在Python中统计程序运行时间的方法有多种,主要包括使用time模块、datetime模块、timeit模块、cProfile模块等。每种方法都有其独特的优势和适用场景。本文将详细介绍这些方法,并提供具体的示例代码。
一、使用time模块
time模块是Python中最简单的方法之一,用于统计程序运行时间。主要通过time.time()
函数来记录程序开始和结束的时间,然后计算时间差。
使用步骤:
- 导入time模块。
- 在程序开始时记录开始时间。
- 在程序结束时记录结束时间。
- 计算并输出时间差。
import time
记录开始时间
start_time = time.time()
模拟程序运行(例如,休眠2秒)
time.sleep(2)
记录结束时间
end_time = time.time()
计算运行时间
elapsed_time = end_time - start_time
print(f"程序运行时间: {elapsed_time} 秒")
二、使用datetime模块
datetime模块也可以用于记录程序的开始和结束时间,并计算时间差。相比time模块,datetime模块提供了更多的时间和日期操作功能。
使用步骤:
- 导入datetime模块。
- 在程序开始时记录开始时间。
- 在程序结束时记录结束时间。
- 计算并输出时间差。
from datetime import datetime
记录开始时间
start_time = datetime.now()
模拟程序运行(例如,休眠2秒)
time.sleep(2)
记录结束时间
end_time = datetime.now()
计算运行时间
elapsed_time = end_time - start_time
print(f"程序运行时间: {elapsed_time}")
三、使用timeit模块
timeit模块专门用于计时小段代码的执行时间,尤其适合用于性能测试。它的精度更高,适合用于需要精确计时的场景。
使用步骤:
- 导入timeit模块。
- 定义要计时的代码段。
- 使用timeit模块的
timeit()
函数来计时。
import timeit
定义要计时的代码段
code_to_test = """
import time
time.sleep(2)
"""
计时
elapsed_time = timeit.timeit(code_to_test, number=1)
print(f"程序运行时间: {elapsed_time} 秒")
四、使用cProfile模块
cProfile模块是Python内置的性能分析器,用于分析程序的性能瓶颈。它不仅可以统计程序运行时间,还可以统计函数的调用次数和每次调用的时间,非常适合用于复杂程序的性能分析。
使用步骤:
- 导入cProfile模块。
- 创建性能分析对象。
- 使用性能分析对象的
run()
方法运行程序。 - 输出分析结果。
import cProfile
def test_program():
# 模拟程序运行(例如,休眠2秒)
time.sleep(2)
创建性能分析对象
profiler = cProfile.Profile()
运行程序并进行性能分析
profiler.enable()
test_program()
profiler.disable()
输出分析结果
profiler.print_stats()
五、使用装饰器
装饰器是一种灵活的方式,可以在不改变原函数的情况下,添加统计运行时间的功能。适用于需要多次统计不同函数运行时间的场景。
使用步骤:
- 定义一个装饰器函数。
- 在装饰器函数中统计开始和结束时间。
- 使用装饰器修饰需要计时的函数。
import time
def timeit_decorator(func):
def wrapper(*args, kwargs):
start_time = time.time()
result = func(*args, kwargs)
end_time = time.time()
elapsed_time = end_time - start_time
print(f"{func.__name__} 运行时间: {elapsed_time} 秒")
return result
return wrapper
@timeit_decorator
def test_program():
# 模拟程序运行(例如,休眠2秒)
time.sleep(2)
test_program()
六、使用contextlib上下文管理器
contextlib模块提供了一种优雅的方法来统计程序运行时间,可以通过上下文管理器的方式实现。这种方法适合用于需要在特定代码块前后执行一些操作的场景。
使用步骤:
- 导入contextlib模块。
- 定义一个上下文管理器。
- 在上下文管理器中统计开始和结束时间。
- 使用上下文管理器包裹需要计时的代码块。
import time
