提高Python爬虫效率的方法包括:并发爬取、使用异步IO、减少请求次数、缓存策略、优化网络请求、使用高效的数据解析库、分布式爬虫、压缩传输数据。其中,并发爬取是一种有效且常用的方法,能够显著提升爬虫的效率。
并发爬取是指通过多线程或多进程的方式,让爬虫同时进行多个请求,而不是一个请求一个请求地顺序执行。这可以大大减少爬取的总时间,因为爬虫可以在等待一个请求响应的同时,发送其他请求。Python中的threading
和multiprocessing
库可以用来实现并发爬取。此外,第三方库如concurrent.futures
和aiohttp
提供了更加方便的异步并发爬取方式。
一、并发爬取
- 多线程爬取
多线程爬取是通过创建多个线程来同时进行多个请求,减少爬取的时间。Python的threading
库非常适合这种任务。
import threading
import requests
def fetch_url(url):
response = requests.get(url)
print(f"Fetched {url} with status code {response.status_code}")
urls = ["http://example.com" for _ in range(10)]
threads = []
for url in urls:
thread = threading.Thread(target=fetch_url, args=(url,))
threads.append(thread)
thread.start()
for thread in threads:
thread.join()
这种方法简单有效,但需要注意线程安全和全局解释器锁(GIL)对多线程的限制。
- 多进程爬取
多进程爬取通过创建多个进程来并发执行任务,可以绕过GIL的限制。Python的multiprocessing
库提供了这种功能。
import multiprocessing
import requests
def fetch_url(url):
response = requests.get(url)
print(f"Fetched {url} with status code {response.status_code}")
if __name__ == "__main__":
urls = ["http://example.com" for _ in range(10)]
processes = []
for url in urls:
process = multiprocessing.Process(target=fetch_url, args=(url,))
processes.append(process)
process.start()
for process in processes:
process.join()
多进程爬取更适合CPU密集型任务,但会占用更多的系统资源。
- 异步IO爬取
异步IO爬取通过asyncio
和aiohttp
库实现,可以在一个线程内并发执行多个网络请求,适用于I/O密集型任务。
import asyncio
import aiohttp
async def fetch_url(session, url):
async with session.get(url) as response:
print(f"Fetched {url} with status code {response.status}")
async def main():
async with aiohttp.ClientSession() as session:
tasks = [fetch_url(session, "http://example.com") for _ in range(10)]
await asyncio.gather(*tasks)
asyncio.run(main())
异步IO爬取的优势在于高效利用I/O等待时间,减少总的爬取时间。
二、使用异步IO
异步IO是一种在单线程中实现并发的方法,特别适合网络I/O密集型任务。Python的asyncio
库和aiohttp
库提供了强大的异步IO功能。
- asyncio库
asyncio
库是Python标准库的一部分,它提供了编写异步代码的支持。通过使用async
和await
关键字,可以定义和执行异步函数。
import asyncio
async def hello_world():
await asyncio.sleep(1)
print("Hello, World!")
asyncio.run(hello_world())
- aiohttp库
aiohttp
是一个基于asyncio
的异步HTTP客户端和服务器库。它非常适合用来编写高效的爬虫。
import asyncio
import aiohttp
async def fetch_url(session, url):
async with session.get(url) as response:
print(f"Fetched {url} with status code {response.status}")
async def main():
async with aiohttp.ClientSession() as session:
tasks = [fetch_url(session, "http://example.com") for _ in range(10)]
await asyncio.gather(*tasks)
asyncio.run(main())
通过异步IO的方式,可以在等待一个请求的响应时,去处理其他请求,从而提高爬虫的效率。
三、减少请求次数
减少请求次数是一种简单但有效的优化方法,主要通过以下几种方式实现:
- 合并请求
将多个请求合并为一个请求,可以减少网络开销。例如,如果一个页面包含多个资源,可以通过一个请求获取所有资源。
- 避免重复请求
在爬取过程中,避免重复请求相同的URL。可以使用集合(set)来存储已经请求过的URL。
