如何用Python分析熊市
通过数据分析技术、机器学习算法、可视化工具进行熊市分析。例如,使用Python可以通过编写代码进行数据收集、数据清洗、构建并训练机器学习模型,最终生成可视化图表来分析和预测熊市的走势。下面将详细介绍如何用Python实现这些步骤。
一、数据收集
数据收集是进行市场分析的第一步。对于分析熊市,我们需要收集股票市场的历史数据。可以通过以下几种方式获取数据:
- 使用API获取数据
API是获取金融数据的主要工具之一。常见的API包括Yahoo Finance、Alpha Vantage、Quandl等。这些API通常提供股票价格、交易量、技术指标等数据。
import yfinance as yf
获取指定股票的历史数据
ticker = 'AAPL'
data = yf.download(ticker, start="2010-01-01", end="2023-10-01")
print(data.head())
- 使用Web Scraping获取数据
如果API无法满足需求,可以使用Web Scraping技术从网站上抓取数据。常用的库有BeautifulSoup、Selenium等。
import requests
from bs4 import BeautifulSoup
url = 'https://finance.yahoo.com/quote/AAPL/history'
response = requests.get(url)
soup = BeautifulSoup(response.content, 'html.parser')
提取表格数据
table = soup.find('table', {'data-test': 'historical-prices'})
rows = table.find_all('tr')
for row in rows:
cols = row.find_all('td')
print([col.text for col in cols])
二、数据清洗
数据清洗是数据分析中不可或缺的一步。需要处理缺失值、去除异常值、格式转换等,以确保数据的质量。
- 处理缺失值
缺失值会影响模型的准确性,需要进行处理。可以选择删除含有缺失值的行或列,或者用均值、中位数等填补缺失值。
# 删除含有缺失值的行
data.dropna(inplace=True)
用均值填补缺失值
data.fillna(data.mean(), inplace=True)
- 去除异常值
异常值是指明显偏离其他数据点的值。可以使用统计方法或可视化工具识别和去除异常值。
import numpy as np
使用z-score识别异常值
from scipy import stats
z_scores = np.abs(stats.zscore(data))
data = data[(z_scores < 3).all(axis=1)]
三、构建并训练机器学习模型
为了分析熊市,我们可以使用机器学习模型进行预测和分类。常用的机器学习模型包括线性回归、随机森林、支持向量机等。
- 特征工程
特征工程是指从原始数据中提取有用特征,以提高模型的性能。常见的特征包括技术指标(如移动平均线、相对强弱指数等)、宏观经济指标等。
# 计算移动平均线
data['SMA_50'] = data['Close'].rolling(window=50).mean()
data['SMA_200'] = data['Close'].rolling(window=200).mean()
计算相对强弱指数
import talib
data['RSI'] = talib.RSI(data['Close'])
- 选择模型
根据需求选择合适的机器学习模型。可以使用Scikit-learn库来构建和训练模型。
from sklearn.model_selection import train_test_split
from sklearn.ensemble import RandomForestClassifier
from sklearn.metrics import classification_report
特征和标签
X = data[['SMA_50', 'SMA_200', 'RSI']].dropna()
y = (data['Close'].shift(-1) < data['Close']).astype(int).dropna()
划分训练集和测试集
X_train, X_test, y_train, y_test = train_test_split(X, y, test_size=0.2, random_state=42)
构建并训练随机森林模型
model = RandomForestClassifier(n_estimators=100, random_state=42)
model.fit(X_train, y_train)
预测并评估模型
y_pred = model.predict(X_test)
print(classification_report(y_test, y_pred))
四、可视化分析
可视化是数据分析的重要环节,通过图表可以直观地展示数据和分析结果。常用的可视化工具有Matplotlib、Seaborn、Plotly等。
- 绘制时间序列图
时间序列图可以展示股票价格的变化趋势,帮助识别熊市。
import matplotlib.pyplot as plt
plt.figure(figsize=(14, 7))
plt.plot(data['Close'], label='Close Price')
plt.plot(data['SMA_50'], label='50-Day SMA')
plt.plot(data['SMA_200'], label='200-Day SMA')
plt.title('Stock Price with Moving Averages')
plt.xlabel('Date')
plt.ylabel('Price')
plt.legend()
plt.show()
- 绘制技术指标图
技术指标图可以展示技术分析的结果,帮助识别买卖信号。
plt.figure(figsize=(14, 7))
plt.plot(data['RSI'], label='RSI')
plt.axhline(y=70, color='r', linestyle='--')
plt.axhline(y=30, color='g', linestyle='--')
plt.title('Relative Strength Index (RSI)')
plt.xlabel('Date')
plt.ylabel('RSI')
