如何用Python制作关键词词频列表
使用Python制作关键词词频列表的方法包括:读取文本数据、对文本进行预处理、分词、计算词频、生成词频列表。 其中,对文本进行预处理是关键的一步,它包括去除标点符号、转换为小写等操作,这样可以确保词频统计的准确性。接下来,我们将详细介绍如何实现这些步骤。
一、读取文本数据
要制作关键词词频列表,首先需要读取文本数据。Python提供了多种读取文本数据的方法,最常用的包括直接读取本地文件、从网络上获取文本数据等。
1. 从本地文件读取文本数据
def read_text_file(file_path):
with open(file_path, 'r', encoding='utf-8') as file:
text = file.read()
return text
file_path = 'path/to/your/file.txt'
text_data = read_text_file(file_path)
2. 从网络上获取文本数据
import requests
def fetch_text_from_url(url):
response = requests.get(url)
response.raise_for_status() # Ensure we notice bad responses
return response.text
url = 'https://example.com/some-text-file.txt'
text_data = fetch_text_from_url(url)
二、对文本进行预处理
预处理是非常重要的一步,主要包括去除标点符号、转换为小写、去除停用词等。这些操作可以确保词频统计的准确性。
1. 去除标点符号和特殊字符
import re
def remove_punctuation(text):
return re.sub(r'[^\w\s]', '', text)
text_data = remove_punctuation(text_data)
2. 转换为小写
def convert_to_lowercase(text):
return text.lower()
text_data = convert_to_lowercase(text_data)
3. 去除停用词
停用词是指在文本处理中常常被忽略的词,如"的"、"是"、"在"等。可以使用NLTK库中的停用词列表来去除这些词。
import nltk
from nltk.corpus import stopwords
nltk.download('stopwords')
stop_words = set(stopwords.words('english'))
def remove_stopwords(text):
words = text.split()
filtered_words = [word for word in words if word not in stop_words]
return ' '.join(filtered_words)
text_data = remove_stopwords(text_data)
三、分词
分词是将文本数据分解为独立的单词或词组。对于英文文本,可以直接使用Python的split()
方法进行分词;而对于中文文本,可以使用结巴分词库。
1. 英文文本分词
def tokenize(text):
return text.split()
tokens = tokenize(text_data)
2. 中文文本分词
import jieba
def tokenize_chinese(text):
return list(jieba.cut(text))
tokens = tokenize_chinese(text_data)
四、计算词频
计算词频是制作关键词词频列表的核心步骤。可以使用Python的collections.Counter
类来实现这一功能。
from collections import Counter
def compute_word_frequencies(tokens):
return Counter(tokens)
word_frequencies = compute_word_frequencies(tokens)
五、生成词频列表
最后一步是生成词频列表,可以将词频信息输出为表格或文本文件。
1. 输出为文本文件
def write_frequencies_to_file(word_frequencies, output_file):
with open(output_file, 'w', encoding='utf-8') as file:
for word, freq in word_frequencies.items():
file.write(f'{word}: {freq}\n')
output_file = 'path/to/your/output.txt'
write_frequencies_to_file(word_frequencies, output_file)
2. 输出为CSV文件
import csv
def write_frequencies_to_csv(word_frequencies, output_file):
with open(output_file, 'w', newline='', encoding='utf-8') as csvfile:
writer = csv.writer(csvfile)
writer.writerow(['Word', 'Frequency'])
for word, freq in word_frequencies.items():
writer.writerow([word, freq])
output_file = 'path/to/your/output.csv'
write_frequencies_to_csv(word_frequencies, output_file)
总结
通过上述步骤,我们可以使用Python制作关键词词频列表。从读取文本数据、对文本进行预处理、分词、计算词频到生成词频列表,每一步都至关重要。对文本进行预处理是确保词频统计准确性的重要环节,包括去除标点符号、转换为小写、去除停用词等操作。此外,分词是将文本数据分解为独立的单词或词组的过程,可以使用Python的split()
方法或结巴分词库来实现。最后,通过计算词频和生成词频列表,我们可以得到文本中的关键词及其出现频率。希望通过本文的介绍,您能更好地理解如何使用Python制作关键词词频列表。
相关问答FAQs:
如何使用Python来分析文本中的关键词频率?
可以通过Python的文本处理库,如collections
和nltk
,来分析文本中的关键词频率。首先,读取文本数据,然后使用collections.Counter
来统计每个单词的出现次数。接着,可以利用nltk
进行分词和去除停用词,从而获得更准确的关键词频率。
在制作关键词词频列表时,如何处理停用词?
停用词是指在文本分析中通常会被忽略的常见词汇,如“的”、“是”、“在”等。在Python中,可以使用nltk.corpus.stopwords
来获取这些停用词列表。在分析关键词频率时,建议过滤掉这些停用词,以便更清晰地反映出核心关键词的频率。
如何将关键词词频列表可视化?
为了更好地展示关键词的频率,可以使用Python中的matplotlib
或wordcloud
库来进行可视化。matplotlib
可以帮助你绘制条形图或饼图,而wordcloud
则可以生成词云图,直观地反映出关键词的相对频率。通过这些可视化手段,用户可以更轻松地理解文本中的关键词分布情况。
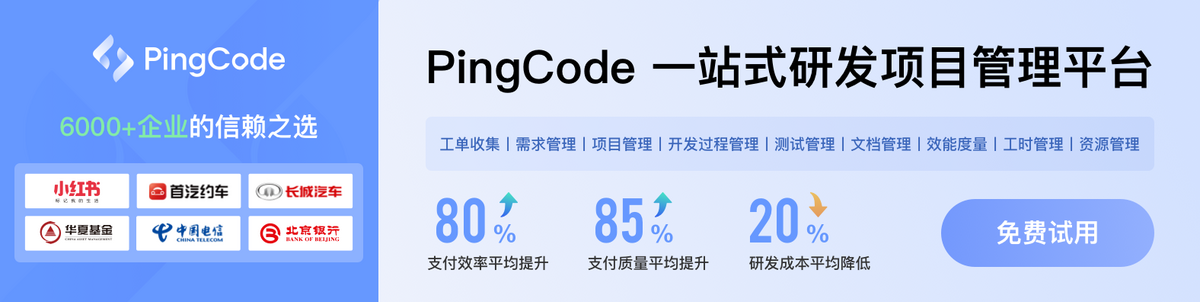