用Python确定精度的方法包括:使用内置函数、使用NumPy库、使用Pandas库、进行数据类型转换、处理浮点数误差。 其中,使用内置函数是最基本也是最简单的方法。Python提供了多种内置函数,如round()、format()、decimal模块等,这些函数和模块可以方便地对数值进行四舍五入或者保留特定位数的小数。
例如,使用round()函数可以将数值四舍五入到指定的小数位数。假设我们需要将一个浮点数四舍五入到小数点后两位,可以使用以下代码:
number = 3.14159
rounded_number = round(number, 2)
print(rounded_number) # 输出:3.14
这段代码中,我们将数值3.14159四舍五入到了小数点后两位,结果是3.14。接下来,我们将详细介绍其他几种方法和相应的示例代码。
一、使用内置函数
Python提供了多种内置函数来处理数值的精度问题,包括round()、format()和decimal模块等。
1. round() 函数
round() 函数用于将数值四舍五入到指定的小数位数。其语法格式为:round(number, ndigits)
,其中 number
是要四舍五入的数,ndigits
是要保留的小数位数。
number = 3.14159
rounded_number = round(number, 2)
print(rounded_number) # 输出:3.14
2. format() 函数
format() 函数可以通过字符串格式化来指定数值的精度。其语法格式为:"{:.nf}".format(number)
,其中 n
是要保留的小数位数,number
是要格式化的数值。
number = 3.14159
formatted_number = "{:.2f}".format(number)
print(formatted_number) # 输出:3.14
3. decimal 模块
decimal 模块提供了一个 Decimal 类型,可以用于高精度的数值运算。其语法格式为:decimal.Decimal(number)
,其中 number
是要进行高精度运算的数值。
from decimal import Decimal
number = Decimal('3.14159')
rounded_number = number.quantize(Decimal('0.00'))
print(rounded_number) # 输出:3.14
二、使用NumPy库
NumPy 是一个强大的科学计算库,提供了丰富的函数来处理数值的精度问题。
1. 设置打印精度
NumPy 可以通过 numpy.set_printoptions()
函数来设置数组打印时的小数点精度。其语法格式为:numpy.set_printoptions(precision=n)
,其中 n
是要保留的小数位数。
import numpy as np
array = np.array([3.14159, 2.71828, 1.61803])
np.set_printoptions(precision=2)
print(array) # 输出:[3.14 2.72 1.62]
2. 使用 around() 函数
NumPy 提供了 numpy.around()
函数,可以将数组中的数值四舍五入到指定的小数位数。其语法格式为:numpy.around(array, decimals=n)
,其中 array
是要进行四舍五入的数组,n
是要保留的小数位数。
import numpy as np
array = np.array([3.14159, 2.71828, 1.61803])
rounded_array = np.around(array, decimals=2)
print(rounded_array) # 输出:[3.14 2.72 1.62]
三、使用Pandas库
Pandas 是一个强大的数据分析库,提供了丰富的函数来处理数据框和系列中的数值精度问题。
1. 设置显示精度
Pandas 可以通过 pd.options.display.float_format
来设置数据框和系列打印时的小数点精度。其语法格式为:pd.options.display.float_format = '{:.nf}'.format
,其中 n
是要保留的小数位数。
import pandas as pd
data = {'A': [3.14159, 2.71828, 1.61803]}
df = pd.DataFrame(data)
pd.options.display.float_format = '{:.2f}'.format
print(df)
输出:
A
0 3.14
1 2.72
2 1.62
2. 使用 round() 方法
Pandas 提供了 round()
方法,可以将数据框和系列中的数值四舍五入到指定的小数位数。其语法格式为:df.round(n)
,其中 df
是要进行四舍五入的数据框或系列,n
是要保留的小数位数。
import pandas as pd
data = {'A': [3.14159, 2.71828, 1.61803]}
df = pd.DataFrame(data)