plt.legend()
plt.show()
五、模型评估与优化
模型评估是确保模型性能的重要步骤。常用的评估指标包括准确率、召回率、F1分数等。可以通过交叉验证、调参等方法优化模型。
- 交叉验证
交叉验证可以有效评估模型的泛化能力,减少过拟合。
from sklearn.model_selection import cross_val_score
交叉验证
scores = cross_val_score(model, X, y, cv=5)
print('Cross-validation scores:', scores)
print('Mean cross-validation score:', scores.mean())
- 调参
调参是通过调整模型参数提高模型性能的过程。可以使用网格搜索或随机搜索进行调参。
from sklearn.model_selection import GridSearchCV
定义参数网格
param_grid = {
'n_estimators': [50, 100, 200],
'max_depth': [None, 10, 20, 30]
}
网格搜索
grid_search = GridSearchCV(model, param_grid, cv=5)
grid_search.fit(X_train, y_train)
最优参数
print('Best parameters:', grid_search.best_params_)
六、实战案例
接下来,我们将通过一个实战案例来综合运用上述技术,分析某股票的熊市。
- 数据收集与清洗
ticker = 'AAPL'
data = yf.download(ticker, start="2010-01-01", end="2023-10-01")
data.dropna(inplace=True)
- 特征工程
data['SMA_50'] = data['Close'].rolling(window=50).mean()
data['SMA_200'] = data['Close'].rolling(window=200).mean()
data['RSI'] = talib.RSI(data['Close'])
data.dropna(inplace=True)
- 构建并训练模型
X = data[['SMA_50', 'SMA_200', 'RSI']]
y = (data['Close'].shift(-1) < data['Close']).astype(int)
X_train, X_test, y_train, y_test = train_test_split(X, y, test_size=0.2, random_state=42)
model = RandomForestClassifier(n_estimators=100, random_state=42)
model.fit(X_train, y_train)
y_pred = model.predict(X_test)
print(classification_report(y_test, y_pred))
- 可视化分析
plt.figure(figsize=(14, 7))
plt.plot(data['Close'], label='Close Price')
plt.plot(data['SMA_50'], label='50-Day SMA')
plt.plot(data['SMA_200'], label='200-Day SMA')
plt.title('Stock Price with Moving Averages')
plt.xlabel('Date')
plt.ylabel('Price')
plt.legend()
plt.show()
plt.figure(figsize=(14, 7))
plt.plot(data['RSI'], label='RSI')
plt.axhline(y=70, color='r', linestyle='--')
plt.axhline(y=30, color='g', linestyle='--')
plt.title('Relative Strength Index (RSI)')
plt.xlabel('Date')
plt.ylabel('RSI')
plt.legend()
plt.show()
- 模型评估与优化
scores = cross_val_score(model, X, y, cv=5)
print('Cross-validation scores:', scores)
print('Mean cross-validation score:', scores.mean())
param_grid = {
'n_estimators': [50, 100, 200],
'max_depth': [None, 10, 20, 30]
}
grid_search = GridSearchCV(model, param_grid, cv=5)
grid_search.fit(X_train, y_train)
print('Best parameters:', grid_search.best_params_)
通过上述步骤,我们可以利用Python进行全面的熊市分析,从数据收集、清洗,到特征工程、模型训练,再到可视化分析和模型评估,帮助我们更好地理解和预测熊市的走势。
相关问答FAQs:
熊市的定义是什么,如何识别一个熊市?
熊市通常指的是市场价格下降20%或更多的情况,持续时间通常超过两个月。识别熊市可以通过观察主要股指的走势、市场情绪指标以及经济指标的变化来实现。通过Python,可以分析历史数据,计算股指的变化百分比,绘制图表,帮助可视化趋势,进而判断市场是否进入熊市。
使用Python进行熊市分析需要哪些库?
进行熊市分析常用的Python库包括Pandas、NumPy、Matplotlib和Seaborn等。Pandas可以处理和分析时间序列数据,NumPy用于数值计算,Matplotlib和Seaborn则可以帮助绘制可视化图表。此外,使用yfinance库可以轻松获取股票市场的数据,便于进行深入分析。
在熊市中,Python分析可以提供哪些具体的投资建议?
Python分析可以帮助投资者识别风险和机会。例如,通过分析不同资产类别在熊市中的表现,投资者可以决定是否进行资产配置调整。此外,通过历史数据分析,投资者可以发现某些股票在熊市期间的表现模式,从而制定相应的投资策略,比如选择抗跌性强的股票或者在市场低迷时进行逢低买入。
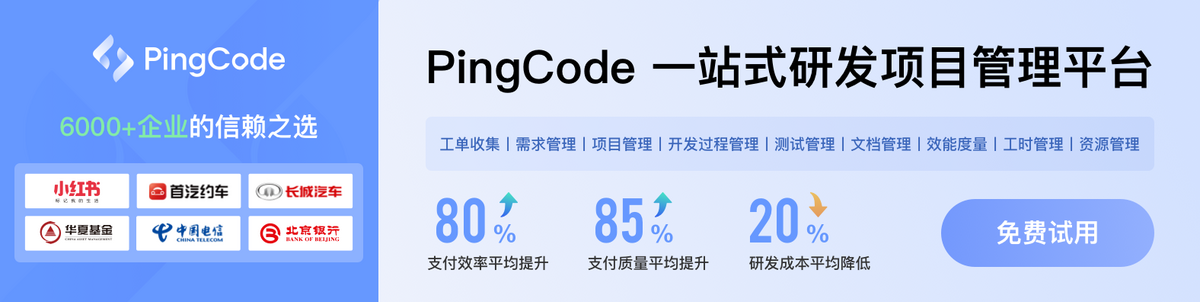