使用Python查重的方法有:哈希算法、编辑距离、TF-IDF算法、Simhash算法。其中,哈希算法是一种常见且高效的方法,可以快速检测文件或文本的重复。哈希算法通过将文本内容转换为固定长度的哈希值来比较不同文本之间的相似度。如果两个文本的哈希值相同,那么它们的内容很可能是相同的。接下来,我们详细介绍如何使用哈希算法进行查重。
一、哈希算法
哈希算法是通过计算文本的哈希值来判断文本是否重复的。哈希值是一个固定长度的字符串,它是根据文本内容计算出来的。如果两个文本的哈希值相同,那么这两个文本的内容很可能是相同的。
1.1 使用MD5哈希算法
MD5(Message-Digest Algorithm 5)是一种广泛使用的哈希函数,可以将任意长度的输入转换为固定长度的输出(128位)。下面是一个使用MD5哈希算法进行文本查重的示例:
import hashlib
def get_md5_hash(text):
md5 = hashlib.md5()
md5.update(text.encode('utf-8'))
return md5.hexdigest()
def check_duplicates(text_list):
hash_set = set()
for text in text_list:
hash_value = get_md5_hash(text)
if hash_value in hash_set:
print(f"Duplicate found: {text}")
else:
hash_set.add(hash_value)
示例文本列表
texts = ["hello world", "example text", "hello world", "another example"]
check_duplicates(texts)
在这个示例中,get_md5_hash
函数用于计算文本的MD5哈希值,check_duplicates
函数用于检测文本列表中的重复项。如果某个文本的哈希值已经在哈希集合中存在,那么该文本就是重复的。
1.2 使用SHA-256哈希算法
SHA-256(Secure Hash Algorithm 256-bit)是一种更安全的哈希函数,可以将任意长度的输入转换为256位的输出。下面是一个使用SHA-256哈希算法进行文本查重的示例:
import hashlib
def get_sha256_hash(text):
sha256 = hashlib.sha256()
sha256.update(text.encode('utf-8'))
return sha256.hexdigest()
def check_duplicates(text_list):
hash_set = set()
for text in text_list:
hash_value = get_sha256_hash(text)
if hash_value in hash_set:
print(f"Duplicate found: {text}")
else:
hash_set.add(hash_value)
示例文本列表
texts = ["hello world", "example text", "hello world", "another example"]
check_duplicates(texts)
在这个示例中,get_sha256_hash
函数用于计算文本的SHA-256哈希值,check_duplicates
函数用于检测文本列表中的重复项。如果某个文本的哈希值已经在哈希集合中存在,那么该文本就是重复的。
二、编辑距离
编辑距离(Edit Distance)是指将一个字符串转换为另一个字符串所需的最少编辑操作次数。常见的编辑操作包括插入、删除和替换字符。编辑距离越小,两个字符串的相似度越高。Levenshtein距离是一种常用的编辑距离算法。
2.1 计算Levenshtein距离
下面是一个计算Levenshtein距离的示例:
def levenshtein_distance(s1, s2):
if len(s1) < len(s2):
return levenshtein_distance(s2, s1)
if len(s2) == 0:
return len(s1)
previous_row = range(len(s2) + 1)
for i, c1 in enumerate(s1):
current_row = [i + 1]
for j, c2 in enumerate(s2):
insertions = previous_row[j + 1] + 1
deletions = current_row[j] + 1
substitutions = previous_row[j] + (c1 != c2)
current_row.append(min(insertions, deletions, substitutions))
previous_row = current_row
return previous_row[-1]
示例
s1 = "kitten"
s2 = "sitting"
distance = levenshtein_distance(s1, s2)
print(f"Levenshtein distance between '{s1}' and '{s2}' is {distance}")
在这个示例中,levenshtein_distance
函数用于计算两个字符串之间的Levenshtein距离。该函数使用动态规划的方法,通过逐字符比较两个字符串来计算最小编辑操作次数。
2.2 查找相似文本
我们可以使用Levenshtein距离来查找文本列表中相似的文本:
def find_similar_texts(text_list, threshold):
