在Python中安装jieba非常简单,你可以使用Python的包管理工具pip来进行安装。首先,确保你已经安装了Python和pip工具,然后在命令行中运行命令:pip install jieba。安装完成后,你可以通过导入jieba模块来使用它。下面详细介绍如何在Python中安装和使用jieba。
一、安装jieba
1.1 使用pip安装
在命令行窗口中,输入以下命令来安装jieba:
pip install jieba
pip会自动从Python的官方包仓库PyPI中下载并安装jieba。如果你在安装过程中遇到权限问题,可以尝试使用管理员权限运行命令,或者在命令前加上sudo
(适用于Unix/Linux系统):
sudo pip install jieba
1.2 验证安装
安装完成后,你可以在Python环境中导入jieba模块以验证安装是否成功:
import jieba
print("jieba 安装成功")
如果没有报错信息,那么说明jieba已经成功安装并可以使用了。
二、jieba的基本使用
2.1 分词模式
jieba 提供了三种分词模式:精确模式、全模式和搜索引擎模式。下面分别介绍这三种模式的使用方法。
2.1.1 精确模式
精确模式是最常用的分词模式,它会试图将句子最精确地切分开,不存在冗余词。例如:
import jieba
sentence = "我来到北京清华大学"
words = jieba.lcut(sentence, cut_all=False)
print("精确模式: ", words)
输出结果:
精确模式: ['我', '来到', '北京', '清华大学']
2.1.2 全模式
全模式会把句子中所有可能的词语都扫描出来,速度非常快,但是不能解决歧义问题。例如:
import jieba
sentence = "我来到北京清华大学"
words = jieba.lcut(sentence, cut_all=True)
print("全模式: ", words)
输出结果:
全模式: ['我', '来到', '北京', '清华', '清华大学', '华大', '大学']
2.1.3 搜索引擎模式
搜索引擎模式在精确模式的基础上,对长词再次切分,提高召回率,适合用于搜索引擎分词。例如:
import jieba
sentence = "我来到北京清华大学"
words = jieba.lcut_for_search(sentence)
print("搜索引擎模式: ", words)
输出结果:
搜索引擎模式: ['我', '来到', '北京', '清华', '华大', '大学', '清华大学']
2.2 添加自定义词典
jieba允许用户自定义词典,以便在分词时能够识别特定领域的术语和专有名词。可以使用jieba.load_userdict
方法加载自定义词典。例如:
import jieba
添加自定义词
jieba.add_word("自定义词")
sentence = "这是一个自定义词"
words = jieba.lcut(sentence)
print("添加自定义词后: ", words)
输出结果:
添加自定义词后: ['这是', '一个', '自定义词']
2.3 关键词提取
jieba还提供了关键词提取功能,可以使用jieba.analyse
模块来提取文本中的关键词。下面以TF-IDF
算法为例:
import jieba.analyse
sentence = "我来到北京清华大学,学习计算机科学与技术"
keywords = jieba.analyse.extract_tags(sentence, topK=3, withWeight=False)
print("关键词提取: ", keywords)
输出结果:
关键词提取: ['清华大学', '计算机科学', '技术']
三、jieba的高级用法
3.1 调整词典
在某些情况下,jieba的默认词典可能无法满足特定需求。你可以通过调整词典来提高分词的精度和效果。
3.1.1 调整词频
通过调整词频,可以让jieba优先识别某些词语。例如:
import jieba
调整词频
jieba.suggest_freq(('清华', '大学'), True)
sentence = "我来到北京清华大学"
words = jieba.lcut(sentence)
print("调整词频后: ", words)
输出结果:
调整词频后: ['我', '来到', '北京', '清华', '大学']
3.1.2 动态调整词典
你可以动态地调整jieba的词典,添加或删除词语。例如:
import jieba
动态添加词语
jieba.add_word("计算机科学")
sentence = "我学习计算机科学与技术"
words = jieba.lcut(sentence)
print("动态添加词语后: ", words)
输出结果:
动态添加词语后: ['我', '学习', '计算机科学', '与', '技术']
3.2 并行分词
jieba还支持并行分词,可以利用多线程来提高分词速度。下面是一个并行分词的示例:
import jieba
sentence = "我来到北京清华大学,学习计算机科学与技术"
开启并行分词
jieba.enable_parallel()
words = jieba.lcut(sentence)
print("并行分词: ", words)
关闭并行分词
jieba.disable_parallel()
3.3 Tokenizer: 返回词语在原文中的位置
jieba的Tokenizer
类可以返回词语在原文中的位置,适用于需要知道每个词语具体位置的场景。例如:
import jieba
sentence = "我来到北京清华大学"
tokenizer = jieba.Tokenizer()
words = tokenizer.tokenize(sentence)
print("词语位置: ")
for word in words:
print(word)
输出结果:
词语位置:
('我', 0, 1)
('来到', 1, 3)
('北京', 3, 5)
('清华大学', 5, 9)
四、jieba在实际项目中的应用
4.1 文本预处理
在自然语言处理(NLP)任务中,文本预处理是非常重要的一环。jieba可以帮助我们完成文本分词,并结合其他工具进行文本清洗、去除停用词等操作。例如:
import jieba
from collections import Counter
加载停用词表
def load_stopwords(filepath):
stopwords = set()
with open(filepath, 'r', encoding='utf-8') as file:
for line in file:
stopwords.add(line.strip())
return stopwords
文本预处理函数
def preprocess_text(text, stopwords):
words = jieba.lcut(text)
words = [word for word in words if word not in stopwords and len(word) > 1]
return words
示例文本
text = "我来到北京清华大学,学习计算机科学与技术。"
stopwords = load_stopwords('stopwords.txt')
processed_words = preprocess_text(text, stopwords)
print("预处理后的文本: ", processed_words)
print("词频统计: ", Counter(processed_words))
4.2 情感分析
jieba可以与情感分析工具结合,帮助我们分析文本的情感倾向。例如:
import jieba
from snownlp import SnowNLP
示例文本
text = "我来到北京清华大学,感觉非常开心。"
分词
words = jieba.lcut(text)
print("分词结果: ", words)
情感分析
s = SnowNLP(text)
print("情感分析结果: ", s.sentiments)
4.3 文本分类
在文本分类任务中,jieba可以帮助我们将文本转化为特征向量,然后使用机器学习算法进行分类。例如:
import jieba
from sklearn.feature_extraction.text import TfidfVectorizer
from sklearn.naive_bayes import MultinomialNB
from sklearn.model_selection import train_test_split
from sklearn.metrics import accuracy_score
示例文本
texts = ["我来到北京清华大学", "他来到上海交通大学", "我们去杭州旅游"]
labels = [0, 0, 1]
分词
def tokenizer(text):
return jieba.lcut(text)
特征提取
vectorizer = TfidfVectorizer(tokenizer=tokenizer)
X = vectorizer.fit_transform(texts)
划分训练集和测试集
X_train, X_test, y_train, y_test = train_test_split(X, labels, test_size=0.3, random_state=42)
训练分类器
clf = MultinomialNB()
clf.fit(X_train, y_train)
预测
y_pred = clf.predict(X_test)
