在Python中,构建决策树的节点可以通过几个关键步骤来实现:使用现有库如Scikit-learn、手动实现算法、选择分裂点、递归地构建树结构。使用现有库如Scikit-learn、手动实现算法、选择分裂点、递归地构建树结构。其中,使用现有库如Scikit-learn是最为简单且高效的方法。
一、使用现有库如Scikit-learn
在Python中,Scikit-learn库提供了便捷的方法来构建和使用决策树。以下是如何通过Scikit-learn构建决策树的节点:
- 安装并导入库
首先,需要确保已经安装了Scikit-learn库。如果没有安装,可以通过pip进行安装:
pip install scikit-learn
然后,在代码中导入所需模块:
from sklearn.tree import DecisionTreeClassifier
- 准备数据
接下来,准备训练数据。数据通常以特征矩阵和目标向量的形式提供。
# 示例数据
X = [[0, 0], [1, 1]]
y = [0, 1]
- 初始化和训练模型
初始化决策树分类器,并使用训练数据来训练模型:
clf = DecisionTreeClassifier()
clf.fit(X, y)
- 预测
使用训练好的模型进行预测:
print(clf.predict([[2., 2.]]))
二、手动实现算法
手动实现决策树节点构建涉及更多的细节和算法知识。以下是关键步骤:
- 定义节点类
首先,定义一个节点类来表示树的每个节点:
class Node:
def __init__(self, gini, num_samples, num_samples_per_class, predicted_class):
self.gini = gini
self.num_samples = num_samples
self.num_samples_per_class = num_samples_per_class
self.predicted_class = predicted_class
self.feature_index = 0
self.threshold = 0
self.left = None
self.right = None
- 计算基尼不纯度
基尼不纯度是衡量数据集不纯度的一种方法。以下是计算基尼不纯度的函数:
def gini_impurity(y):
m = len(y)
return 1.0 - sum((np.sum(y == c) / m) 2 for c in np.unique(y))
- 寻找最佳分裂点
为了找到最佳分裂点,计算每个特征的基尼不纯度,并选择能够最大程度降低不纯度的分裂点:
def best_split(X, y):
m, n = X.shape
if m <= 1:
return None, None
num_parent = [np.sum(y == c) for c in np.unique(y)]
best_gini = 1.0 - sum((num / m) 2 for num in num_parent)
best_idx, best_thr = None, None
for idx in range(n):
thresholds, classes = zip(*sorted(zip(X[:, idx], y)))
num_left = [0] * len(np.unique(y))
num_right = num_parent.copy()
for i in range(1, m):
c = classes[i - 1]
num_left[c] += 1
num_right[c] -= 1
gini_left = 1.0 - sum((num_left[x] / i) 2 for x in np.unique(y))
gini_right = 1.0 - sum((num_right[x] / (m - i)) 2 for x in np.unique(y))
gini = (i * gini_left + (m - i) * gini_right) / m
if thresholds[i] == thresholds[i - 1]:
continue
if gini < best_gini:
best_gini = gini
best_idx = idx
best_thr = (thresholds[i] + thresholds[i - 1]) / 2
return best_idx, best_thr
- 构建决策树
递归地构建决策树,直到满足停止条件:
def build_tree(X, y, depth=0):
num_samples_per_class = [np.sum(y == i) for i in np.unique(y)]
predicted_class = np.argmax(num_samples_per_class)
node = Node(
gini=gini_impurity(y),
num_samples=len(y),
num_samples_per_class=num_samples_per_class,
predicted_class=predicted_class,
)
if depth < max_depth:
idx, thr = best_split(X, y)
if idx is not None:
indices_left = X[:, idx] < thr
X_left, y_left = X[indices_left], y[indices_left]
X_right, y_right = X[~indices_left], y[~indices_left]
node.feature_index = idx
node.threshold = thr
node.left = build_tree(X_left, y_left, depth + 1)
node.right = build_tree(X_right, y_right, depth + 1)
return node
三、选择分裂点
选择分裂点是构建决策树的关键步骤。一个好的分裂点可以最大程度地减少数据集的不纯度。可以使用基尼不纯度、信息增益等度量方法来选择最佳分裂点。
-
基尼不纯度
基尼不纯度是决策树算法中常用的一种度量方法。它通过衡量数据集中样本的不纯度来选择最佳分裂点。基尼不纯度越低,数据集越纯。
-
信息增益
信息增益是另一种常用的方法,通过计算分裂前后的信息熵来选择最佳分裂点。信息增益越高,说明分裂前后的信息熵差异越大,即分裂效果越好。
四、递归地构建树结构
在选择好分裂点后,通过递归的方式构建树结构。递归地处理每个子集,直到满足停止条件(如达到最大深度或节点样本数小于某个阈值)。
-