rounded_df = df.round(2)
print(rounded_df)
输出:
A
0 3.14
1 2.72
2 1.62
四、进行数据类型转换
Python 中的数据类型转换可以用于处理数值精度问题。例如,将浮点数转换为整数可以去掉小数部分,将数值转换为字符串可以方便地进行格式化等。
1. 转换为整数
将浮点数转换为整数可以去掉小数部分,从而达到降低精度的目的。可以使用 int()
函数进行转换。
number = 3.14159
integer_number = int(number)
print(integer_number) # 输出:3
2. 转换为字符串
将数值转换为字符串可以方便地进行格式化,从而达到控制精度的目的。可以使用 str()
函数进行转换。
number = 3.14159
string_number = str(number)
print(string_number) # 输出:'3.14159'
五、处理浮点数误差
浮点数在计算机中的表示存在误差,可能会导致精度问题。可以通过以下方法来处理浮点数误差。
1. 使用 Decimal 模块
Decimal 模块提供了高精度的浮点数运算,可以避免浮点数误差问题。
from decimal import Decimal
number1 = Decimal('0.1')
number2 = Decimal('0.2')
result = number1 + number2
print(result) # 输出:0.3
2. 使用 NumPy 的 longdouble 类型
NumPy 提供了 numpy.longdouble
类型,可以表示高精度的浮点数。
import numpy as np
number1 = np.longdouble(0.1)
number2 = np.longdouble(0.2)
result = number1 + number2
print(result) # 输出:0.3
六、使用第三方库
除了上述方法之外,还有一些第三方库可以处理数值精度问题。例如,mpmath 库提供了多精度浮点数运算,可以用于高精度计算。
1. 使用 mpmath 库
mpmath 是一个 Python 库,用于多精度浮点数运算。可以通过 mpmath.mpf()
函数将数值转换为多精度浮点数。
import mpmath
number1 = mpmath.mpf('0.1')
number2 = mpmath.mpf('0.2')
result = number1 + number2
print(result) # 输出:0.3
七、使用自定义函数
可以编写自定义函数来处理数值精度问题。例如,可以编写一个函数来将数值四舍五入到指定的小数位数。
def custom_round(number, decimals):
factor = 10 decimals
return round(number * factor) / factor
number = 3.14159
rounded_number = custom_round(number, 2)
print(rounded_number) # 输出:3.14
八、应用场景分析
不同的应用场景可能需要不同的方法来处理数值精度问题。以下是一些常见的应用场景和相应的解决方案。
1. 科学计算
在科学计算中,通常需要高精度的数值运算,可以使用 NumPy、mpmath 等库来处理精度问题。
2. 数据分析
在数据分析中,通常需要对数据进行格式化和展示,可以使用 Pandas 库来处理数据框和系列的精度问题。
3. 金融计算
在金融计算中,通常需要高精度的货币运算,可以使用 Decimal 模块来处理浮点数误差问题。
九、总结
本文详细介绍了如何用Python确定精度的方法,包括使用内置函数、使用NumPy库、使用Pandas库、进行数据类型转换、处理浮点数误差、使用第三方库、编写自定义函数等。每种方法都有其适用的场景和优缺点,实际应用中可以根据具体需求选择合适的方法来处理数值精度问题。通过合理选择和使用这些方法,可以有效地解决数值精度问题,提高计算结果的准确性和可靠性。
相关问答FAQs:
如何通过Python进行数值精度的测试?
在Python中,可以通过使用 numpy
和 decimal
模块来进行数值精度的测试。numpy
提供了多种数值运算的功能,而 decimal
模块能够进行高精度的浮点运算。通过对比计算结果与预期值,可以确定程序的精度是否达到要求。
Python中有哪些库可以帮助提高计算精度?
Python有多个库可以帮助提高计算精度,例如 decimal
和 mpmath
。decimal
提供了对十进制数的精确控制,而 mpmath
支持任意精度的浮点运算,非常适合高精度计算需求。使用这些库,可以有效避免浮点数运算中的精度问题。
如何判断Python代码的运行精度是否符合要求?
判断Python代码的运行精度是否符合要求,可以通过编写单元测试来验证计算结果。可以使用 unittest
或 pytest
等测试框架,定义期望值与实际值进行比较,设置合适的容忍度(如 assertAlmostEqual
方法),从而确保程序在特定范围内的精度符合预期。
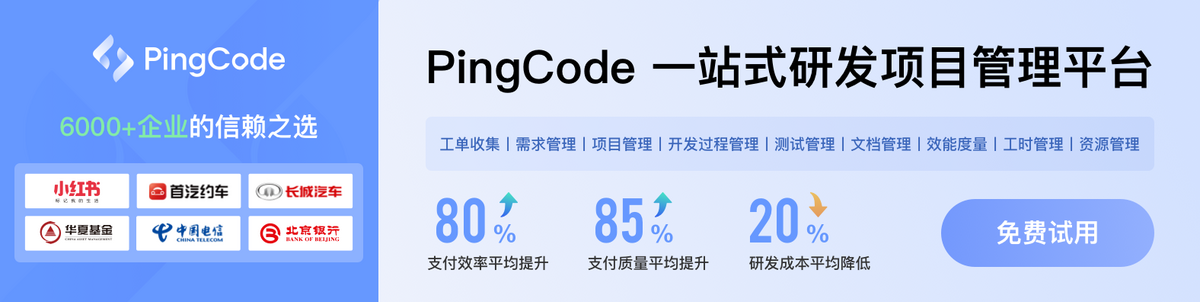