使用Python绘制输出矩阵可以通过几种常见的方法来实现,包括使用matplotlib
、seaborn
、pandas
中的plot
方法等。 其中,matplotlib
是最基础也是最广泛使用的绘图库,能够提供非常灵活的绘图功能。具体方法包括:
- 使用
matplotlib
绘制热图:这是最常见的方法,能够直观地显示矩阵中的数值大小。 - 使用
seaborn
绘制热图:seaborn
是基于matplotlib
的高级绘图库,提供了更多美观的默认样式和更简洁的API。 - 使用
pandas
绘图:如果矩阵数据存储在pandas DataFrame
中,可以直接调用其内置的绘图方法来生成图表。
使用matplotlib
绘制热图
matplotlib
是一个强大的绘图库,可以通过其子模块pyplot
来绘制各种类型的图表。下面是一个使用matplotlib
绘制热图的示例。
import matplotlib.pyplot as plt
import numpy as np
创建一个随机矩阵
matrix = np.random.rand(10, 10)
使用matplotlib绘制热图
plt.imshow(matrix, cmap='viridis', interpolation='nearest')
plt.colorbar()
plt.title('Heatmap of Matrix')
plt.xlabel('Column')
plt.ylabel('Row')
plt.show()
在上面的代码中,np.random.rand(10, 10)
生成了一个10×10的随机矩阵。plt.imshow
函数用于绘制热图,cmap
参数指定了颜色映射,interpolation
参数控制插值方式。plt.colorbar
添加了一个颜色条以指示颜色与数据值的对应关系。
使用seaborn
绘制热图
seaborn
提供了一个更高级和简洁的API来绘制图表,特别是热图。在使用seaborn
之前,需要先安装它:
pip install seaborn
下面是一个使用seaborn
绘制热图的示例:
import seaborn as sns
import numpy as np
创建一个随机矩阵
matrix = np.random.rand(10, 10)
使用seaborn绘制热图
sns.heatmap(matrix, cmap='viridis', annot=True)
plt.title('Heatmap of Matrix')
plt.xlabel('Column')
plt.ylabel('Row')
plt.show()
在这个示例中,sns.heatmap
函数用于绘制热图,cmap
参数指定了颜色映射,annot=True
参数表示在每个单元格中显示数值。
使用pandas
绘图
如果你的矩阵数据存储在pandas DataFrame
中,可以直接使用pandas
的绘图方法:
import pandas as pd
import numpy as np
import matplotlib.pyplot as plt
创建一个随机矩阵并转换为DataFrame
matrix = np.random.rand(10, 10)
df = pd.DataFrame(matrix)
使用pandas绘制热图
plt.matshow(df, cmap='viridis')
plt.colorbar()
plt.title('Heatmap of Matrix')
plt.xlabel('Column')
plt.ylabel('Row')
plt.show()
在这个示例中,plt.matshow
函数用于绘制热图。
深入理解和应用
使用上述方法可以轻松地绘制出矩阵的图表,但在实际应用中,往往需要对图表进行更多的定制和优化。以下是一些更高级的技巧和示例,以帮助你更好地展示矩阵数据。
一、优化热图的显示效果
调整颜色映射:颜色映射决定了热图中数值与颜色的对应关系。matplotlib
和seaborn
提供了多种颜色映射,可以根据数据特点选择合适的颜色映射。
import matplotlib.pyplot as plt
import numpy as np
matrix = np.random.rand(10, 10)
plt.imshow(matrix, cmap='coolwarm', interpolation='nearest')
plt.colorbar()
plt.title('Heatmap with Coolwarm Color Map')
plt.xlabel('Column')
plt.ylabel('Row')
plt.show()
自定义颜色条:颜色条帮助我们理解颜色与数值的对应关系,可以对其进行自定义以提高可读性。
import seaborn as sns
import numpy as np
matrix = np.random.rand(10, 10)
sns.heatmap(matrix, cmap='YlGnBu', annot=True, cbar_kws={'label': 'Value'})
plt.title('Customized Color Bar')
plt.xlabel('Column')
plt.ylabel('Row')
plt.show()
调整刻度和标签:可以通过xticks
和yticks
函数调整刻度和标签,使图表更易于阅读。
import matplotlib.pyplot as plt
import numpy as np
matrix = np.random.rand(10, 10)
plt.imshow(matrix, cmap='viridis', interpolation='nearest')
plt.colorbar()
plt.xticks(np.arange(10), labels=[f'Col {i}' for i in range(10)], rotation=45)
plt.yticks(np.arange(10), labels=[f'Row {i}' for i in range(10)])
plt.title('Heatmap with Custom Labels')
plt.xlabel('Column')
plt.ylabel('Row')
plt.show()