similar_texts = []
for i in range(len(text_list)):
for j in range(i + 1, len(text_list)):
distance = levenshtein_distance(text_list[i], text_list[j])
if distance <= threshold:
similar_texts.append((text_list[i], text_list[j], distance))
return similar_texts
示例文本列表
texts = ["kitten", "sitting", "bitten", "betting"]
threshold = 3
similar_texts = find_similar_texts(texts, threshold)
for text1, text2, distance in similar_texts:
print(f"Similar texts: '{text1}' and '{text2}' with distance {distance}")
在这个示例中,find_similar_texts
函数用于查找文本列表中Levenshtein距离小于或等于给定阈值的文本对。该函数通过两两比较文本来查找相似的文本。
三、TF-IDF算法
TF-IDF(Term Frequency-Inverse Document Frequency)是一种常用的文本相似度计算方法。TF-IDF算法通过计算词频和逆文档频率来衡量词语在文档中的重要性。余弦相似度(Cosine Similarity)常用于计算两个文本的相似度。
3.1 计算TF-IDF
我们可以使用scikit-learn
库来计算文本的TF-IDF值:
from sklearn.feature_extraction.text import TfidfVectorizer
def calculate_tfidf(text_list):
vectorizer = TfidfVectorizer()
tfidf_matrix = vectorizer.fit_transform(text_list)
return tfidf_matrix
示例文本列表
texts = ["the cat in the hat", "the quick brown fox", "the cat in the hat again"]
tfidf_matrix = calculate_tfidf(texts)
print(tfidf_matrix.toarray())
在这个示例中,calculate_tfidf
函数使用TfidfVectorizer
类来计算文本列表的TF-IDF值。tfidf_matrix
是一个稀疏矩阵,表示每个文本的TF-IDF值。
3.2 计算余弦相似度
我们可以使用余弦相似度来比较两个文本的TF-IDF值:
from sklearn.metrics.pairwise import cosine_similarity
def calculate_cosine_similarity(tfidf_matrix):
cosine_sim = cosine_similarity(tfidf_matrix)
return cosine_sim
示例文本列表
texts = ["the cat in the hat", "the quick brown fox", "the cat in the hat again"]
tfidf_matrix = calculate_tfidf(texts)
cosine_sim = calculate_cosine_similarity(tfidf_matrix)
print(cosine_sim)
在这个示例中,calculate_cosine_similarity
函数使用cosine_similarity
函数来计算TF-IDF矩阵的余弦相似度。cosine_sim
是一个相似度矩阵,表示每对文本之间的相似度。
3.3 查找相似文本
我们可以使用余弦相似度来查找文本列表中相似的文本:
def find_similar_texts(text_list, threshold):
tfidf_matrix = calculate_tfidf(text_list)
cosine_sim = calculate_cosine_similarity(tfidf_matrix)
similar_texts = []
for i in range(len(text_list)):
for j in range(i + 1, len(text_list)):
if cosine_sim[i, j] >= threshold:
similar_texts.append((text_list[i], text_list[j], cosine_sim[i, j]))
return similar_texts
示例文本列表
texts = ["the cat in the hat", "the quick brown fox", "the cat in the hat again"]
threshold = 0.5
similar_texts = find_similar_texts(texts, threshold)
for text1, text2, similarity in similar_texts:
print(f"Similar texts: '{text1}' and '{text2}' with similarity {similarity}")
在这个示例中,find_similar_texts
函数用于查找文本列表中余弦相似度大于或等于给定阈值的文本对。该函数通过两两比较文本的余弦相似度来查找相似的文本。