停止条件
为了避免过拟合,通常会设置一些停止条件,如达到最大树深度、节点样本数小于某个阈值、基尼不纯度低于某个阈值等。
-
递归处理
通过递归的方式处理每个子集,不断选择最佳分裂点,构建子节点,直到满足停止条件。这样可以逐步构建出完整的决策树结构。
五、实例代码
以下是一个完整的实例代码,展示了如何在Python中手动构建决策树的节点:
import numpy as np
class Node:
def __init__(self, gini, num_samples, num_samples_per_class, predicted_class):
self.gini = gini
self.num_samples = num_samples
self.num_samples_per_class = num_samples_per_class
self.predicted_class = predicted_class
self.feature_index = 0
self.threshold = 0
self.left = None
self.right = None
def gini_impurity(y):
m = len(y)
return 1.0 - sum((np.sum(y == c) / m) 2 for c in np.unique(y))
def best_split(X, y):
m, n = X.shape
if m <= 1:
return None, None
num_parent = [np.sum(y == c) for c in np.unique(y)]
best_gini = 1.0 - sum((num / m) 2 for num in num_parent)
best_idx, best_thr = None, None
for idx in range(n):
thresholds, classes = zip(*sorted(zip(X[:, idx], y)))
num_left = [0] * len(np.unique(y))
num_right = num_parent.copy()
for i in range(1, m):
c = classes[i - 1]
num_left[c] += 1
num_right[c] -= 1
gini_left = 1.0 - sum((num_left[x] / i) 2 for x in np.unique(y))
gini_right = 1.0 - sum((num_right[x] / (m - i)) 2 for x in np.unique(y))
gini = (i * gini_left + (m - i) * gini_right) / m
if thresholds[i] == thresholds[i - 1]:
continue
if gini < best_gini:
best_gini = gini
best_idx = idx
best_thr = (thresholds[i] + thresholds[i - 1]) / 2
return best_idx, best_thr
def build_tree(X, y, depth=0, max_depth=3):
num_samples_per_class = [np.sum(y == i) for i in np.unique(y)]
predicted_class = np.argmax(num_samples_per_class)
node = Node(
gini=gini_impurity(y),
num_samples=len(y),
num_samples_per_class=num_samples_per_class,
predicted_class=predicted_class,
)
if depth < max_depth:
idx, thr = best_split(X, y)
if idx is not None:
indices_left = X[:, idx] < thr
X_left, y_left = X[indices_left], y[indices_left]
X_right, y_right = X[~indices_left], y[~indices_left]
node.feature_index = idx
node.threshold = thr
node.left = build_tree(X_left, y_left, depth + 1, max_depth)
node.right = build_tree(X_right, y_right, depth + 1, max_depth)
return node
示例数据
X = np.array([[2, 3], [1, 1], [4, 5], [3, 2]])
y = np.array([0, 0, 1, 1])
构建决策树
tree = build_tree(X, y)
打印树的根节点
print("根节点基尼不纯度:", tree.gini)
print("样本数:", tree.num_samples)
print("每类样本数:", tree.num_samples_per_class)
print("预测类别:", tree.predicted_class)
通过上述步骤,可以在Python中手动实现决策树的节点构建,并了解其内部工作原理。使用现有库如Scikit-learn可以大大简化这一过程,并提高代码的可读性和效率。无论选择哪种方式,理解决策树的工作原理和构建过程对于掌握机器学习算法至关重要。
相关问答FAQs:
什么是决策树节点,如何在Python中定义它们?
决策树节点是决策树结构中的基本组成部分,每个节点代表一个特征的判断或决策。可以通过使用类来定义节点,在类中包含特征、阈值以及左右子节点的引用。使用numpy和pandas等库可以更容易地处理数据并构建决策树。
在Python中,如何选择决策树节点的最佳特征?
选择最佳特征通常使用信息增益、增益率或基尼指数等标准。可以利用sklearn库中的DecisionTreeClassifier
或DecisionTreeRegressor
函数,它们会自动选择最佳特征并建立决策树。通过传入数据集,这些函数将计算各特征的信息增益,并选择最优特征作为节点。
如何处理决策树中的过拟合问题?
过拟合是决策树常见的问题,可以通过设置树的最大深度、最小样本分裂数、最小样本叶子节点数等参数来进行控制。sklearn中的决策树模型提供了多种参数选项,合理调整这些参数可以提高模型的泛化能力,避免在训练数据上过拟合。
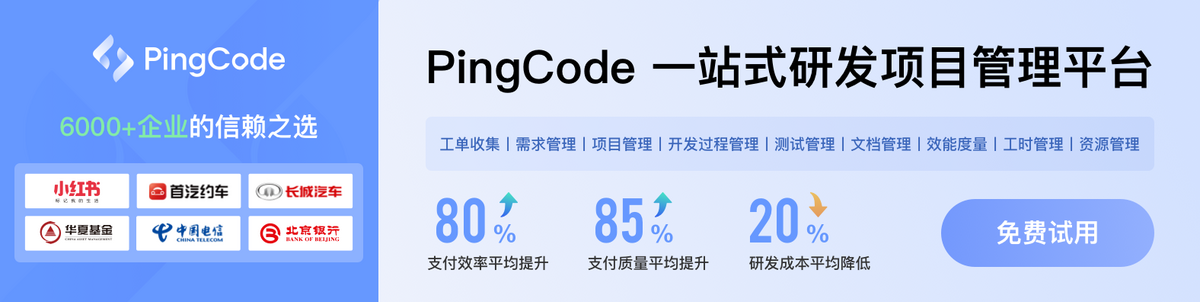