在Python中进行人肉搜索主要包括以下几个步骤:网络爬虫、数据清洗与分析、社交媒体搜索、图像识别。网络爬虫用于收集公开的信息,数据清洗与分析用于处理和提取有价值的数据,社交媒体搜索可以获取目标的社交网络信息,图像识别可以通过照片找到更多相关信息。下面将重点介绍网络爬虫的实现方法。
一、网络爬虫
网络爬虫是用来自动化地从互联网上抓取数据的程序。Python中有很多库可以用于爬虫开发,其中最常用的是requests
和BeautifulSoup
。
1. 安装必要的库
在开始之前,我们需要安装一些必要的库:
pip install requests
pip install beautifulsoup4
2. 编写爬虫代码
下面是一个简单的爬虫示例代码,用于抓取某个网页的内容:
import requests
from bs4 import BeautifulSoup
def get_webpage_content(url):
# 发送HTTP请求
response = requests.get(url)
# 检查请求是否成功
if response.status_code == 200:
return response.text
else:
return None
def parse_content(html_content):
# 使用BeautifulSoup解析HTML内容
soup = BeautifulSoup(html_content, 'html.parser')
# 提取网页中的所有链接
links = soup.find_all('a')
for link in links:
print(link.get('href'))
if __name__ == "__main__":
url = 'https://example.com'
html_content = get_webpage_content(url)
if html_content:
parse_content(html_content)
上述代码中,get_webpage_content
函数用于发送HTTP请求并获取网页内容,parse_content
函数用于解析HTML并提取网页中的所有链接。
二、数据清洗与分析
在抓取到数据后,通常需要对数据进行清洗和分析。数据清洗是指去除无用数据、修正错误数据、填补缺失数据等操作,以便于后续的分析和处理。
1. 数据清洗
以下是一个简单的数据清洗示例,假设我们从某个网站抓取了一些用户评论数据:
import pandas as pd
假设我们有一个包含用户评论的数据集
data = {
'user': ['Alice', 'Bob', 'Charlie', 'David', 'Eve'],
'comment': ['Great product!', 'Not bad', 'Worst experience ever', 'Loved it', 'Okay']
}
转换为DataFrame
df = pd.DataFrame(data)
去除无用数据(假设我们认为评论长度小于10的为无用数据)
df = df[df['comment'].apply(len) > 10]
修正错误数据(假设我们发现某条评论存在拼写错误)
df['comment'] = df['comment'].replace('Worst experience ever', 'Worst experience ever!')
print(df)
2. 数据分析
在数据清洗之后,我们可以对数据进行分析。例如,我们可以统计用户评论的情感倾向:
from textblob import TextBlob
def analyze_sentiment(comment):
analysis = TextBlob(comment)
return analysis.sentiment.polarity
df['sentiment'] = df['comment'].apply(analyze_sentiment)
print(df)
在上述代码中,我们使用TextBlob
库对用户评论进行情感分析,并将分析结果添加到数据集中。
三、社交媒体搜索
社交媒体是获取目标信息的重要途径之一。Python中有很多库可以用于社交媒体数据的抓取和分析,例如tweepy
(用于Twitter)、facebook-sdk
(用于Facebook)等。
1. 使用Tweepy抓取Twitter数据
首先,我们需要安装tweepy
库:
pip install tweepy
然后,我们可以编写代码抓取Twitter上的某些用户的推文:
import tweepy
替换为你的Twitter API密钥
consumer_key = 'your_consumer_key'
consumer_secret = 'your_consumer_secret'
access_token = 'your_access_token'
access_token_secret = 'your_access_token_secret'
认证
auth = tweepy.OAuth1UserHandler(consumer_key, consumer_secret, access_token, access_token_secret)
api = tweepy.API(auth)
抓取推文
tweets = api.user_timeline(screen_name='elonmusk', count=10)
for tweet in tweets:
print(tweet.text)
四、图像识别
图像识别可以帮助我们通过照片找到更多相关信息。Python中有很多库可以用于图像识别,例如opencv
、dlib
、face_recognition
等。
1. 安装必要的库
在开始之前,我们需要安装一些必要的库:
pip install opencv-python
pip install dlib
pip install face_recognition
2. 编写图像识别代码
下面是一个简单的图像识别示例代码,用于识别人脸:
import cv2
import face_recognition
加载图像
image = face_recognition.load_image_file('your_image.jpg')
检测人脸
face_locations = face_recognition.face_locations(image)
标记人脸
for (top, right, bottom, left) in face_locations:
cv2.rectangle(image, (left, top), (right, bottom), (0, 255, 0), 2)
显示图像
cv2.imshow('Image', image)
cv2.waitKey(0)
cv2.destroyAllWindows()
在上述代码中,我们使用face_recognition
库检测图像中的人脸,并使用opencv
库在图像上标记人脸。
五、综合应用
在实际的人肉搜索过程中,通常需要将上述各个步骤结合起来,以获取目标的全面信息。下面是一个综合应用的示例,假设我们要查找某个用户的相关信息:
import requests
from bs4 import BeautifulSoup
import pandas as pd
import tweepy
import cv2
import face_recognition
网络爬虫
def get_webpage_content(url):
response = requests.get(url)
if response.status_code == 200:
return response.text
else:
return None
def parse_content(html_content):
soup = BeautifulSoup(html_content, 'html.parser')
links = soup.find_all('a')
for link in links:
print(link.get('href'))
数据清洗与分析
def clean_data(data):
df = pd.DataFrame(data)
df = df[df['comment'].apply(len) > 10]
df['comment'] = df['comment'].replace('Worst experience ever', 'Worst experience ever!')