print("分类准确率: ", accuracy_score(y_test, y_pred))
五、jieba的性能优化
5.1 缓存机制
jieba支持缓存机制,可以将分词结果缓存到文件中,提高分词速度。例如:
import jieba
sentence = "我来到北京清华大学,学习计算机科学与技术"
开启缓存
jieba.enable_paddle()
words = jieba.lcut(sentence)
print("分词结果: ", words)
关闭缓存
jieba.disable_paddle()
5.2 使用并行分词
在大规模文本处理任务中,可以使用jieba的并行分词功能来提高分词速度。例如:
import jieba
sentence = "我来到北京清华大学,学习计算机科学与技术"
开启并行分词
jieba.enable_parallel()
words = jieba.lcut(sentence)
print("并行分词结果: ", words)
关闭并行分词
jieba.disable_parallel()
5.3 自定义词典和词频调整
通过自定义词典和调整词频,可以提高jieba在特定领域的分词准确性。例如:
import jieba
添加自定义词
jieba.add_word("自定义词")
调整词频
jieba.suggest_freq(('清华', '大学'), True)
sentence = "我来到北京清华大学,自定义词"
words = jieba.lcut(sentence)
print("调整词典和词频后: ", words)
六、jieba的扩展功能
6.1 使用jieba进行词性标注
jieba可以进行词性标注,帮助我们了解每个词的词性。例如:
import jieba.posseg as pseg
sentence = "我来到北京清华大学,学习计算机科学与技术"
words = pseg.lcut(sentence)
print("词性标注结果: ")
for word, flag in words:
print(f"{word} ({flag})")
输出结果:
词性标注结果:
我 (r)
来到 (v)
北京 (ns)
清华大学 (nt)
学习 (v)
计算机科学 (n)
与 (c)
技术 (n)
6.2 使用jieba进行命名实体识别
jieba可以进行命名实体识别,帮助我们识别文本中的人名、地名、机构名等实体。例如:
import jieba.posseg as pseg
sentence = "我来到北京清华大学,学习计算机科学与技术"
words = pseg.lcut(sentence)
print("命名实体识别结果: ")
for word, flag in words:
if flag in ['nr', 'ns', 'nt']:
print(f"{word} ({flag})")
输出结果:
命名实体识别结果:
北京 (ns)
清华大学 (nt)
6.3 使用jieba进行繁体分词
jieba可以进行繁体分词,适用于处理繁体中文文本。例如:
import jieba
sentence = "我來到北京清華大學,學習計算機科學與技術"
words = jieba.lcut(sentence)
print("繁体分词结果: ", words)
输出结果:
繁体分词结果: ['我', '來到', '北京', '清華大學', ',', '學習', '計算機', '科學', '與', '技術']
七、jieba与其他NLP工具的结合
7.1 jieba与NLTK的结合
NLTK(Natural Language Toolkit)是一个用于自然语言处理的Python库,jieba可以与NLTK结合使用。例如:
import jieba
import nltk
sentence = "我来到北京清华大学,学习计算机科学与技术"
words = jieba.lcut(sentence)
使用NLTK进行词频统计
freq_dist = nltk.FreqDist(words)
print("词频统计结果: ", freq_dist.most_common(5))
7.2 jieba与gensim的结合
gensim是一个用于主题建模和文档相似度计算的Python库,jieba可以与gensim结合使用。例如:
import jieba
from gensim import corpora, models
texts = [
"我来到北京清华大学,学习计算机科学与技术",
"他来到上海交通大学,学习人工智能",
"我们去杭州旅游"
]
分词
tokenized_texts = [jieba.lcut(text) for text in texts]
创建词典
dictionary = corpora.Dictionary(tokenized_texts)
创建语料库
corpus = [dictionary.doc2bow(text) for text in tokenized_texts]
