在Python中设置最佳值(也称为超参数优化)是一个极其重要的任务,因为不同的值会对模型的性能产生显著影响。使用网格搜索、随机搜索、贝叶斯优化、交叉验证、使用学习曲线是设置最佳值的一些有效方法。其中,网格搜索是一个常用的技术,它通过穷举搜索来寻找最佳组合。网格搜索(Grid Search)是一种系统地遍历多个参数组合的技术,能够保证找到最优解。它通过为每个参数指定一组可能的值,然后通过交叉验证评估每个组合来选择最佳参数。
一、网格搜索(Grid Search)
网格搜索是一种简单且常用的超参数优化方法。它通过系统地遍历指定的参数值组合来找到最优参数。
1、原理
网格搜索的基本原理是将每个超参数的可能取值组成一个网格,然后对每个组合进行交叉验证,最终选择表现最好的参数组合。这个方法虽然简单,但由于需要遍历所有可能的组合,计算量较大,适用于参数空间较小的情况。
2、实现步骤
(1)定义参数网格:为每个超参数指定一组可能的取值。
from sklearn.model_selection import GridSearchCV
from sklearn.ensemble import RandomForestClassifier
param_grid = {
'n_estimators': [100, 200, 300],
'max_depth': [None, 10, 20, 30],
'min_samples_split': [2, 5, 10]
}
(2)初始化模型:选择要优化的模型。
model = RandomForestClassifier()
(3)执行网格搜索:使用网格搜索来寻找最佳参数组合。
grid_search = GridSearchCV(estimator=model, param_grid=param_grid, cv=5)
grid_search.fit(X_train, y_train)
(4)查看最佳参数:通过best_params_
属性获取最优参数组合。
best_params = grid_search.best_params_
print("Best parameters found: ", best_params)
二、随机搜索(Random Search)
随机搜索是一种改进的超参数优化方法,它通过随机采样参数空间来寻找最佳参数。
1、原理
与网格搜索不同,随机搜索不会遍历所有可能的组合,而是在参数空间中随机选择一定数量的组合进行评估。这样可以在大幅降低计算量的同时,仍然有较高的概率找到接近最优的参数组合。
2、实现步骤
(1)定义参数分布:为每个超参数指定一个概率分布或范围。
from sklearn.model_selection import RandomizedSearchCV
from scipy.stats import randint
param_dist = {
'n_estimators': randint(100, 500),
'max_depth': [None, 10, 20, 30],
'min_samples_split': randint(2, 11)
}
(2)初始化模型:选择要优化的模型。
model = RandomForestClassifier()
(3)执行随机搜索:使用随机搜索来寻找最佳参数组合。
random_search = RandomizedSearchCV(estimator=model, param_distributions=param_dist, n_iter=50, cv=5)
random_search.fit(X_train, y_train)
(4)查看最佳参数:通过best_params_
属性获取最优参数组合。
best_params = random_search.best_params_
print("Best parameters found: ", best_params)
三、贝叶斯优化(Bayesian Optimization)
贝叶斯优化是一种更为智能的超参数优化方法,它通过构建代理模型来预测参数空间的表现,从而更高效地找到最优参数。
1、原理
贝叶斯优化利用代理模型(通常是高斯过程)来模拟目标函数的表现,并根据预测的结果选择下一个评估的参数组合。这个过程会不断迭代,逐步收敛到最优参数。
2、实现步骤
(1)安装必要的库:贝叶斯优化通常需要使用bayesian-optimization
库。
pip install bayesian-optimization
(2)定义目标函数:目标函数应该返回模型的评估指标(如准确率)。
from bayes_opt import BayesianOptimization
from sklearn.model_selection import cross_val_score
def rf_cv(n_estimators, max_depth, min_samples_split):
model = RandomForestClassifier(
n_estimators=int(n_estimators),
max_depth=int(max_depth),
min_samples_split=int(min_samples_split)
)
return cross_val_score(model, X_train, y_train, cv=5).mean()
(3)定义参数空间:为每个超参数指定一个范围。
param_bounds = {
'n_estimators': (100, 500),
'max_depth': (10, 30),
'min_samples_split': (2, 11)
}
(4)执行贝叶斯优化:使用贝叶斯优化来寻找最佳参数组合。
optimizer = BayesianOptimization(f=rf_cv, pbounds=param_bounds, random_state=42)