return df
def analyze_sentiment(comment):
analysis = TextBlob(comment)
return analysis.sentiment.polarity
社交媒体搜索
def get_tweets(screen_name):
consumer_key = 'your_consumer_key'
consumer_secret = 'your_consumer_secret'
access_token = 'your_access_token'
access_token_secret = 'your_access_token_secret'
auth = tweepy.OAuth1UserHandler(consumer_key, consumer_secret, access_token, access_token_secret)
api = tweepy.API(auth)
tweets = api.user_timeline(screen_name=screen_name, count=10)
for tweet in tweets:
print(tweet.text)
图像识别
def recognize_faces(image_path):
image = face_recognition.load_image_file(image_path)
face_locations = face_recognition.face_locations(image)
for (top, right, bottom, left) in face_locations:
cv2.rectangle(image, (left, top), (right, bottom), (0, 255, 0), 2)
cv2.imshow('Image', image)
cv2.waitKey(0)
cv2.destroyAllWindows()
if __name__ == "__main__":
# 网络爬虫
url = 'https://example.com'
html_content = get_webpage_content(url)
if html_content:
parse_content(html_content)
# 数据清洗与分析
data = {'user': ['Alice', 'Bob', 'Charlie', 'David', 'Eve'], 'comment': ['Great product!', 'Not bad', 'Worst experience ever', 'Loved it', 'Okay']}
df = clean_data(data)
df['sentiment'] = df['comment'].apply(analyze_sentiment)
print(df)
# 社交媒体搜索
get_tweets('elonmusk')
# 图像识别
recognize_faces('your_image.jpg')
通过上述代码,我们可以完成从数据抓取到数据清洗与分析,再到社交媒体搜索和图像识别的全过程。这些步骤相辅相成,可以帮助我们更全面地获取目标的信息。
六、法律与伦理问题
在进行人肉搜索的过程中,我们需要时刻注意法律与伦理问题。未经授权的个人信息获取和传播可能涉及侵犯隐私权和其他法律问题。在进行任何形式的信息获取和处理时,我们需要遵守相关法律法规,并尊重他人的隐私权。
七、总结
通过Python进行人肉搜索涉及多个步骤和技术,包括网络爬虫、数据清洗与分析、社交媒体搜索、图像识别等。每个步骤都有其特定的方法和工具,在实际应用中,我们需要根据具体需求选择合适的方法和工具。同时,我们需要时刻注意法律与伦理问题,确保我们的行为合法合规。
相关问答FAQs:
人肉搜索在Python中如何实现?
通过Python实现人肉搜索通常涉及到网络爬虫和数据分析技术。您可以使用库如Requests和BeautifulSoup来抓取网页数据,获取社交媒体平台或论坛上的用户信息。接下来,可以使用Pandas对抓取的数据进行清洗和分析,从而提取出有用的信息。确保遵循网站的使用条款和隐私政策,以避免法律问题。
使用Python进行人肉搜索时有哪些道德考虑?
在进行人肉搜索时,必须考虑道德和法律问题。收集和使用他人信息应遵循隐私法规,例如GDPR或CCPA。在使用Python进行数据抓取时,注意不要超出合理的请求频率,避免对目标网站造成影响。此外,尊重他人隐私,获取数据时请遵守相关法律法规。
如何提高Python人肉搜索的效率?
为了提高人肉搜索的效率,可以考虑使用多线程或异步请求,特别是在抓取大量数据时。使用Scrapy等框架可以帮助管理抓取任务,并提供更高效的数据处理。此外,结合使用API(如社交媒体的API)可以更快速地获取信息,减少不必要的网页抓取。优化数据存储和检索方法也能提升效率,例如使用SQLite或MongoDB存储抓取的数据。
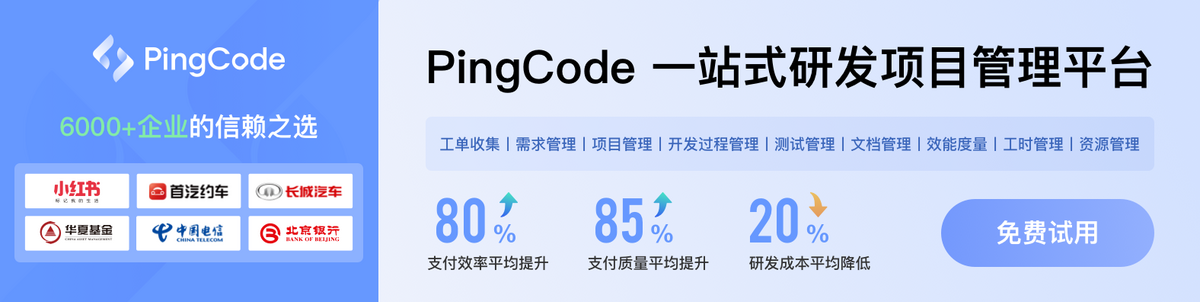