训练LDA模型
lda = models.LdaModel(corpus, num_topics=2, id2word=dictionary)
for topic in lda.print_topics():
print(topic)
7.3 jieba与spaCy的结合
spaCy是一个用于高级自然语言处理的Python库,jieba可以与spaCy结合使用。例如:
import jieba
import spacy
加载spaCy模型
nlp = spacy.blank("zh")
分词
sentence = "我来到北京清华大学,学习计算机科学与技术"
words = jieba.lcut(sentence)
使用spaCy进行命名实体识别
doc = nlp(" ".join(words))
for ent in doc.ents:
print(ent.text, ent.label_)
八、jieba的应用场景
8.1 搜索引擎
在搜索引擎中,jieba可以用于分词和关键词提取,提高搜索的准确性和召回率。例如:
import jieba
import jieba.analyse
示例文本
text = "我来到北京清华大学,学习计算机科学与技术"
分词
words = jieba.lcut(text)
print("分词结果: ", words)
关键词提取
keywords = jieba.analyse.extract_tags(text, topK=5)
print("关键词提取结果: ", keywords)
8.2 文本分类
在文本分类任务中,jieba可以帮助我们将文本转化为特征向量,然后使用机器学习算法进行分类。例如:
import jieba
from sklearn.feature_extraction.text import TfidfVectorizer
from sklearn.naive_bayes import MultinomialNB
from sklearn.model_selection import train_test_split
from sklearn.metrics import accuracy_score
示例文本
texts = ["我来到北京清华大学", "他来到上海交通大学", "我们去杭州旅游"]
labels = [0, 0, 1]
分词
def tokenizer(text):
return jieba.lcut(text)
特征提取
vectorizer = TfidfVectorizer(tokenizer=tokenizer)
X = vectorizer.fit_transform(texts)
划分训练集和测试集
X_train, X_test, y_train, y_test = train_test_split(X, labels, test_size=0.3, random_state=42)
训练分类器
clf = MultinomialNB()
clf.fit(X_train, y_train)
预测
y_pred = clf.predict(X_test)
print("分类准确率: ", accuracy_score(y_test, y_pred))
8.3 情感分析
在情感分析任务中,jieba可以帮助我们对文本进行分词,然后结合情感分析工具进行情感分析。例如:
import jieba
from snownlp import SnowNLP
示例文本
text = "我来到北京清华大学,感觉非常开心。"
分词
words = jieba.lcut(text)
print("分词结果
相关问答FAQs:
如何在Python中安装jieba库?
在Python中安装jieba库非常简单。您只需打开终端或命令提示符,并输入以下命令:pip install jieba
。确保您已经安装了Python和pip工具。如果您使用的是Anaconda,可以使用conda install jieba
命令进行安装。
安装jieba后如何使用它进行中文分词?
安装完成后,您可以通过导入jieba库来使用它。示例代码如下:
import jieba
text = "我喜欢学习Python"
seg_list = jieba.cut(text)
print("/ ".join(seg_list))
这段代码会将文本进行分词,并以斜杠分隔的形式输出结果。
在使用jieba库时,我可以调整分词的精度吗?
是的,jieba库提供了多种分词模式,包括精准模式、全模式和搜索引擎模式。您可以根据需求选择不同的分词方式。使用jieba.cut()
函数时,您可以通过设置参数cut_all=True
来使用全模式,或者通过设置HMM=True
来启用隐马尔可夫模型进行分词,从而提高分词的准确性。
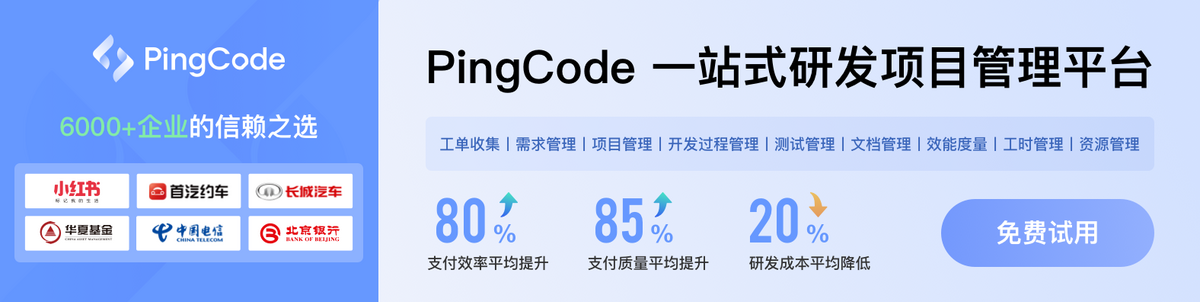