实现一个语音助手可以通过几个主要步骤完成:语音识别、自然语言处理、语音合成、集成与测试。其中,语音识别用于将语音转换为文本,自然语言处理用于理解用户意图,语音合成用于将文本转换回语音,集成与测试则是将所有组件组合起来并进行测试。接下来,我们将详细探讨这些步骤。
一、语音识别
语音识别是语音助手的核心功能之一。Python中有多个库可以实现语音识别功能,例如Google Speech API、CMU Sphinx和DeepSpeech等。
- Google Speech API
Google Speech API是一个广泛使用的语音识别服务,支持多种语言且识别准确率高。使用这一API,需要注册Google Cloud Platform并获取API密钥。通过安装SpeechRecognition
库,我们可以很方便地调用Google Speech API。
import speech_recognition as sr
def recognize_speech_from_mic():
recognizer = sr.Recognizer()
microphone = sr.Microphone()
with microphone as source:
print("Say something!")
audio = recognizer.listen(source)
try:
# 使用Google Speech API
text = recognizer.recognize_google(audio)
print("Google Speech Recognition thinks you said: " + text)
except sr.UnknownValueError:
print("Google Speech Recognition could not understand audio")
except sr.RequestError as e:
print("Could not request results from Google Speech Recognition service; {0}".format(e))
recognize_speech_from_mic()
- CMU Sphinx
CMU Sphinx是一个开源的语音识别工具包,适合在本地运行,不需要网络连接。它的优点是可以自定义词汇表和语言模型。安装pocketsphinx
库后,可以通过以下代码进行语音识别:
import speech_recognition as sr
def recognize_speech_from_mic():
recognizer = sr.Recognizer()
microphone = sr.Microphone()
with microphone as source:
print("Say something!")
audio = recognizer.listen(source)
try:
# 使用CMU Sphinx
text = recognizer.recognize_sphinx(audio)
print("Sphinx thinks you said: " + text)
except sr.UnknownValueError:
print("Sphinx could not understand audio")
except sr.RequestError as e:
print("Sphinx error; {0}".format(e))
recognize_speech_from_mic()
- DeepSpeech
DeepSpeech是Mozilla开发的一个基于深度学习的语音识别引擎,支持离线识别。需要预训练好的模型文件,并安装deepspeech
库。
import deepspeech
import numpy as np
import wave
def recognize_speech_from_file(filename):
model_file_path = 'deepspeech-models/deepspeech-0.9.3-models.pbmm'
scorer_file_path = 'deepspeech-models/deepspeech-0.9.3-models.scorer'
model = deepspeech.Model(model_file_path)
model.enableExternalScorer(scorer_file_path)
with wave.open(filename, 'rb') as w:
frames = w.getnframes()
buffer = w.readframes(frames)
data16 = np.frombuffer(buffer, dtype=np.int16)
text = model.stt(data16)
print("DeepSpeech thinks you said: " + text)
recognize_speech_from_file('your_audio_file.wav')
二、自然语言处理
自然语言处理(NLP)用于理解用户的意图。Python中有多个NLP库可供选择,如NLTK、spaCy和Transformers等。
- NLTK
NLTK是一个功能强大的自然语言处理库,提供了多种文本处理工具。
import nltk
from nltk.tokenize import word_tokenize
nltk.download('punkt')
def process_text(text):
tokens = word_tokenize(text)
print("Tokens:", tokens)
process_text("Hello, how can I help you today?")
- spaCy
spaCy是一个现代的、快速的自然语言处理库,支持多种语言。
import spacy
nlp = spacy.load("en_core_web_sm")
def process_text(text):
doc = nlp(text)
print("Tokens:", [token.text for token in doc])
process_text("Hello, how can I help you today?")
- Transformers
Transformers是Hugging Face提供的一个用于自然语言处理的库,支持预训练的深度学习模型。
from transformers import pipeline
def process_text(text):
nlp_pipeline = pipeline("sentiment-analysis")
result = nlp_pipeline(text)
print("Sentiment:", result)
process_text("I love using Python for data science!")
三、语音合成
语音合成用于将文本转换为语音。Python中常用的库包括gTTS和pyttsx3等。
- gTTS
gTTS(Google Text-to-Speech)是一个简单易用的语音合成库,支持多种语言。
from gtts import gTTS
import os
def text_to_speech(text):
tts = gTTS(text=text, lang='en')
tts.save("output.mp3")
os.system("start output.mp3")
text_to_speech("Hello, how can I assist you today?")
- pyttsx3
pyttsx3是一个离线语音合成库,支持多平台。
import pyttsx3
def text_to_speech(text):
engine = pyttsx3.init()
engine.say(text)
engine.runAndWait()
text_to_speech("Hello, how can I assist you today?")