visited_urls = set()
def fetch_url(url):
if url not in visited_urls:
response = requests.get(url)
visited_urls.add(url)
print(f"Fetched {url} with status code {response.status_code}")
else:
print(f"Skipped {url}, already visited")
- 使用HEAD请求
在需要判断资源是否更新时,可以使用HEAD请求而不是GET请求。HEAD请求只获取响应头部,不会下载响应体,从而减少数据传输量。
response = requests.head("http://example.com")
if response.status_code == 200:
print("Resource is available")
四、缓存策略
缓存策略可以有效减少重复请求,提高爬虫的效率。常见的缓存策略包括:
- 本地缓存
将已经请求过的数据缓存到本地文件或数据库中,在再次请求时直接读取缓存数据。
import os
def fetch_url(url):
cache_file = f"cache/{url.replace('/', '_')}.html"
if os.path.exists(cache_file):
with open(cache_file, 'r') as file:
content = file.read()
print(f"Loaded {url} from cache")
else:
response = requests.get(url)
with open(cache_file, 'w') as file:
file.write(response.text)
print(f"Fetched {url} with status code {response.status_code}")
- 使用缓存库
使用现成的缓存库如requests-cache
,可以轻松实现请求缓存。
import requests
import requests_cache
requests_cache.install_cache('cache')
response = requests.get("http://example.com")
print(response.from_cache)
五、优化网络请求
优化网络请求可以减少请求时间,提高爬虫的效率。常见的优化方法包括:
- 使用连接池
连接池可以重用TCP连接,减少建立连接的开销。requests
库支持连接池。
import requests
from requests.adapters import HTTPAdapter
from requests.packages.urllib3.util.retry import Retry
session = requests.Session()
retry = Retry(connect=3, backoff_factor=0.5)
adapter = HTTPAdapter(max_retries=retry)
session.mount('http://', adapter)
session.mount('https://', adapter)
response = session.get("http://example.com")
print(response.status_code)
- 启用HTTP/2
HTTP/2支持多路复用,可以在一个TCP连接上同时发送多个请求。httpx
库支持HTTP/2。
import httpx
client = httpx.Client(http2=True)
response = client.get("http://example.com")
print(response.status_code)
- 压缩传输数据
启用数据压缩可以减少数据传输量。requests
库和aiohttp
库都支持数据压缩。
import requests
response = requests.get("http://example.com", headers={"Accept-Encoding": "gzip"})
print(response.headers.get("Content-Encoding"))
import aiohttp
import asyncio
async def fetch_url(session, url):
async with session.get(url, headers={"Accept-Encoding": "gzip"}) as response:
print(response.headers.get("Content-Encoding"))
async def main():
async with aiohttp.ClientSession() as session:
await fetch_url(session, "http://example.com")
asyncio.run(main())
六、使用高效的数据解析库
选择高效的数据解析库可以减少数据处理时间,提高爬虫的效率。常见的数据解析库包括:
- lxml
lxml
是一个高效的XML和HTML解析库,支持XPath查询。
from lxml import html
tree = html.fromstring(response.content)
title = tree.xpath('//title/text()')
print(title)
- BeautifulSoup
BeautifulSoup
是一个简单易用的HTML解析库,支持多种解析器。
from bs4 import BeautifulSoup
soup = BeautifulSoup(response.content, 'html.parser')
title = soup.title.string
print(title)
