优化Python爬虫算法的关键在于提高爬虫的效率、稳定性和数据处理能力。具体可以从并发处理、请求优化、数据存储、异常处理等方面入手。本文将详细讨论以下几个方面:
一、提高并发处理能力
二、优化HTTP请求
三、数据存储与缓存
四、异常处理与重试机制
五、数据解析与处理
六、爬虫反制策略
一、提高并发处理能力
在爬虫算法中,提高并发处理能力是优化的一个重要方面。并发处理可以通过多线程、多进程或异步编程来实现。
1、多线程与多进程
多线程和多进程是常见的并发处理方式。多线程适用于I/O密集型任务,而多进程适用于CPU密集型任务。
-
多线程:利用
threading
模块,可以在爬虫中同时进行多个网络请求,从而提高爬取速度。import threading
def crawl(url):
# 爬取逻辑
pass
urls = ['http://example.com'] * 10
threads = []
for url in urls:
thread = threading.Thread(target=crawl, args=(url,))
threads.append(thread)
thread.start()
for thread in threads:
thread.join()
-
多进程:利用
multiprocessing
模块,可以在不同的进程中执行爬取任务,适用于CPU密集型任务。from multiprocessing import Pool
def crawl(url):
# 爬取逻辑
pass
urls = ['http://example.com'] * 10
pool = Pool(processes=4)
pool.map(crawl, urls)
pool.close()
pool.join()
2、异步编程
异步编程是一种高效的并发处理方式,尤其适用于I/O密集型任务。利用asyncio
和aiohttp
库,可以实现高效的异步爬虫。
import asyncio
import aiohttp
async def fetch(session, url):
async with session.get(url) as response:
return await response.text()
async def main(urls):
async with aiohttp.ClientSession() as session:
tasks = [fetch(session, url) for url in urls]
return await asyncio.gather(*tasks)
urls = ['http://example.com'] * 10
results = asyncio.run(main(urls))
二、优化HTTP请求
HTTP请求的优化直接关系到爬虫的效率和稳定性。通过合理设置请求头、代理池、重试机制等,可以显著提高爬虫的性能。
1、请求头设置
通过设置请求头,可以伪装成浏览器访问,提高请求成功率。
import requests
headers = {
'User-Agent': 'Mozilla/5.0 (Windows NT 10.0; Win64; x64) AppleWebKit/537.36 (KHTML, like Gecko) Chrome/58.0.3029.110 Safari/537.3'
}
response = requests.get('http://example.com', headers=headers)
2、使用代理池
使用代理池可以有效避免IP被封禁,提高爬取成功率。
import random
proxies = [
'http://123.456.789.000:8080',
'http://234.567.890.123:8080'
]
proxy = random.choice(proxies)
response = requests.get('http://example.com', proxies={'http': proxy, 'https': proxy})
3、设置超时和重试机制
设置请求超时和重试机制,可以提高爬虫的稳定性。
from requests.adapters import HTTPAdapter
from requests.packages.urllib3.util.retry import Retry
session = requests.Session()
retry = Retry(total=5, backoff_factor=1, status_forcelist=[500, 502, 503, 504])
adapter = HTTPAdapter(max_retries=retry)
session.mount('http://', adapter)
session.mount('https://', adapter)
response = session.get('http://example.com', timeout=5)
三、数据存储与缓存
数据存储和缓存是爬虫算法中非常重要的一环。选择合适的存储方式和缓存策略,可以大大提高数据处理的效率和可靠性。
1、选择合适的存储方式
根据数据量和使用场景,选择合适的存储方式。常见的存储方式有文件存储、数据库存储等。
-
文件存储:适用于小规模数据存储,可以使用CSV、JSON等格式。
import csv
data = [['name', 'age'], ['Alice', 30], ['Bob', 25]]
with open('data.csv', 'w', newline='') as file:
writer = csv.writer(file)
writer.writerows(data)
-
数据库存储:适用于大规模数据存储,可以使用MySQL、MongoDB等数据库。
import pymysql
connection = pymysql.connect(host='localhost', user='user', password='passwd', db='database')
cursor = connection.cursor()
cursor.execute("INSERT INTO table (name, age) VALUES ('Alice', 30)")
connection.commit()
connection.close()
2、缓存策略
使用缓存可以减少重复请求,提高爬虫效率。常见的缓存策略有内存缓存、文件缓存等。
-
内存缓存:可以使用
functools.lru_cache
装饰器实现简单的内存缓存。from functools import lru_cache