二、增加数据的可视化维度
添加注释:在热图中添加数值注释可以帮助更直观地理解数据。
import seaborn as sns
import numpy as np
matrix = np.random.rand(10, 10)
sns.heatmap(matrix, cmap='viridis', annot=True, fmt='.2f')
plt.title('Heatmap with Annotations')
plt.xlabel('Column')
plt.ylabel('Row')
plt.show()
多图比较:在一个图表中展示多个热图,可以方便地进行数据比较。
import matplotlib.pyplot as plt
import numpy as np
matrix1 = np.random.rand(10, 10)
matrix2 = np.random.rand(10, 10)
fig, (ax1, ax2) = plt.subplots(1, 2, figsize=(12, 6))
ax1.imshow(matrix1, cmap='viridis', interpolation='nearest')
ax1.set_title('Matrix 1')
ax2.imshow(matrix2, cmap='viridis', interpolation='nearest')
ax2.set_title('Matrix 2')
plt.suptitle('Comparison of Two Matrices')
plt.show()
三、在实际项目中的应用
在实际项目中,绘制矩阵图表常用于以下几种场景:
数据探索和分析:通过绘制热图,可以快速了解数据的分布和特征,发现潜在的规律和异常。
机器学习模型的可视化:在训练和评估机器学习模型时,常常需要可视化混淆矩阵、相关矩阵等,以评估模型性能。
科学研究和报告:在科学研究和报告中,使用热图可以直观地展示实验结果和数据分析。
四、综合案例:机器学习混淆矩阵的可视化
混淆矩阵是评估分类模型性能的重要工具,下面是一个综合案例,展示如何使用matplotlib
和seaborn
绘制混淆矩阵的热图。
import numpy as np
import seaborn as sns
import matplotlib.pyplot as plt
from sklearn.datasets import load_digits
from sklearn.model_selection import train_test_split
from sklearn.ensemble import RandomForestClassifier
from sklearn.metrics import confusion_matrix
加载数据集
digits = load_digits()
X_train, X_test, y_train, y_test = train_test_split(digits.data, digits.target, test_size=0.3, random_state=42)
训练分类模型
clf = RandomForestClassifier(n_estimators=100, random_state=42)
clf.fit(X_train, y_train)
y_pred = clf.predict(X_test)
计算混淆矩阵
cm = confusion_matrix(y_test, y_pred)
可视化混淆矩阵
plt.figure(figsize=(10, 7))
sns.heatmap(cm, annot=True, fmt='d', cmap='Blues')
plt.title('Confusion Matrix')
plt.xlabel('Predicted Label')
plt.ylabel('True Label')
plt.show()
在这个案例中,首先加载手写数字数据集并进行训练和测试集划分。然后使用随机森林分类器进行训练和预测,并计算混淆矩阵。最后,使用seaborn
绘制混淆矩阵的热图,其中annot=True
表示在热图中添加数值注释,fmt='d'
表示数值的格式为整数,cmap='Blues'
表示使用蓝色颜色映射。
五、总结与展望
通过上述示例和案例,我们可以看到,Python提供了丰富的工具和方法来绘制矩阵图表,能够帮助我们更直观地理解和分析数据。在实际应用中,可以根据具体需求选择合适的绘图库和方法,并进行必要的定制和优化,以提高图表的可读性和美观性。
随着数据科学和机器学习的不断发展,数据可视化的重要性日益凸显。未来,可能会有更多更强大的绘图工具和库出现,帮助我们更高效地进行数据分析和展示。同时,我们也应不断学习和探索,掌握更多的可视化技术和技巧,以应对不断变化的数据分析需求。
相关问答FAQs:
如何使用Python绘制矩阵的可视化?
使用Python绘制矩阵可以借助多种库,如Matplotlib和Seaborn等。Matplotlib提供了基本的绘图功能,可以通过imshow()
函数将矩阵以图像的形式展现。Seaborn则提供了更为美观的热图功能,使用heatmap()
函数可以轻松创建热图并添加注释。通过这些工具,用户可以直观地理解矩阵数据的分布和关系。
绘制矩阵时,如何选择合适的颜色映射?
选择合适的颜色映射对于矩阵可视化至关重要。一般来说,使用渐变色或者离散色彩可以帮助突出重要的数值差异。Matplotlib和Seaborn都提供多种内置的颜色映射选项,用户可以根据数据的特性和需要传达的信息选择合适的颜色方案。例如,对于热图,常用的颜色映射有'viridis'、'plasma'和'inferno'等,这些可以通过cmap
参数指定。
如何在绘制矩阵时添加标签和注释以增强可读性?
为了提高矩阵图的可读性,可以在绘图时添加轴标签、标题以及数据注释。使用Matplotlib,可以通过xlabel()
和ylabel()
函数为坐标轴添加标签,而title()
函数可以添加图表标题。若希望在热图中显示每个单元格的数值,可以使用Seaborn的annot=True
参数,结合fmt
参数来控制格式。这些元素不仅使图表更具信息量,还能帮助观众更快地理解数据所传达的意义。
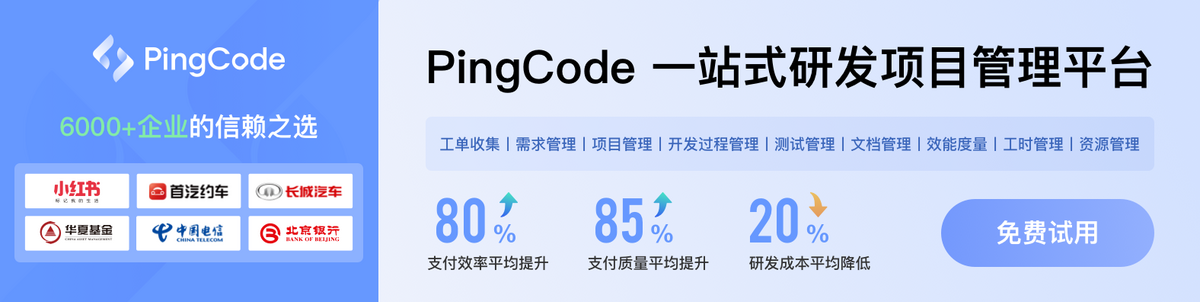