from contextlib import contextmanager
@contextmanager
def timeit_context():
start_time = time.time()
yield
end_time = time.time()
elapsed_time = end_time - start_time
print(f"代码块运行时间: {elapsed_time} 秒")
with timeit_context():
# 模拟程序运行(例如,休眠2秒)
time.sleep(2)
七、使用第三方库
除了Python内置的模块外,还有一些第三方库可以用于统计程序运行时间,例如line_profiler
、memory_profiler
等。这些库提供了更丰富的功能,可以帮助开发者深入分析程序的性能。
使用line_profiler
line_profiler可以按行统计代码的运行时间,非常适合用于找出代码中的性能瓶颈。
- 安装line_profiler库。
- 使用
@profile
装饰器修饰需要分析的函数。 - 运行程序并查看分析结果。
pip install line_profiler
# example.py
import time
@profile
def test_program():
# 模拟程序运行(例如,休眠2秒)
time.sleep(2)
if __name__ == "__main__":
test_program()
kernprof -l -v example.py
八、使用日志记录
在某些情况下,可能需要将程序运行时间记录到日志文件中,以便后续分析。这种方法适用于需要长期监控程序性能的场景。
使用步骤:
- 导入logging模块。
- 配置日志记录器。
- 在程序开始和结束时记录时间。
- 计算并记录运行时间。
import time
import logging
配置日志记录器
logging.basicConfig(filename='program.log', level=logging.INFO, format='%(asctime)s - %(message)s')
记录开始时间
start_time = time.time()
logging.info('程序开始运行')
模拟程序运行(例如,休眠2秒)
time.sleep(2)
记录结束时间
end_time = time.time()
logging.info('程序结束运行')
计算运行时间
elapsed_time = end_time - start_time
logging.info(f'程序运行时间: {elapsed_time} 秒')
总结
统计程序运行时间是性能优化和调试的基础工作。本文介绍了多种统计程序运行时间的方法,包括time模块、datetime模块、timeit模块、cProfile模块、装饰器、contextlib上下文管理器、第三方库和日志记录等。每种方法都有其独特的优势和适用场景,开发者可以根据具体需求选择合适的方法。
在实际应用中,可以结合多种方法进行综合分析,以便更准确地找到性能瓶颈并进行优化。希望本文能对您在统计程序运行时间和性能分析方面有所帮助。
相关问答FAQs:
如何在Python中测量代码块的执行时间?
在Python中,您可以使用time
模块中的time()
函数来测量代码块的执行时间。首先,在执行代码之前记录开始时间,然后在代码执行完成后记录结束时间。通过计算结束时间与开始时间的差值,即可获得执行时间。示例如下:
import time
start_time = time.time()
# 运行您的代码
end_time = time.time()
execution_time = end_time - start_time
print(f"执行时间为: {execution_time}秒")
使用哪个模块可以更方便地统计运行时间?timeit
模块是专为性能测试而设计的,它可以帮助您更方便地测量小段代码的执行时间。使用timeit
的好处在于,它会自动多次执行代码以获得更准确的统计结果。示例如下:
import timeit
execution_time = timeit.timeit('your_code_here', number=1000) # 代码执行1000次
print(f"平均执行时间为: {execution_time / 1000}秒")
是否有可视化工具可以帮助分析代码执行时间?
确实有一些可视化工具可以帮助您分析代码的执行时间和性能。比如,line_profiler
和memory_profiler
可以提供逐行代码的时间和内存使用情况。这些工具可以与Jupyter Notebook结合使用,便于对代码性能进行深入分析。安装这些工具后,可以使用装饰器来标记需要分析的函数。
通过这些方法,您可以有效地统计Python程序的运行时间,并根据需要优化代码性能。
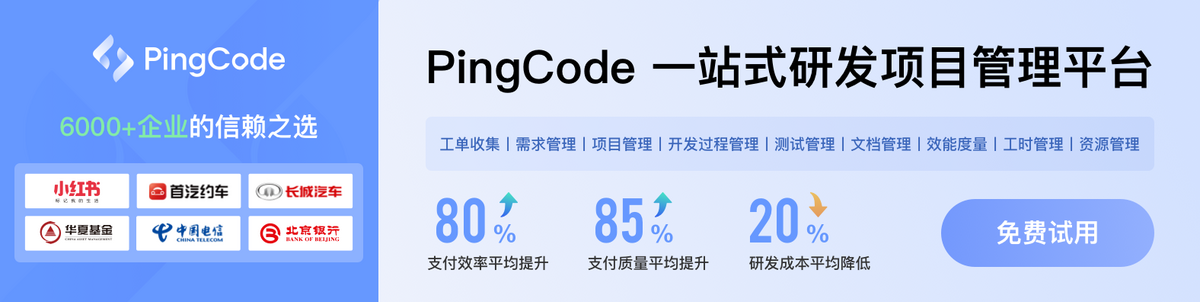