optimizer.maximize(init_points=10, n_iter=50)
(5)查看最佳参数:通过max
属性获取最优参数组合。
best_params = optimizer.max['params']
print("Best parameters found: ", best_params)
四、交叉验证(Cross-Validation)
交叉验证是一种评估模型性能的方法,通过多次分割数据集来获得更稳定的评估结果。
1、原理
交叉验证的基本原理是将数据集分为K个子集,然后进行K次训练和测试,每次使用一个子集作为测试集,其他子集作为训练集。最终的评估结果是K次测试结果的平均值。
2、实现步骤
(1)选择交叉验证方法:常见的交叉验证方法包括K折交叉验证、留一法交叉验证等。
from sklearn.model_selection import cross_val_score
from sklearn.ensemble import RandomForestClassifier
model = RandomForestClassifier()
scores = cross_val_score(model, X_train, y_train, cv=5)
(2)查看评估结果:通过mean
方法获取平均评估结果。
print("Cross-validation scores: ", scores)
print("Mean score: ", scores.mean())
五、学习曲线(Learning Curve)
学习曲线是一种评估模型性能随训练数据量变化的方法,可以帮助我们了解模型的泛化能力和数据需求。
1、原理
学习曲线通过在不同大小的训练集上训练模型,并评估其在训练集和验证集上的表现,从而反映出模型的学习过程和数据需求。通过观察学习曲线,我们可以判断模型是否存在过拟合或欠拟合问题。
2、实现步骤
(1)生成学习曲线:使用learning_curve
函数生成学习曲线数据。
from sklearn.model_selection import learning_curve
import numpy as np
train_sizes, train_scores, test_scores = learning_curve(
model, X_train, y_train, cv=5, n_jobs=-1, train_sizes=np.linspace(0.1, 1.0, 10)
)
(2)计算平均得分:计算训练集和验证集的平均得分。
train_scores_mean = np.mean(train_scores, axis=1)
test_scores_mean = np.mean(test_scores, axis=1)
(3)绘制学习曲线:使用matplotlib
绘制学习曲线。
import matplotlib.pyplot as plt
plt.figure()
plt.plot(train_sizes, train_scores_mean, 'o-', color="r", label="Training score")
plt.plot(train_sizes, test_scores_mean, 'o-', color="g", label="Cross-validation score")
plt.xlabel("Training examples")
plt.ylabel("Score")
plt.legend(loc="best")
plt.title("Learning Curve")
plt.show()
六、结合多种方法优化超参数
在实际应用中,常常需要结合多种方法来进行超参数优化。例如,可以先使用随机搜索来粗略搜索参数空间,然后再使用网格搜索对结果进行精细调整。
1、初步随机搜索
首先,使用随机搜索在较大的参数空间中进行初步搜索,找到表现较好的参数范围。
random_search = RandomizedSearchCV(estimator=model, param_distributions=param_dist, n_iter=50, cv=5)
random_search.fit(X_train, y_train)
best_params_random = random_search.best_params_
2、精细网格搜索
然后,根据随机搜索的结果,缩小参数范围,使用网格搜索进行精细调整。
param_grid_fine = {
'n_estimators': [best_params_random['n_estimators']-50, best_params_random['n_estimators'], best_params_random['n_estimators']+50],
'max_depth': [best_params_random['max_depth']-5, best_params_random['max_depth'], best_params_random['max_depth']+5],
'min_samples_split': [best_params_random['min_samples_split']-1, best_params_random['min_samples_split'], best_params_random['min_samples_split']+1]
}
grid_search_fine = GridSearchCV(estimator=model, param_grid=param_grid_fine, cv=5)
grid_search_fine.fit(X_train, y_train)
best_params_fine = grid_search_fine.best_params_
七、参数调优的实践建议
在进行参数调优时,有几个实践建议可以帮助提高效率和效果。
1、分步进行
