Python绘制二维密度图的步骤包括:导入必要的库、准备数据、使用Seaborn或Matplotlib绘制图形、调整图形样式和保存图形。下面我们将详细介绍这些步骤。
一、导入必要的库
在开始绘制二维密度图之前,首先需要导入一些Python库,例如:NumPy、Pandas、Matplotlib和Seaborn。这些库提供了数据处理和图形绘制的基本功能。
import numpy as np
import pandas as pd
import matplotlib.pyplot as plt
import seaborn as sns
二、准备数据
在绘制图形之前,必须准备好数据。数据可以是从文件中读取的,也可以是通过代码生成的。例如,我们可以使用NumPy生成一些随机数据。
# 生成随机数据
data = np.random.multivariate_normal([0, 0], [[1, 0.5], [0.5, 1]], size=1000)
df = pd.DataFrame(data, columns=['x', 'y'])
三、使用Seaborn绘制二维密度图
Seaborn是一个基于Matplotlib的高级图形库,专门用于绘制统计图形。使用Seaborn,可以非常方便地绘制二维密度图。
# 使用Seaborn绘制二维密度图
plt.figure(figsize=(8, 6))
sns.kdeplot(x=df['x'], y=df['y'], cmap="Blues", shade=True, bw_adjust=0.5)
plt.title('2D Density Plot using Seaborn')
plt.xlabel('X-axis')
plt.ylabel('Y-axis')
plt.show()
四、使用Matplotlib绘制二维密度图
虽然Seaborn更为方便,但有时我们可能需要使用Matplotlib进行更细致的控制。使用Matplotlib绘制二维密度图需要更多的步骤,但可以提供更高的灵活性。
# 使用Matplotlib绘制二维密度图
plt.figure(figsize=(8, 6))
计算二维直方图
hist, xedges, yedges = np.histogram2d(df['x'], df['y'], bins=30, density=True)
绘制密度图
xcenters = (xedges[:-1] + xedges[1:]) / 2
ycenters = (yedges[:-1] + yedges[1:]) / 2
plt.contourf(xcenters, ycenters, hist.T, cmap="Blues")
plt.title('2D Density Plot using Matplotlib')
plt.xlabel('X-axis')
plt.ylabel('Y-axis')
plt.colorbar(label='Density')
plt.show()
五、调整图形样式
无论使用Seaborn还是Matplotlib,都可以对图形进行进一步的调整,例如设置颜色、添加标题、调整坐标轴等等。
# 调整Seaborn绘制的图形样式
plt.figure(figsize=(8, 6))
sns.kdeplot(x=df['x'], y=df['y'], cmap="viridis", shade=True, bw_adjust=0.5)
plt.title('2D Density Plot with Adjusted Style')
plt.xlabel('X-axis')
plt.ylabel('Y-axis')
plt.grid(True)
plt.show()
# 调整Matplotlib绘制的图形样式
plt.figure(figsize=(8, 6))
计算二维直方图
hist, xedges, yedges = np.histogram2d(df['x'], df['y'], bins=30, density=True)
绘制密度图
xcenters = (xedges[:-1] + xedges[1:]) / 2
ycenters = (yedges[:-1] + yedges[1:]) / 2
plt.contourf(xcenters, ycenters, hist.T, cmap="inferno")
plt.title('2D Density Plot with Adjusted Style')
plt.xlabel('X-axis')
plt.ylabel('Y-axis')
plt.colorbar(label='Density')
plt.grid(True)
plt.show()
六、保存图形
绘制完成后,可以将图形保存为不同格式的文件,例如PNG、JPG或PDF。
# 保存Seaborn绘制的图形
plt.figure(figsize=(8, 6))
sns.kdeplot(x=df['x'], y=df['y'], cmap="Blues", shade=True, bw_adjust=0.5)
plt.title('2D Density Plot')
plt.xlabel('X-axis')
plt.ylabel('Y-axis')
plt.savefig('2d_density_plot_seaborn.png')
# 保存Matplotlib绘制的图形
plt.figure(figsize=(8, 6))
计算二维直方图
hist, xedges, yedges = np.histogram2d(df['x'], df['y'], bins=30, density=True)
绘制密度图
xcenters = (xedges[:-1] + xedges[1:]) / 2
ycenters = (yedges[:-1] + yedges[1:]) / 2
plt.contourf(xcenters, ycenters, hist.T, cmap="Blues")
plt.title('2D Density Plot')
plt.xlabel('X-axis')
plt.ylabel('Y-axis')
plt.colorbar(label='Density')
plt.savefig('2d_density_plot_matplotlib.png')
通过上述步骤,您可以使用Python绘制出专业且美观的二维密度图。这些图形不仅可以帮助您更好地理解数据,还可以用于科学研究、数据分析报告和展示等多个领域。
相关问答FAQs:
如何使用Python绘制二维密度图?
在Python中,绘制二维密度图最常用的库是Matplotlib和Seaborn。你可以使用Seaborn的kdeplot()
函数或Matplotlib的hist2d()
函数来实现。首先,确保你安装了这些库,可以通过命令pip install matplotlib seaborn
来完成。接下来,使用示例数据集,可以轻松绘制出二维密度图。
二维密度图与散点图有什么区别?
散点图主要用于展示数据点之间的关系,而二维密度图则显示数据点的分布密度。通过密度图,你可以更直观地看到数据集中点的聚集区域和空白区域,帮助你更好地理解数据的分布特性。
绘制二维密度图时有哪些常见参数可以调整?
在绘制二维密度图时,可以调整多个参数以优化图形的效果。例如,可以设置cmap
参数来更改色彩映射,使用levels
参数控制等高线的数量,以及通过bw_adjust
参数来调整核密度估计的带宽。这些参数的调整可以使得图形更具可读性和美观性。
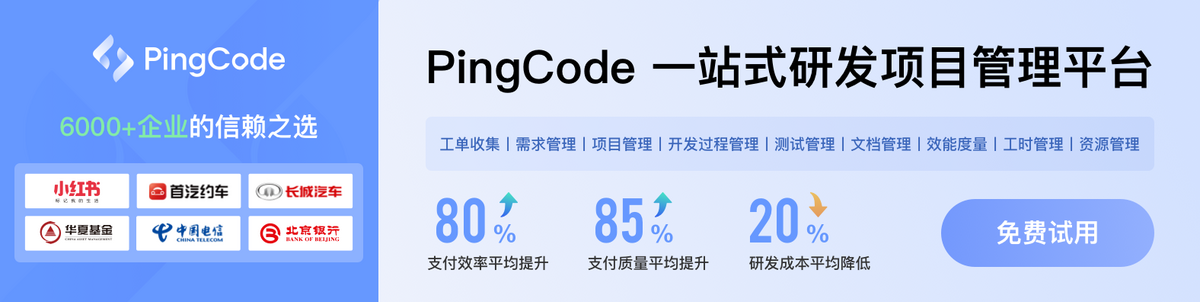