Python可以通过以下几种方式识别照片里的正方形:使用OpenCV库进行图像处理、利用轮廓检测、应用霍夫变换、通过形状匹配。 其中,使用OpenCV库进行图像处理和轮廓检测是最常见的方法。下面将详细描述如何使用OpenCV库和轮廓检测来识别正方形。
一、安装并导入必要的库
在开始编码之前,我们需要安装并导入必要的库。OpenCV是一个开源的计算机视觉库,提供了许多强大的工具和算法来处理图像和视频。我们还需要NumPy库来处理数组操作。
pip install opencv-python-headless numpy
import cv2
import numpy as np
二、读取和预处理图像
首先,我们需要读取图像并进行一些预处理步骤,如灰度转换和边缘检测。灰度转换可以减少计算量,而边缘检测可以帮助我们识别图像中的轮廓。
# 读取图像
image = cv2.imread('image.jpg')
转换为灰度图像
gray = cv2.cvtColor(image, cv2.COLOR_BGR2GRAY)
应用高斯模糊
blurred = cv2.GaussianBlur(gray, (5, 5), 0)
使用Canny边缘检测
edges = cv2.Canny(blurred, 50, 150)
三、寻找轮廓
使用边缘检测结果,我们可以在图像中寻找轮廓。OpenCV的findContours
函数可以帮助我们实现这一点。
# 寻找轮廓
contours, _ = cv2.findContours(edges, cv2.RETR_TREE, cv2.CHAIN_APPROX_SIMPLE)
四、过滤并识别正方形
在找到的轮廓中,我们需要过滤出正方形。可以通过轮廓的近似多边形来判断其形状。如果一个多边形有四个顶点且所有内角接近90度,那么我们可以认为它是一个正方形。
for contour in contours:
# 近似多边形
epsilon = 0.02 * cv2.arcLength(contour, True)
approx = cv2.approxPolyDP(contour, epsilon, True)
# 判断是否为正方形
if len(approx) == 4:
# 计算每个顶点之间的距离
sides = []
for i in range(4):
pt1 = approx[i][0]
pt2 = approx[(i + 1) % 4][0]
sides.append(np.linalg.norm(pt1 - pt2))
# 判断所有边是否相等(允许一定误差)
if max(sides) - min(sides) < 10:
# 绘制正方形轮廓
cv2.drawContours(image, [approx], -1, (0, 255, 0), 2)
显示结果图像
cv2.imshow('Squares', image)
cv2.waitKey(0)
cv2.destroyAllWindows()
五、优化和扩展
我们可以进一步优化和扩展这个基础代码,以适应不同的需求和应用场景。
1、调整边缘检测参数
根据图像的特点,可以调整Canny边缘检测的参数,以更准确地检测到正方形的边缘。
edges = cv2.Canny(blurred, lower_threshold, upper_threshold)
2、滤波和形态学操作
在边缘检测之前,可以应用更多的滤波和形态学操作,如膨胀和腐蚀,以减少噪声和孤立点。
kernel = np.ones((5, 5), np.uint8)
edges = cv2.dilate(edges, kernel, iterations=1)
edges = cv2.erode(edges, kernel, iterations=1)
3、适应不同的正方形大小
可以根据应用场景调整近似多边形的参数,适应不同大小的正方形。
epsilon = 0.01 * cv2.arcLength(contour, True)
4、处理不同的图像类型
可以扩展代码,使其适用于不同类型的图像,如彩色图像、二值图像等。
# 处理彩色图像
image = cv2.imread('color_image.jpg')
hsv = cv2.cvtColor(image, cv2.COLOR_BGR2HSV)
mask = cv2.inRange(hsv, lower_hsv, upper_hsv)
六、实际应用
识别正方形的技术可以应用在许多实际场景中,如:
1、二维码识别
二维码通常包含多个正方形图案,可以通过识别这些正方形来定位和解码二维码。
# 示例代码
import cv2
import numpy as np
读取图像
image = cv2.imread('qrcode.jpg')
gray = cv2.cvtColor(image, cv2.COLOR_BGR2GRAY)
blurred = cv2.GaussianBlur(gray, (5, 5), 0)
edges = cv2.Canny(blurred, 50, 150)
contours, _ = cv2.findContours(edges, cv2.RETR_TREE, cv2.CHAIN_APPROX_SIMPLE)
for contour in contours:
epsilon = 0.02 * cv2.arcLength(contour, True)
approx = cv2.approxPolyDP(contour, epsilon, True)
if len(approx) == 4:
sides = []
for i in range(4):
pt1 = approx[i][0]
pt2 = approx[(i + 1) % 4][0]
sides.append(np.linalg.norm(pt1 - pt2))
if max(sides) - min(sides) < 10:
cv2.drawContours(image, [approx], -1, (0, 255, 0), 2)
cv2.imshow('QR Code', image)
cv2.waitKey(0)
cv2.destroyAllWindows()
2、图像识别与分类