参数调优最好分步进行,先对影响较大的参数进行调整,然后再调整次要参数。例如,对于随机森林,可以先调整树的数量和深度,然后再调整分裂标准和叶节点数量。
2、使用较小的数据集
在初步调整参数时,可以使用较小的数据集进行快速测试,找到较好的参数范围后,再使用全量数据进行精细调整。
3、关注模型的泛化能力
在调整参数时,不仅要关注模型在训练集上的表现,更要关注其在验证集上的表现,以避免过拟合和欠拟合问题。
4、结合业务需求
参数调优不仅仅是为了提高模型的准确率,还要结合业务需求,例如模型的计算效率、响应速度等。选择适当的参数平衡模型性能和实际应用需求。
八、案例分析
为了更好地理解超参数优化的方法,我们通过一个实际案例来演示整个过程。
1、问题描述
假设我们要使用随机森林模型对一个分类任务进行建模,目标是通过调整超参数提高模型的准确率。
2、数据准备
首先,我们准备好训练数据和测试数据。
from sklearn.datasets import load_iris
from sklearn.model_selection import train_test_split
data = load_iris()
X_train, X_test, y_train, y_test = train_test_split(data.data, data.target, test_size=0.2, random_state=42)
3、初步随机搜索
使用随机搜索在较大的参数空间中进行初步搜索。
param_dist = {
'n_estimators': randint(100, 500),
'max_depth': [None, 10, 20, 30],
'min_samples_split': randint(2, 11)
}
random_search = RandomizedSearchCV(estimator=RandomForestClassifier(), param_distributions=param_dist, n_iter=50, cv=5)
random_search.fit(X_train, y_train)
best_params_random = random_search.best_params_
print("Best parameters from random search: ", best_params_random)
4、精细网格搜索
根据随机搜索的结果,缩小参数范围,使用网格搜索进行精细调整。
param_grid_fine = {
'n_estimators': [best_params_random['n_estimators']-50, best_params_random['n_estimators'], best_params_random['n_estimators']+50],
'max_depth': [best_params_random['max_depth']-5, best_params_random['max_depth'], best_params_random['max_depth']+5],
'min_samples_split': [best_params_random['min_samples_split']-1, best_params_random['min_samples_split'], best_params_random['min_samples_split']+1]
}
grid_search_fine = GridSearchCV(estimator=RandomForestClassifier(), param_grid=param_grid_fine, cv=5)
grid_search_fine.fit(X_train, y_train)
best_params_fine = grid_search_fine.best_params_
print("Best parameters from grid search: ", best_params_fine)
5、模型评估
使用最佳参数训练模型,并在测试集上进行评估。
model_best = RandomForestClassifier(best_params_fine)
model_best.fit(X_train, y_train)
accuracy = model_best.score(X_test, y_test)
print("Model accuracy with best parameters: ", accuracy)
通过上述步骤,我们可以系统地进行超参数优化,找到最优参数组合,从而提升模型的性能。
九、总结
通过本文,我们详细介绍了Python中设置最佳值的方法,包括网格搜索、随机搜索、贝叶斯优化、交叉验证、学习曲线等。同时,我们通过实际案例演示了如何结合多种方法进行参数调优。希望通过这些方法和实践建议,能够帮助大家更高效地进行模型调优,提高模型的性能和泛化能力。
相关问答FAQs:
如何在Python中设置最佳值?
在Python中设置最佳值通常涉及到调整参数以优化模型或算法的性能。可以通过交叉验证、网格搜索或随机搜索等方法来找到最佳参数。使用像Scikit-learn这样的库,可以轻松实现这些技术,从而提高模型的准确性和效率。
在机器学习项目中,如何确定最佳值?
确定最佳值需要评估不同参数对模型性能的影响。可以通过绘制学习曲线、混淆矩阵和ROC曲线等可视化工具来观察模型的表现。同时,采用K折交叉验证可以有效避免过拟合,并帮助找到最优参数。
最佳值设置对程序性能有何影响?
设置最佳值能够显著提升程序的性能和准确性。在数据科学和机器学习中,合理的参数配置能够提高模型对新数据的泛化能力,减少误差,从而使得预测结果更加可靠。这对于实际应用中至关重要,尤其是在面临复杂数据集时。
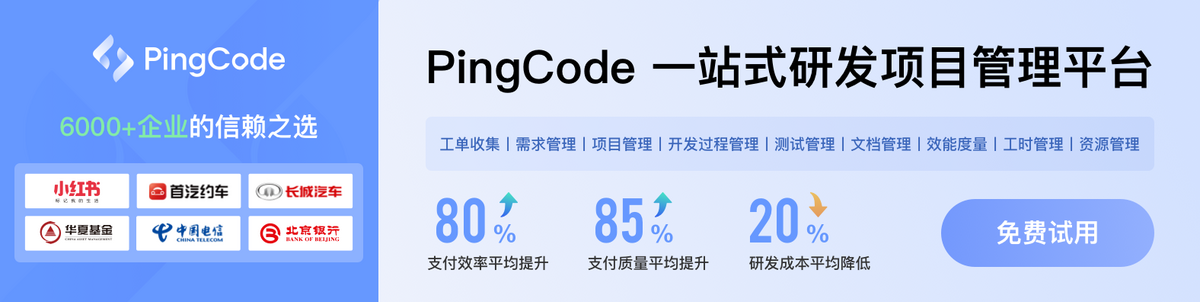