@lru_cache(maxsize=128)
def get_data(url):
response = requests.get(url)
return response.text
-
文件缓存:可以使用第三方库
requests-cache
实现文件缓存。import requests_cache
requests_cache.install_cache('cache', backend='sqlite', expire_after=3600)
response = requests.get('http://example.com')
四、异常处理与重试机制
在爬虫过程中,常常会遇到各种异常情况,如网络波动、服务器错误等。合理的异常处理与重试机制是保证爬虫稳定性的重要手段。
1、异常处理
通过捕获异常,可以避免程序因未处理的异常而中断。
try:
response = requests.get('http://example.com')
response.raise_for_status()
except requests.exceptions.RequestException as e:
print(f"Error: {e}")
2、重试机制
重试机制可以在请求失败时进行多次尝试,提高请求成功率。
import time
def fetch_with_retry(url, retries=3, backoff_factor=1):
for i in range(retries):
try:
response = requests.get(url, timeout=5)
response.raise_for_status()
return response.text
except requests.exceptions.RequestException:
if i < retries - 1:
time.sleep(backoff_factor * (2 i))
else:
raise
content = fetch_with_retry('http://example.com')
五、数据解析与处理
数据解析与处理是爬虫算法的重要组成部分。合理的数据解析与处理方法,可以提高数据的利用价值和处理效率。
1、HTML解析
常用的HTML解析库有BeautifulSoup
、lxml
等。
-
BeautifulSoup:一个简单易用的HTML解析库。
from bs4 import BeautifulSoup
html = '<html><body><h1>Hello, world!</h1></body></html>'
soup = BeautifulSoup(html, 'html.parser')
print(soup.h1.text)
-
lxml:一个高性能的XML和HTML解析库。
from lxml import etree
html = '<html><body><h1>Hello, world!</h1></body></html>'
tree = etree.HTML(html)
print(tree.xpath('//h1/text()')[0])
2、JSON解析
Python内置的json
库可以方便地进行JSON数据的解析和处理。
import json
json_data = '{"name": "Alice", "age": 30}'
data = json.loads(json_data)
print(data['name'])
六、爬虫反制策略
爬虫在实际应用中经常会遇到反制措施,如IP封禁、验证码等。合理的反制策略,可以有效提高爬虫的成功率。
1、代理池与IP轮换
使用代理池和IP轮换,可以有效避免IP被封禁。
import random
proxies = [
'http://123.456.789.000:8080',
'http://234.567.890.123:8080'
]
def get_proxy():
return random.choice(proxies)
proxy = get_proxy()
response = requests.get('http://example.com', proxies={'http': proxy, 'https': proxy})
2、模拟用户行为
通过模拟用户行为,可以降低被检测到是爬虫的风险。例如,随机设置请求间隔时间、模拟鼠标点击等。
import time
import random
def random_sleep():
time.sleep(random.uniform(1, 3))
urls = ['http://example.com'] * 10
for url in urls:
response = requests.get(url)
random_sleep()
3、处理验证码
对于一些需要验证码的网站,可以使用第三方验证码识别服务,如打码平台,或者使用OCR技术进行识别。
from PIL import Image
import pytesseract
image = Image.open('captcha.png')
text = pytesseract.image_to_string(image)
print(text)
总结
优化Python爬虫算法是一个系统工程,需要从并发处理、请求优化、数据存储、异常处理、数据解析和反制策略等多个方面进行综合考虑。通过提高并发处理能力、优化HTTP请求、选择合适的数据存储方式、合理的异常处理与重试机制、有效的数据解析与处理方法,以及应对反制措施,可以显著提高爬虫的效率、稳定性和数据处理能力。希望本文的内容能够帮助您在实际应用中更好地优化Python爬虫算法。
相关问答FAQs:
如何提高Python爬虫的抓取速度?
要提高Python爬虫的抓取速度,可以通过多线程或异步IO来实现并发请求,从而加快数据抓取的效率。此外,使用高效的库,如aiohttp
和requests-futures
,能够帮助实现更快的请求。同时,合理设置请求间隔,避免过于频繁地请求同一网站,以防被封禁。
在优化爬虫算法时,有哪些常见的反爬虫措施需要注意?
许多网站会使用反爬虫技术来保护其数据,这包括IP限制、请求频率限制、用户代理检测等。为了应对这些措施,可以采用动态IP代理、设置随机的用户代理,以及实现请求的随机延迟等策略,从而减少被检测到的风险。
如何提高爬取数据的准确性和完整性?
提高数据准确性和完整性可以从多个方面着手。首先,确保爬虫代码的健壮性,处理可能出现的异常情况。其次,采用数据去重策略,避免重复抓取相同的数据。最后,结合数据验证和清洗步骤,确保抓取到的数据是最新和最相关的,从而提升最终数据的质量。
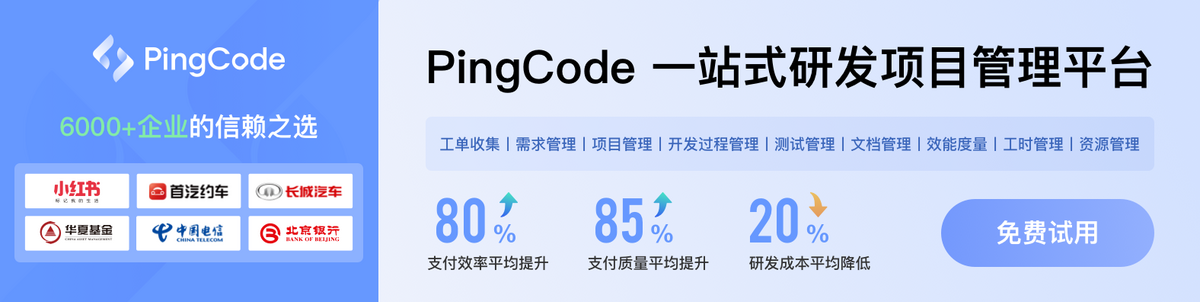