img1_path, img2_path):
img1 = cv2.imread(img1_path)
img2 = cv2.imread(img2_path)
img1_hsv = cv2.cvtColor(img1, cv2.COLOR_BGR2HSV)
img2_hsv = cv2.cvtColor(img2, cv2.COLOR_BGR2HSV)
difference = cv2.absdiff(img1_hsv, img2_hsv)
mean_diff = cv2.mean(difference)
return mean_diff
result = color_space_comparison('image1.png', 'image2.png')
print("Color Space Difference:", result)
在上述代码中,我们将图像转换为HSV颜色空间,并计算两幅图像在HSV颜色空间中的绝对差异。差异值越小表示两幅图像越相似。
总结
本文详细介绍了Python中比较像素值的多种方法,包括逐像素比较、计算图像差异、使用结构相似性指数(SSIM)、模板匹配、直方图比较、特征点匹配、深度学习模型、图像哈希比较和颜色空间转换比较。每种方法都有其适用场景和特点,选择合适的方法可以有效地完成图像比较任务。希望本文能够帮助您更好地理解和应用这些方法。
相关问答FAQs:
如何在Python中读取和处理图像的像素值?
在Python中,可以使用Pillow库(PIL)来读取和处理图像。首先,通过Image.open()
方法加载图像,然后可以使用load()
方法获取像素数据。这样,你就可以访问每个像素的RGB值,并进行比较。安装Pillow库可以通过命令pip install Pillow
来完成。
在比较两个图像的像素值时,有哪些常用的方法?
比较两个图像的像素值可以通过多种方式实现。一种常用方法是逐像素比较RGB值,使用NumPy库可以更高效地处理这些数据。通过将图像转换为数组,您可以直接使用数组运算进行比较。此外,计算图像之间的均方根误差(RMSE)也是一种有效的比较方式,能够量化两幅图像之间的差异。
使用OpenCV库进行像素比较时,有哪些常见的技巧?
OpenCV是另一个强大的图像处理库,适用于像素比较。您可以使用cv2.imread()
函数读取图像,并使用cv2.absdiff()
函数计算两个图像之间的绝对差异。这种方式特别适合在图像中寻找变化区域。此外,使用cv2.threshold()
函数可以将差异图像转换为二进制图像,便于进一步分析和处理。
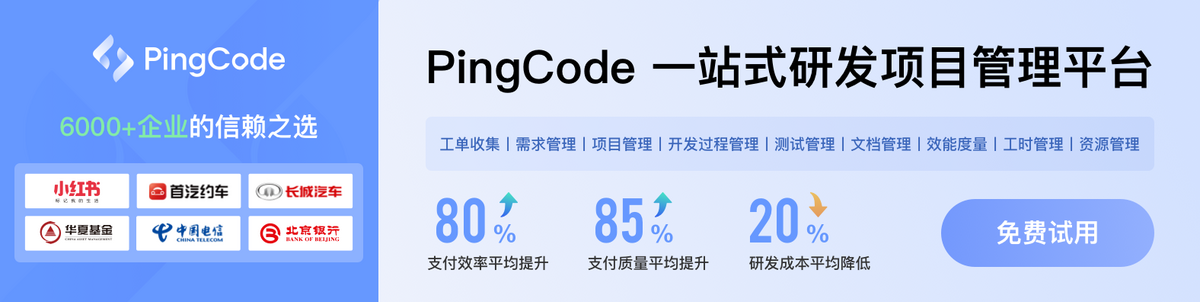