Python直接使用C4.5分类器的方法包括:利用现有的开源库、手动实现C4.5算法、集成到机器学习框架中。在这篇文章中,我们将深入探讨这几种方法,尤其是利用现有的开源库,这样可以节省大量的时间和精力。
一、利用现有的开源库
现有的开源库提供了现成的C4.5分类器实现,方便开发者直接调用,节省了重头构建算法的时间和精力。
1. Python库 sklearn
的限制
虽然 scikit-learn
是Python中使用最广泛的机器学习库之一,但它并未直接提供C4.5算法的实现。scikit-learn
中最接近C4.5的是 DecisionTreeClassifier
,它实现了CART算法。
2. 使用c45
库
一个常见的选择是使用 c45
库,这个库是对C4.5算法的Python实现。首先,你需要安装这个库:
pip install c45
安装完成后,你可以使用以下代码来构建和训练一个C4.5分类器:
import c45
加载数据集
data = c45.load_data('your_dataset.csv')
创建C4.5分类器
classifier = c45.C45()
训练分类器
classifier.train(data)
进行预测
predictions = classifier.predict(test_data)
这个库可以处理各种类型的数据,并且操作接口非常简洁。
二、手动实现C4.5算法
如果你对算法细节有深入了解,或者现有的库无法满足你的需求,你可以选择手动实现C4.5算法。
1. 数据处理
首先,你需要对数据进行预处理,包括处理缺失值、数值标准化和特征选择。
import pandas as pd
加载数据集
data = pd.read_csv('your_dataset.csv')
处理缺失值
data.fillna(data.mean(), inplace=True)
数值标准化
from sklearn.preprocessing import StandardScaler
scaler = StandardScaler()
data_scaled = scaler.fit_transform(data)
2. 信息增益计算
C4.5算法的核心是通过信息增益比来选择最佳分割点,这需要计算信息增益和分裂点的信息增益比。
import numpy as np
def information_gain(data, feature, target):
total_entropy = entropy(data[target])
values, counts = np.unique(data[feature], return_counts=True)
weighted_entropy = sum((counts[i] / np.sum(counts)) * entropy(data.where(data[feature] == values[i]).dropna()[target]) for i in range(len(values)))
info_gain = total_entropy - weighted_entropy
return info_gain
def entropy(column):
elements, counts = np.unique(column, return_counts=True)
entropy = -sum((counts[i]/np.sum(counts)) * np.log2(counts[i]/np.sum(counts)) for i in range(len(elements)))
return entropy
3. 构建决策树
通过递归方法构建决策树,每次选择信息增益比最高的特征进行分裂。
class DecisionTree:
def __init__(self, data, target):
self.data = data
self.target = target
self.tree = self.build_tree(data)
def build_tree(self, data, tree=None):
features = data.columns[:-1]
if len(np.unique(data[self.target])) == 1:
return np.unique(data[self.target])[0]
elif len(features) == 0:
return np.unique(data[self.target])[np.argmax(np.unique(data[self.target], return_counts=True)[1])]
else:
item_values = [information_gain(data, feature, self.target) for feature in features]
best_feature_index = np.argmax(item_values)
best_feature = features[best_feature_index]
tree = {best_feature: {}}
feature_values = np.unique(data[best_feature])
for value in feature_values:
sub_data = data.where(data[best_feature] == value).dropna()
subtree = self.build_tree(sub_data)
tree[best_feature][value] = subtree
return tree
使用决策树
dt = DecisionTree(data, 'target')
print(dt.tree)
三、集成到机器学习框架中
将C4.5算法集成到现有的机器学习框架中有助于提高开发效率,并可以利用框架提供的其他功能,如交叉验证、模型评估等。
1. 使用scikit-learn
与自定义算法结合
虽然 scikit-learn
不直接支持C4.5算法,但你可以通过自定义分类器并继承 BaseEstimator
和 ClassifierMixin
来实现。
from sklearn.base import BaseEstimator, ClassifierMixin
class C45Classifier(BaseEstimator, ClassifierMixin):
def __init__(self):
self.tree = None
def fit(self, X, y):
data = pd.DataFrame(X)
data['target'] = y
self.tree = DecisionTree(data, 'target').tree
return self
def predict(self, X):
predictions = []
for _, row in pd.DataFrame(X).iterrows():
predictions.append(self._predict_row(row))
return np.array(predictions)
def _predict_row(self, row):
tree = self.tree
while isinstance(tree, dict):
feature = next(iter(tree))
tree = tree[feature][row[feature]]
return tree
通过这种方式,你可以将C4.5分类器与 scikit-learn
的其他功能结合使用,如交叉验证和网格搜索。
四、结论
Python直接使用C4.5分类器的方法主要包括利用现有的开源库、手动实现C4.5算法和集成到机器学习框架中。这些方法各有优缺点,开发者可以根据具体需求选择合适的方法。通过现有的开源库可以快速实现,手动实现可以深入理解算法细节,集成到框架中可以提高开发效率并利用更多的功能。希望这篇文章能为你提供有价值的参考,帮助你在项目中成功应用C4.5分类器。
相关问答FAQs:
如何在Python中实现C4.5分类器的功能?
在Python中,虽然没有内置的C4.5分类器,但可以使用一些库来实现类似的功能。比如,scikit-learn
库提供了多种决策树算法,可以通过设置参数来模拟C4.5的行为。此外,也可以使用专门实现C4.5算法的库,如Orange3
或Liac-Arff
,来直接运行C4.5分类器。
C4.5分类器与其他分类算法相比,有什么优势?
C4.5分类器在处理缺失值和连续属性方面表现出色,能够生成可读性强的决策树。与一些其他分类算法相比,C4.5具有较好的性能,尤其是在特征选择和剪枝过程中,能够有效减少过拟合的风险。此外,C4.5还能够处理大规模数据集,适用于多种实际应用场景。
使用C4.5分类器时,如何优化模型性能?
优化C4.5分类器性能的方法包括特征选择、数据预处理和调整模型参数。特征选择可以通过去除冗余特征来提高模型效率。数据预处理如标准化和归一化可以改善模型的学习效果。此外,模型参数如树的深度和最小样本分裂数等也可以通过交叉验证等技术进行调优,以获得最佳的分类效果。
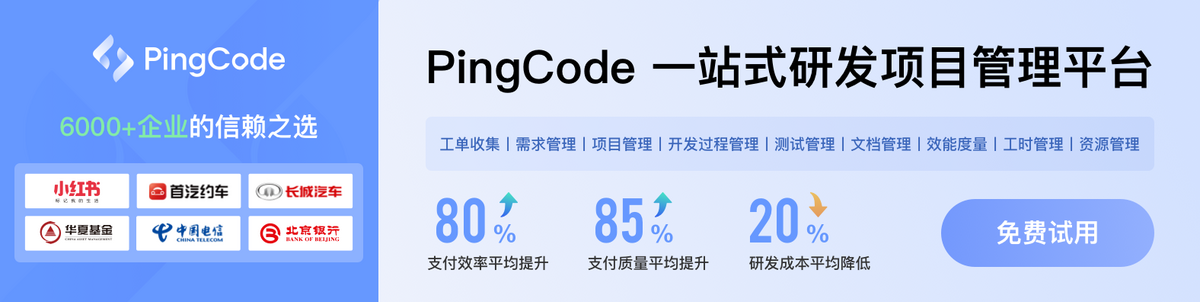