Python爬虫多线程可以通过使用threading
模块、concurrent.futures
模块、以及multiprocessing
模块来实现,以提高爬虫的效率和速度。多线程能够让爬虫在处理I/O密集型任务时更加高效,因为它能够在等待网络请求时执行其他任务。
多线程是指在一个进程中同时执行多个线程,每个线程共享相同的内存空间。对于爬虫来说,网络I/O通常是主要的瓶颈,多线程可以在等待网络请求响应的同时执行其他请求,从而提高效率。为了实现多线程爬虫,可以使用Python的threading
模块来创建和管理线程。
一、THREADING模块
threading
模块是Python标准库的一部分,它提供了一种简单的方法来实现多线程。对于爬虫来说,threading
模块可以用来同时处理多个URL请求。
1. 创建线程
在Python中,可以通过threading.Thread
类创建线程。以下是一个简单的例子,展示了如何使用threading
模块创建和启动多个线程:
import threading
import requests
def fetch_url(url):
response = requests.get(url)
print(f"Fetched {url} with status code {response.status_code}")
urls = ["http://example.com", "http://example.org", "http://example.net"]
threads = []
for url in urls:
thread = threading.Thread(target=fetch_url, args=(url,))
threads.append(thread)
thread.start()
for thread in threads:
thread.join()
2. 线程同步
在多线程编程中,线程同步是一个重要的问题。Python提供了多种同步机制,如锁(Lock)、条件变量(Condition)等。对于爬虫来说,通常需要在爬取数据时进行同步,以避免数据竞争和不一致。
import threading
lock = threading.Lock()
def fetch_url_with_lock(url):
global lock
response = requests.get(url)
lock.acquire()
try:
print(f"Fetched {url} with status code {response.status_code}")
finally:
lock.release()
二、CONCURRENT.FUTURES模块
concurrent.futures
模块是Python 3.2引入的一个高级并发模块,它提供了一个高级接口来管理线程和进程池。对于爬虫来说,concurrent.futures.ThreadPoolExecutor
是一个非常有用的工具。
1. 使用ThreadPoolExecutor
ThreadPoolExecutor
可以很方便地管理多个线程,并自动处理线程的创建和销毁。以下是一个使用ThreadPoolExecutor
的例子:
from concurrent.futures import ThreadPoolExecutor
def fetch_url(url):
response = requests.get(url)
return f"Fetched {url} with status code {response.status_code}"
urls = ["http://example.com", "http://example.org", "http://example.net"]
with ThreadPoolExecutor(max_workers=5) as executor:
results = executor.map(fetch_url, urls)
for result in results:
print(result)
2. 处理异常
在使用concurrent.futures
时,可以通过捕获Future
对象的异常来处理请求失败的情况:
def fetch_url_with_exception(url):
try:
response = requests.get(url)
return f"Fetched {url} with status code {response.status_code}"
except requests.RequestException as e:
return f"Error fetching {url}: {e}"
with ThreadPoolExecutor(max_workers=5) as executor:
futures = [executor.submit(fetch_url_with_exception, url) for url in urls]
for future in futures:
print(future.result())