四、Simhash算法
Simhash是一种用于近似文本查重的算法。它通过对文本进行哈希计算,生成一个指纹(fingerprint),然后通过比较指纹之间的汉明距离来判断文本的相似度。
4.1 计算Simhash值
我们可以使用simhash
库来计算文本的Simhash值:
from simhash import Simhash
def calculate_simhash(text):
return Simhash(text)
示例文本
text = "the quick brown fox jumps over the lazy dog"
simhash_value = calculate_simhash(text)
print(simhash_value.value)
在这个示例中,calculate_simhash
函数使用Simhash
类来计算文本的Simhash值。simhash_value.value
是一个整数,表示文本的Simhash指纹。
4.2 计算汉明距离
我们可以使用汉明距离来比较两个Simhash值的相似度:
def hamming_distance(hash1, hash2):
return bin(hash1 ^ hash2).count('1')
示例文本
text1 = "the quick brown fox jumps over the lazy dog"
text2 = "the quick brown fox jumps over the lazy cat"
simhash1 = calculate_simhash(text1)
simhash2 = calculate_simhash(text2)
distance = hamming_distance(simhash1.value, simhash2.value)
print(f"Hamming distance between '{text1}' and '{text2}' is {distance}")
在这个示例中,hamming_distance
函数用于计算两个Simhash值之间的汉明距离。汉明距离越小,两个文本的相似度越高。
4.3 查找相似文本
我们可以使用Simhash值和汉明距离来查找文本列表中相似的文本:
def find_similar_texts(text_list, threshold):
simhash_values = [calculate_simhash(text).value for text in text_list]
similar_texts = []
for i in range(len(text_list)):
for j in range(i + 1, len(text_list)):
distance = hamming_distance(simhash_values[i], simhash_values[j])
if distance <= threshold:
similar_texts.append((text_list[i], text_list[j], distance))
return similar_texts
示例文本列表
texts = ["the quick brown fox jumps over the lazy dog",
"the quick brown fox jumps over the lazy cat",
"the quick brown fox leaps over the lazy dog"]
threshold = 5
similar_texts = find_similar_texts(texts, threshold)
for text1, text2, distance in similar_texts:
print(f"Similar texts: '{text1}' and '{text2}' with distance {distance}")
在这个示例中,find_similar_texts
函数用于查找文本列表中汉明距离小于或等于给定阈值的文本对。该函数通过两两比较文本的Simhash值来查找相似的文本。
总结
使用Python进行文本查重可以通过多种方法实现,包括哈希算法、编辑距离、TF-IDF算法和Simhash算法。每种方法都有其优缺点,适用于不同的应用场景。
- 哈希算法:高效、简单,适用于精确查重。
- 编辑距离:适用于检测文本的细微差异,但计算复杂度较高。
- TF-IDF算法:适用于比较文本的相似度,尤其是长文本,计算复杂度适中。
- Simhash算法:适用于海量文本的近似查重,计算高效。
根据具体需求选择合适的查重方法,可以有效地提高查重效率和准确性。
相关问答FAQs:
如何用Python实现文件内容的查重?
要实现文件内容的查重,可以使用Python中的哈希函数来生成文件内容的唯一标识符。例如,可以读取文件内容,使用hashlib
库生成MD5或SHA-256哈希值,然后将这些哈希值存储在一个集合中。通过对比哈希值,可以有效判断文件是否重复。
Python查重有什么常用的库推荐?
对于查重任务,常用的库包括pandas
,适合处理数据表格中的重复项;difflib
,可以用于比较文本文件的相似性;以及hashlib
,用于生成文件或字符串的哈希值。每个库都有其特定的功能,选择合适的库可以提高查重效率。
在处理大数据时,如何优化Python查重的效率?
在处理大量数据时,可以考虑使用分块读取文件的方法,这样可以减少内存占用。同时,结合使用集合来存储已经处理过的项,以便快速查找重复内容。此外,使用多线程或多进程技术,可以显著提高查重的速度。
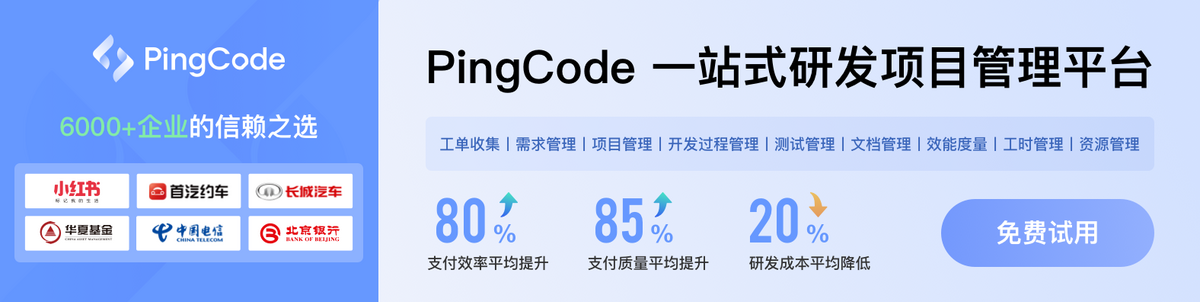