四、集成与测试
在完成语音识别、自然语言处理和语音合成的单独实现后,下一步是将这些组件集成到一个完整的语音助手中,并进行测试。
- 集成
集成时,需要设计一个主程序来协调各个组件之间的交互。例如,可以设计一个循环,持续监听用户的语音输入,通过语音识别将其转换为文本,使用自然语言处理理解文本内容,然后根据理解的意图进行相应的操作,最后通过语音合成将响应内容读出来。
import speech_recognition as sr
import pyttsx3
def recognize_speech_and_respond():
recognizer = sr.Recognizer()
microphone = sr.Microphone()
engine = pyttsx3.init()
while True:
with microphone as source:
print("Listening...")
audio = recognizer.listen(source)
try:
text = recognizer.recognize_google(audio)
print("You said: " + text)
# 处理和响应用户输入的代码
response = process_text_and_get_response(text)
engine.say(response)
engine.runAndWait()
except sr.UnknownValueError:
print("Sorry, I did not understand that.")
engine.say("Sorry, I did not understand that.")
engine.runAndWait()
except sr.RequestError:
print("Could not request results from speech recognition service.")
engine.say("Could not request results from speech recognition service.")
engine.runAndWait()
def process_text_and_get_response(text):
# 简单示例:根据用户输入返回固定的响应
if "hello" in text.lower():
return "Hello! How can I help you today?"
elif "bye" in text.lower():
return "Goodbye! Have a great day!"
else:
return "I'm not sure how to respond to that."
recognize_speech_and_respond()
- 测试
测试语音助手的各个功能模块,确保它们能够正常工作。测试时,可以从以下几个方面入手:
- 准确性:检查语音识别和自然语言处理的准确性,确保输入的语音能够被准确识别和理解。
- 响应速度:测试语音助手的响应速度,确保其在合理时间内作出响应。
- 用户体验:评估语音助手的用户体验,确保其交互方式自然流畅。
- 异常处理:测试异常情况的处理能力,如识别错误或网络故障等。
通过以上步骤,可以构建一个基础的Python语音助手。进一步的优化和功能扩展可以包括:集成更多功能模块(如天气查询、日程管理等)、提高语音识别和自然语言处理的准确性、改进用户界面设计等。不断的迭代和改进,将有助于提升语音助手的性能和用户体验。
相关问答FAQs:
如何开始使用Python创建一个语音助手?
要开始使用Python创建语音助手,您需要安装一些基本的库,例如SpeechRecognition、pyttsx3和pyaudio。这些库分别负责语音识别、文本转语音和音频输入。安装后,您可以编写一个简单的程序,使用麦克风捕捉用户的声音,并将其转换为文本,然后通过合成语音回应用户。
Python语音助手能实现哪些功能?
Python语音助手可以实现多种功能,包括但不限于:发送邮件、设置提醒、查询天气、播放音乐、搜索互联网和控制智能家居设备。通过集成第三方API和服务,您可以不断扩展助手的功能,使其更加智能和实用。
如何提高语音助手的识别准确性?
提高语音助手的识别准确性可以通过多种方式实现。首先,确保使用高质量的麦克风,以减少背景噪音。其次,调整语音识别库的参数,如设置语言模型和噪声抑制。此外,定期更新语音识别模型和算法也是提升准确性的有效方法。您还可以通过训练助手识别特定用户的语音来优化体验。
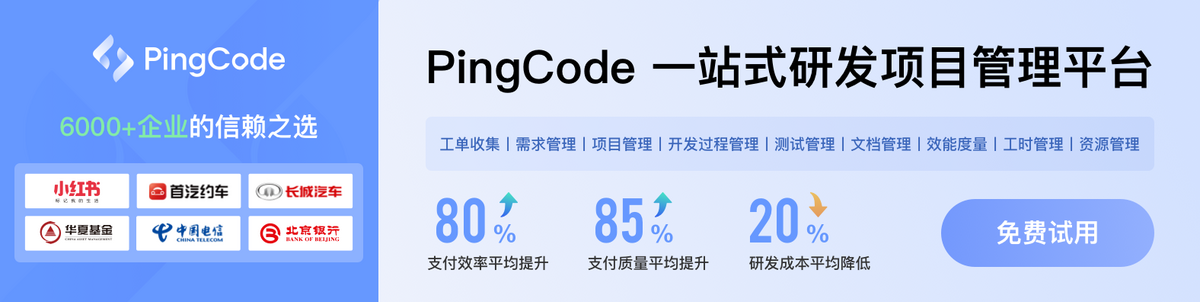