三、MULTIPROCESSING模块
虽然threading
和concurrent.futures
可以有效地提高I/O密集型任务的效率,但在CPU密集型任务中,多线程的效果可能不如多进程。Python的multiprocessing
模块可以用来创建多个进程,以充分利用多核CPU的优势。
1. 使用ProcessPoolExecutor
ProcessPoolExecutor
是concurrent.futures
模块的一部分,它可以用来管理进程池。以下是一个使用ProcessPoolExecutor
的例子:
from concurrent.futures import ProcessPoolExecutor
def compute_intensive_task(data):
# 假设这是一个计算密集型任务
return sum(x * x for x in data)
data = [range(10000), range(10000), range(10000)]
with ProcessPoolExecutor(max_workers=3) as executor:
results = executor.map(compute_intensive_task, data)
for result in results:
print(result)
2. 对比多线程
在使用多进程时,每个进程都有自己的内存空间,因此不会出现线程间的数据竞争问题。然而,多进程的创建和销毁开销比多线程更大,因此在选择使用多线程还是多进程时,需要根据具体的任务性质来决定。
四、综合应用
在实际应用中,爬虫通常需要处理多个网站的数据,因此可以结合使用threading
和concurrent.futures
来提高效率。同时,还需要考虑请求的频率、反爬虫机制和数据存储等问题。
1. 请求频率控制
在多线程爬虫中,需要控制请求的频率以避免被目标网站封禁。可以使用time.sleep
函数来实现简单的频率控制,或者使用Queue
来管理请求任务。
import time
import queue
def fetch_with_delay(url, delay):
time.sleep(delay)
response = requests.get(url)
print(f"Fetched {url} with status code {response.status_code}")
2. 反爬虫机制应对
许多网站都有反爬虫机制,如检测请求频率、检查User-Agent、使用CAPTCHA等。可以通过模拟浏览器请求、使用代理和IP轮换等方式来规避这些机制。
headers = {'User-Agent': 'Mozilla/5.0'}
response = requests.get(url, headers=headers)
3. 数据存储
爬虫获取的数据需要进行存储和处理,可以使用数据库(如MySQL、MongoDB)或文件(如CSV、JSON)来保存数据。
import csv
def save_to_csv(data, filename):
with open(filename, 'w', newline='') as csvfile:
writer = csv.writer(csvfile)
writer.writerow(['URL', 'Status'])
for row in data:
writer.writerow(row)
五、总结
Python爬虫多线程可以通过使用threading
模块、concurrent.futures
模块和multiprocessing
模块来实现,具体选择取决于任务的性质。对于I/O密集型任务,多线程通常是更好的选择,而对于CPU密集型任务,多进程可能更为合适。在实际应用中,还需要结合请求频率控制、反爬虫机制应对和数据存储等策略,以实现高效、可靠的爬虫系统。无论选择哪种方法,确保代码的可维护性和高效性都是至关重要的。
相关问答FAQs:
如何在Python爬虫中实现多线程?
在Python爬虫中实现多线程可以通过使用threading
模块或concurrent.futures.ThreadPoolExecutor
来提高抓取效率。你可以创建多个线程,每个线程负责爬取不同的网页。需要注意的是,网络请求应当是线程安全的,同时要控制线程数量,避免对目标网站造成过大的压力。
多线程爬虫会遇到哪些常见问题?
多线程爬虫可能会面临许多挑战,例如线程安全、数据竞争和爬取效率的平衡。若多个线程同时访问共享数据,可能会导致数据不一致,需使用锁机制来保证数据的完整性。此外,过多的线程可能导致网络请求过于频繁,进而触发网站的反爬虫机制。
如何控制多线程爬虫的爬取速度?
控制多线程爬虫的爬取速度可以通过设置请求之间的延迟时间、限制线程数量和使用连接池来实现。适当的请求间隔可以避免对目标网站造成过大的压力,同时应遵循网站的robots.txt
规则,确保爬虫行为的合法性和道德性。通过这些方法,可以有效地管理爬虫的行为,避免被封禁。
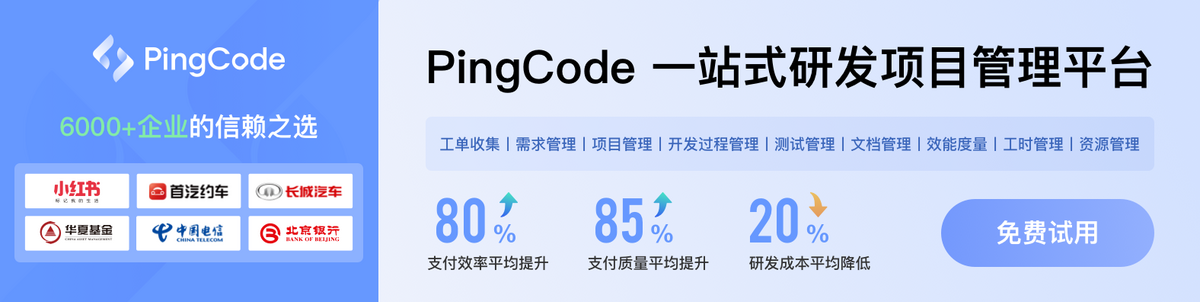