- pyquery
pyquery
是一个类似jQuery的Python库,支持CSS选择器。
from pyquery import PyQuery as pq
doc = pq(response.content)
title = doc('title').text()
print(title)
七、分布式爬虫
分布式爬虫通过将爬取任务分配到多个节点上,可以大大提高爬虫的效率和吞吐量。常见的分布式爬虫框架包括:
- Scrapy
Scrapy是一个强大的爬虫框架,支持分布式爬取。可以通过Scrapy-Redis扩展实现分布式爬取。
# settings.py
SCHEDULER = "scrapy_redis.scheduler.Scheduler"
DUPEFILTER_CLASS = "scrapy_redis.dupefilter.RFPDupeFilter"
REDIS_URL = 'redis://localhost:6379'
spider.py
import scrapy
from scrapy_redis.spiders import RedisSpider
class MySpider(RedisSpider):
name = 'myspider'
redis_key = 'myspider:start_urls'
def parse(self, response):
# your parsing logic here
pass
- PySpider
PySpider是一个支持分布式爬取的爬虫框架,具有强大的WebUI和任务调度功能。
from pyspider.libs.base_handler import BaseHandler
class Handler(BaseHandler):
def on_start(self):
self.crawl('http://example.com', callback=self.index_page)
def index_page(self, response):
for each in response.doc('a[href^="http"]').items():
self.crawl(each.attr.href, callback=self.detail_page)
def detail_page(self, response):
return {
"url": response.url,
"title": response.doc('title').text(),
}
八、压缩传输数据
压缩传输数据可以减少数据传输量,提高爬虫的效率。常见的压缩方式包括:
- 启用Gzip压缩
HTTP协议支持Gzip压缩,可以通过设置请求头来启用Gzip压缩。
import requests
response = requests.get("http://example.com", headers={"Accept-Encoding": "gzip"})
print(response.headers.get("Content-Encoding"))
- 启用Brotli压缩
Brotli是另一种高效的压缩算法,支持的服务器和客户端越来越多。
import requests
response = requests.get("http://example.com", headers={"Accept-Encoding": "br"})
print(response.headers.get("Content-Encoding"))
九、总结
提高Python爬虫效率的方法有很多,包括并发爬取、使用异步IO、减少请求次数、缓存策略、优化网络请求、使用高效的数据解析库、分布式爬虫和压缩传输数据。通过合理选择和组合这些方法,可以显著提升爬虫的效率和性能。
- 并发爬取:通过多线程、多进程或异步IO实现并发爬取,可以显著减少总的爬取时间。
- 使用异步IO:异步IO适用于I/O密集型任务,可以在一个线程内并发执行多个网络请求。
- 减少请求次数:通过合并请求、避免重复请求和使用HEAD请求,可以减少网络开销。
- 缓存策略:通过本地缓存和使用缓存库,可以减少重复请求,提高爬虫效率。
- 优化网络请求:通过使用连接池、启用HTTP/2和压缩传输数据,可以减少请求时间和数据传输量。
- 使用高效的数据解析库:选择高效的数据解析库可以减少数据处理时间。
- 分布式爬虫:通过将爬取任务分配到多个节点上,可以大大提高爬虫的效率和吞吐量。
- 压缩传输数据:通过启用Gzip和Brotli压缩,可以减少数据传输量。
通过以上方法,可以显著提高Python爬虫的效率,满足大规模数据爬取的需求。在实际应用中,可以根据具体情况选择适合的方法,并进行合理组合和优化。
相关问答FAQs:
如何选择合适的库来提高Python爬虫的效率?
在Python中,有许多库可以帮助提高爬虫的效率,例如Scrapy、Requests和BeautifulSoup。Scrapy是一个功能强大的框架,能够处理复杂的爬虫任务,并提供异步处理功能,显著提高爬虫速度。Requests库适合于简单的HTTP请求,而BeautifulSoup则用于解析HTML。选择合适的库可以根据项目的需求和复杂程度来决定,确保在处理数据时更加高效。
使用代理和分布式爬虫能否提高爬虫效率?
是的,使用代理服务器和分布式爬虫能够显著提高爬虫的效率。代理服务器可以帮助绕过IP限制和防爬虫机制,使得爬虫可以更频繁地访问目标网站。同时,分布式爬虫通过将任务分配给多个爬虫实例,可以实现并行处理,进一步加快数据抓取的速度。这种方法对于大规模数据抓取尤其有效。
如何优化爬虫的请求速度和数据处理速度?
优化请求速度可以通过设置合适的请求间隔、使用异步请求和减少不必要的请求来实现。例如,使用aiohttp
库进行异步请求,可以在等待响应时继续执行其他操作,从而提高整体效率。在数据处理方面,可以使用多线程或多进程来加速数据解析和存储,同时将数据存储方式优化为批量插入,以减少数据库操作的时间开销。
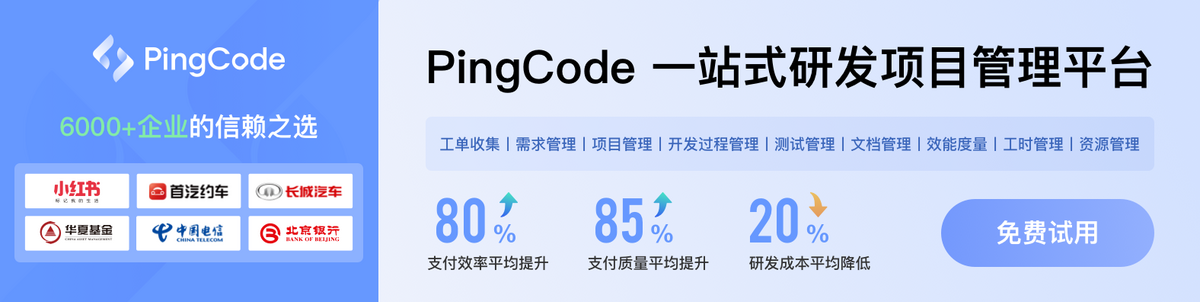