在图像识别与分类任务中,可以通过识别正方形来辅助特征提取和目标检测。
# 示例代码
import cv2
import numpy as np
读取图像
image = cv2.imread('shapes.jpg')
gray = cv2.cvtColor(image, cv2.COLOR_BGR2GRAY)
blurred = cv2.GaussianBlur(gray, (5, 5), 0)
edges = cv2.Canny(blurred, 50, 150)
contours, _ = cv2.findContours(edges, cv2.RETR_TREE, cv2.CHAIN_APPROX_SIMPLE)
for contour in contours:
epsilon = 0.02 * cv2.arcLength(contour, True)
approx = cv2.approxPolyDP(contour, epsilon, True)
if len(approx) == 4:
sides = []
for i in range(4):
pt1 = approx[i][0]
pt2 = approx[(i + 1) % 4][0]
sides.append(np.linalg.norm(pt1 - pt2))
if max(sides) - min(sides) < 10:
cv2.drawContours(image, [approx], -1, (0, 255, 0), 2)
cv2.imshow('Shapes', image)
cv2.waitKey(0)
cv2.destroyAllWindows()
3、工业检测
在工业检测中,可以通过识别正方形来检测产品的形状和尺寸,确保产品符合规格。
# 示例代码
import cv2
import numpy as np
读取图像
image = cv2.imread('products.jpg')
gray = cv2.cvtColor(image, cv2.COLOR_BGR2GRAY)
blurred = cv2.GaussianBlur(gray, (5, 5), 0)
edges = cv2.Canny(blurred, 50, 150)
contours, _ = cv2.findContours(edges, cv2.RETR_TREE, cv2.CHAIN_APPROX_SIMPLE)
for contour in contours:
epsilon = 0.02 * cv2.arcLength(contour, True)
approx = cv2.approxPolyDP(contour, epsilon, True)
if len(approx) == 4:
sides = []
for i in range(4):
pt1 = approx[i][0]
pt2 = approx[(i + 1) % 4][0]
sides.append(np.linalg.norm(pt1 - pt2))
if max(sides) - min(sides) < 10:
cv2.drawContours(image, [approx], -1, (0, 255, 0), 2)
cv2.imshow('Products', image)
cv2.waitKey(0)
cv2.destroyAllWindows()
七、总结
识别照片中的正方形是一个常见的图像处理任务,可以通过使用OpenCV库和轮廓检测技术来实现。本文详细介绍了如何读取和预处理图像、寻找轮廓、过滤并识别正方形,以及优化和扩展代码以适应不同的应用场景。通过调整参数和应用更多的图像处理技术,可以提高识别的准确性和鲁棒性。识别正方形的技术在二维码识别、图像识别与分类、工业检测等领域具有广泛的应用前景。
相关问答FAQs:
如何使用Python识别照片中的正方形?
在Python中,可以使用OpenCV库来识别照片中的正方形。首先,需要将图像转换为灰度图像,然后应用边缘检测算法(如Canny边缘检测)。接着,使用轮廓检测来找到图像中的形状,最后通过判断轮廓的边缘数量和角度来识别正方形。
识别正方形的Python代码示例是什么?
以下是一个基本示例代码,通过OpenCV库识别图像中的正方形:
import cv2
import numpy as np
# 读取图像
image = cv2.imread('image.jpg')
gray = cv2.cvtColor(image, cv2.COLOR_BGR2GRAY)
edges = cv2.Canny(gray, 50, 150)
# 查找轮廓
contours, _ = cv2.findContours(edges, cv2.RETR_TREE, cv2.CHAIN_APPROX_SIMPLE)
for contour in contours:
approx = cv2.approxPolyDP(contour, 0.02 * cv2.arcLength(contour, True), True)
if len(approx) == 4: # 正方形有四个边
cv2.drawContours(image, [approx], 0, (0, 255, 0), 5)
cv2.imshow('Squares', image)
cv2.waitKey(0)
cv2.destroyAllWindows()
这段代码会在识别出的正方形上绘制绿色轮廓。
除了正方形,我还可以使用Python识别哪些形状?
使用OpenCV,除了正方形,还可以识别其他多边形,如三角形、矩形、圆形等。只需根据边缘的数量和角度进行相应的判断。例如,三角形有三个边,矩形有四个边但不需要是正方形。通过调整条件,用户可以识别多种不同的几何形状。
在图像中识别正方形时,如何提高准确性?
提高识别准确性的一个有效方法是对图像进行预处理,例如调整对比度和亮度,使用高斯模糊来减少噪声。此外,选择合适的边缘检测参数和轮廓逼近精度也能显著提高检测的准确性。最后,确保图像质量良好,避免过于模糊或失真的图像,这样会更容易识别正方形。
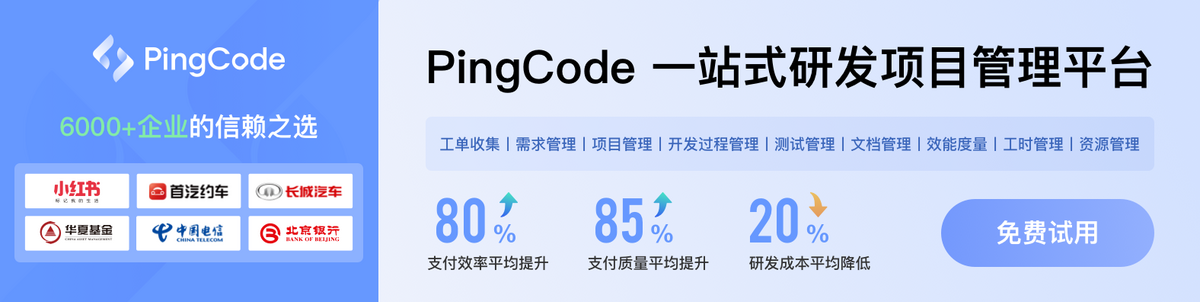